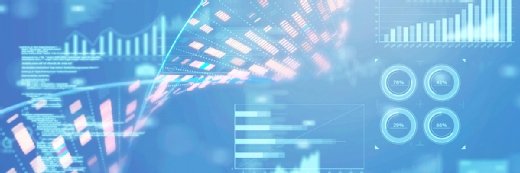
Yuichiro Chino/Moment via Getty
Bias a Chief Barrier to Artificial Intelligence in Healthcare
The potential for algorithms to perpetuate bias and exacerbate disparities is a critical hurdle for artificial intelligence in healthcare.
To ensure the safe and effective use of artificial intelligence in healthcare, researchers and developers will need to work to eliminate bias in these tools, according to a perspective paper by Stanford University researchers.
As artificial intelligence tools grow more prevalent in the medical field, leaders need to make sure these technologies benefit all populations and demographics.
“The white body and the male body have long been the norm in medicine guiding drug discovery, treatment and standards of care, so it’s important that we do not let AI devices fall into that historical pattern,” said Londa Schiebinger, the John L. Hinds Professor in the History of Science in the School of Humanities and Sciences at Stanford.
“We’re hoping to engage the AI biomedical community in preventing bias and creating equity in the initial design of research, rather than having to fix things after the fact.”
To develop equitable AI devices, adequate data collection is a key first step. The team noted that while public datasets often fail to represent minority populations, private datasets that may be more diverse are usually restricted to a single hospital or academic center.
Improving the reliability of AI systems will require leaders to fix this gap, and there are several existing efforts that aim to do so.
“The paucity of annotated photos of darker-skin individuals is a significant barrier for dermatology and telehealth algorithms. The Stanford Skin of Color Project is an ongoing crowd science effort to collect and curate the largest publicly available dataset of dermatologically relevant images from darker skin tones. This data can help to train and assess machine learning models,” researchers said.
“In genetics, there are similar efforts to prioritize the collection and analysis of non-European genetics data, which is necessary for genetic understandings of diseases such as polygenetic risk scores to benefit diverse populations. For example, the recent PAGE study demonstrates a robust framework to identify new genetic correlates of phenotypes using over 49,000 non-European individuals.”
In addition to collecting diverse data, researchers emphasized the importance of consistently evaluating AI tools, even after organizations have deployed them.
“Post deployment monitoring of AI algorithms is an emerging area of research. Researchers have developed statistical tests to detect if the data that an algorithm is applied to is substantially different from the data that the algorithm was trained on. These tests trigger real-time warnings that the deployed AI algorithm may have biases due to its training data. This can be a practical approach for monitoring,” the team wrote.
“We recommend that hospitals and regulators such as the FDA consider the monitoring framework and the AI algorithm as a holistic package to be evaluated and deployed together.”
In terms of longer-term solutions, the team cited the need for regulatory agencies to promote equity in AI use.
“The FDA has set out five criteria for excellence in its Digital Health Innovation Action Plan. The specific guidelines to certify medical computer-aided systems, however, do not mention sex, gender, or other axes of health disparities in data collection,” researchers stated.
“We recommend expanding the current FDA guidelines for software as a medical device (SaMD) to include a four-part pre- and post-market review of ML health tools: an analysis of health disparities in the clinical domain of interest; a review of training data for bias; transparency surrounding decisions made regarding model performance, especially in relation to health disparities; and post-market review of health equity outcomes.”
Universities could also support the development responsible AI by refining coursework and curricula.
“Ethics is most often taught in stand-alone modules (39 percent) and not linked to core curricula (28 percent–the other 33 percent combine both approaches),” the group said.
“The AI for biomedicine community can also benefit from curriculum that incorporates ethics into computational training by drawing from the relevant material in both CS and medical ethics.”
Teams should also be diverse to ensure algorithms don’t exacerbate disparities, the team stated.
“Additional problems may arise from the lack of gender and ethnic diversity in AI teams, a situation that can contribute to perpetuating unconscious biases in research design and outcomes. Teams should be diverse in terms of participants and also in terms of skill sets and methods,” researchers said.
“AI researchers themselves should understand the basics of sex, gender, diversity, and intersectional analysis as these relate to their technical work.”
The widespread use of AI in healthcare will depend on the ability for researchers and developers to eliminate potential bias.
“As with other biomedical technologies such as genome sequencing and editing, it is critical that innovation in biomedical AI is complemented by efforts to reduce human risk and to ensure that its benefits are broadly shared by diverse countries and populations,” the team concluded.
“Clearly, technology alone is not the fix; large social problems that undergird structural inequality need to be addressed. Nonetheless, researchers and educators can do their part to develop education and technologies that strive toward social justices.”