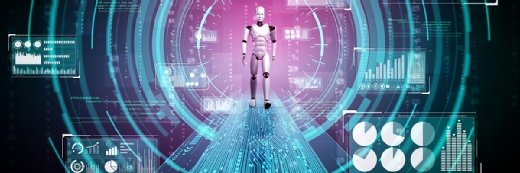
Getty Images
Machine Learning Uses EHR Data to Predict Suicide Attempt Risk
After analyzing EHR data, a machine learning algorithm can accurately predict suicide attempt risk in patients.
A machine learning tool can analyze EHR data to calculate suicide attempt risk and help providers know which patients to screen in nonpsychiatric clinical settings, a study published in JAMA Network Open revealed.
Researchers noted that suicide has been on the rise in the US for a generation, and is estimated to take the lives of 14 in 100,000 Americans each year. It’s the nation’s tenth leading cause of death, and some 8.5 percent of suicide attempts end in death nationally.
In some settings, universal screening might reduce the risk of downstream suicidality. However, the team pointed out that in-person screening takes time and attention, and clinicians conduct these screenings with variable quality.
Researchers from Vanderbilt University Medical Center (VUMC) developed a machine learning algorithm that uses EHR data to predict suicide attempt risk. The model recently underwent a prospective trial at the institution.
Over the course of 11 months – concluding in April 2020 – predictions ran silently in the background as providers saw adult patients at VUMC. Called the Vanderbilt Suicide Attempt and Ideation Likelihood (VSAIL) model, the algorithm uses routine information from the EHR to calculate 30-day risk of return visits for suicide attempt, and by extension, suicidal ideation.
Upon stratifying adult patients into eight groups according to their risk scores per the algorithm, researchers found that the top stratum alone accounted for more than one-third of all suicide attempts documented in the study and approximately half of all cases of suicidal ideation.
As documented in the EHR, one in 23 of these high-risk individuals went on to report suicidal thoughts, and one in 271 went on to attempt suicide.
"Today across the Medical Center, we cannot screen every patient for suicide risk in every encounter -- nor should we," said Colin Walsh, MD, MA, assistant professor of Biomedical Informatics, Medicine and Psychiatry.
"But we know some individuals are never screened despite factors that might put them at higher risk. This risk model is a first pass at that screening and might suggest which patients to screen further in settings where suicidality is not often discussed."
Over the 11-month test, some 78,000 adult patients were seen in the hospital, emergency room, and surgical clinics at VUMC. As subsequently documented in the EHR, 395 individuals in this group reported having suicidal thoughts and 85 lived through at least one suicide attempt, with 23 surviving repeated attempts.
"Here, for every 271 people identified in the highest predicted risk group, one returned for treatment for a suicide attempt," Walsh said.
"This number is on a par with numbers needed to screen for problems like abnormal cholesterol and certain cancers. We might feasibly ask hundreds or even thousands of individuals about suicidal thinking, but we cannot ask the millions who visit our Medical Center every year -- and not all patients need to be asked. Our results suggest artificial intelligence might help as one step in directing limited clinical resources to where they are most needed."
Walsh and his team had previously validated the machine learning algorithm using retrospective EHR data from VUMC.
"Dr. Walsh and his team have shown how to stress test and adapt an artificial intelligence predictive model in an operational electronic health record, paving the way to real world testing of decision support interventions," said the new study's senior author, William Stead, MD, professor of Biomedical Informatics.
AI and data analytics tools are on the rise in the mental healthcare space. A recent study published in JAMA Psychiatry showed that a universal screening tool could leverage predictive analytics algorithms to accurately determine an adolescent’s suicide risk. The algorithm could also alert providers of which patients are in need of follow-up interventions.
Unlike existing tools, the model asks adolescents not only about suicidal thoughts but also about other factors that may put them at risk, including sleep disturbance, trouble concentrating, agitation, and issues with family and school connectedness.
“Different combinations of risk factors can place youth at risk. If we screen only for suicidal thoughts, we will miss some high-risk adolescents,” said lead author Cheryl King, PhD, a professor, clinical child psychologist, and director of the Youth and Young Adult Depression and Suicide Prevention Research Program in the Department of Psychiatry at Michigan Medicine.
“There are many reasons young people may not share suicidal thoughts, possibly because they're ashamed, they aren't experiencing the thoughts at the time of screening, or someone reacted in a way they didn't feel was helpful when they shared suicidal thoughts or sensitive information in the past.”