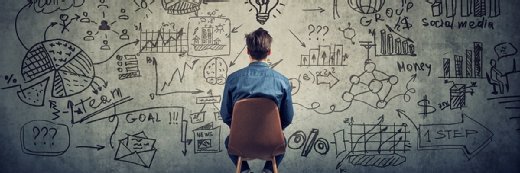
pathdoc - stock.adobe.com
Machine Learning, Whole Genome Sequencing Detect Disease Outbreaks
To prevent healthcare-quired infections, researchers are using machine learning and whole-genome sequencing to better identify potential infectious disease outbreaks.
University of Pittsburg School of Medicine and Carnegie Mellon University researchers are using machine learning and whole-genome sequencing to improve the detection of infectious disease outbreaks.
“The current method used by hospitals to find and stop infectious disease transmission among patients is antiquated. These practices haven’t changed significantly in over a century,” senior author and professor of infectious diseases at Pitt’s School of Medicine and epidemiology at Pitt’s Graduate School of Public Health, Lee Harrison, MD, said in a press release.
“Our process detects important outbreaks that would otherwise fly under the radar of traditional infection prevention monitoring.”
The Enhanced Detection System for Healthcare-Associated Transmission (EDS-HAT) combines genomic sequencing and machine learning connected to vast data in electronic health records. For example, when the sequencing identifies two or more patients in a hospital with nearly identical strains of infection, machine learning quickly mines those patients’ electronic health records for commonalities.
Typically, this process requires clinicians to notice that two or more patients shave a similar infection and alert their infection prevention team.
“This is an incredibly labor-intensive process that is often dependent upon busy health care workers noticing a shared infection between patients to begin with,” said lead author Alexander Sundermann, MPH, CIC, FAPIC, a clinical research coordinator and doctoral candidate at Pitt Public Health.
“That might work if patients are in the same unit of a hospital, but if those patients are in different units with different health care teams and the only shared link was a visit to a procedure room, the chances of that outbreak being detected before other patients are infected falls dramatically.”
From November 2016 to November 2018, UPMC Presbyterian Hospital ran EDS-HAT with a six-month lag for a few select infectious pathogens often linked to healthcare-acquired infections nationwide while continuing with real-time, traditional infection prevention methods. The team then analyzed how will EDS-HAT performed.
According to researchers, EDS-HAT detected 99 clusters of similar infections in the two years and found at least one potential transmission route in 65.7 percent of the clusters. At the same time, infection prevention used whole-genome sequencing to assist in the investigation of 15 suspected outbreaks.
If EDS-HAT was running in real-time, researchers estimated that as many as 63 transmissions of infectious disease from one patient to another could have been prevented. Additionally, the technology could have saved the hospital as much as $692,000.
In one case study, EDS-HAT discovered an outbreak of vancomycin-resistant Enterococcus faecium was linked to an interventional radiology procedure that was being performed according to manufacture instructions. UPMC was then able to alert the manufacturer and prevent future outbreaks.
“In that case, EDS-HAT connected the dots between seemingly unconnected patient infections occurring in different hospital units, stopping that outbreak but also potentially preventing similar outbreaks at other hospitals,” Harrison said. “That example encapsulates the value of EDS-HAT.”
Researchers plan to introduce EDS-HAT in real-time at UMPC Presbyterian Hospital to improve future infection prevention and control programs. According to researchers, the original EDS-HAT will soon expand to include sequencing for respiratory viruses, including COVID-19.