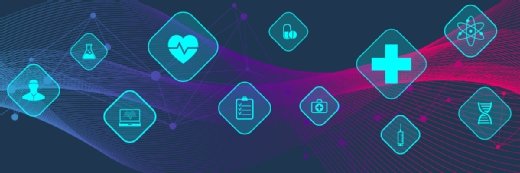
BAIVECTOR - stock.adobe.com
Machine Learning Technique Could Accelerate Drug Discovery
A new machine learning tool calculates the binding affinities between drug candidates and their targets.
A machine learning technique can quickly estimate the binding affinities between drug candidates and their targets, potentially accelerating drug discovery and protein engineering, a study published in the Journal of Physical Chemistry Letters revealed.
Researchers noted that drugs can only work if they stick to their target proteins in the body. Assessing that stickiness is challenging, and often hinders the drug discovery and screening process.
The affinity between a drug molecule and a target protein is measured by a quantity called the binding free energy. The smaller the number, the stickier the bind.
"A lower binding free energy means the drug can better compete against other molecules, meaning it can more effectively disrupt the protein's normal function," said Bin Zhang, the Pfizer-Laubach Career Development Professor in Chemistry at MIT, an associate member of the Broad Institute of MIT and Harvard, and a co-author of a new paper describing the technique.
Calculating the binding free energy of a drug candidate provides an indicator of a drug’s potential effectiveness. However, this is a difficult quantity to calculate.
Current methods of computing binding free energy fall into two broad categories. The first category calculates the quantity exactly, which takes significant time and computer resources. While the second category is less computationally expensive, it yields only an approximation of the binding free energy.
Researchers from MIT set out to develop a method that would combine traditional chemistry calculations with recent advances in machine learning. The new technique, called DeepBAR, computes binding free energy exactly but requires only a fraction of the calculations demanded by previous methods.
The BAR in DeepBAR stands for Bennett acceptance ratio, an algorithm used in exact calculations of binding free energy. The Bennett acceptance ratio typically requires knowledge of two endpoint states – a drug molecule bound to a protein and a drug molecule completely dissociated from a protein – as well as knowledge of many intermediate states, all of which slow down calculation speed.
DeepBAR deploys the Bennett acceptance ratio in machine learning frameworks called deep generative models.
"These models create a reference state for each endpoint, the bound state and the unbound state," said Zhang.
These two reference states are similar enough that the Bennett acceptance ratio can be used directly without all the costly intermediate steps.
Deep generative models are typically used in the field of computer vision, researchers noted.
"It's basically the same model that people use to do computer image synthensis," said Zhang. "We're sort of treating each molecular structure as an image, which the model can learn. So, this project is building on the effort of the machine learning community."
However, adapting a computer vision approach to chemistry did bring some challenges.
"These models were originally developed for 2D images," said Xinqiang Ding, a postdoc in MIT's Department of Chemistry. "But here we have proteins and molecules -- it's really a 3D structure. So, adapting those methods in our case was the biggest technical challenge we had to overcome."
In tests using small protein-like molecules, DeepBAR calculated binding free energy nearly 50 times faster than previous methods.
"Our method is orders of magnitude faster than before, meaning we can have drug discovery that is both efficient and reliable," said Zhang.
"We can really start to think about using this to do drug screening, in particular in the context of COVID-19. DeepBAR has the exact same accuracy as the gold standard, but it's much faster.”
The team also noted that in addition to drug screening, DeepBAR could help protein design and engineering because scientists could use the method to model interactions between multiple proteins.
Going forward, the researchers plan to improve DeepBAR’s ability to run calculations for large proteins, a task made possible by recent advances in computer science.
"This research is an example of combining traditional computational chemistry methods, developed over decades, with the latest developments in machine learning," Ding concluded. "So, we achieved something that would have been impossible before now."