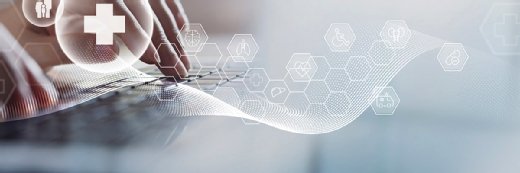
tippapatt - stock.adobe.com
Using Predictive Analytics to Stay Ahead of Patient Deterioration
Researchers from Michigan Medicine and Fifth Eye have developed a predictive analytics tool that can alert providers which patients are at risk of deteriorating.
In hopsitals and emergency departments, it’s essential for providers to continually monitor patients in order to stay ahead of potential adverse events. However, patient deterioration can be very difficult to anticipate, especially for clinicians working in fast-paced critical care settings.
At the Michigan Center for Integrative Research in Clinical Care (MCIRCC) and Fifth Eye, researchers recognized that nurses and physicians need a better way to consistently and reliably monitor patients.
The team developed a predictive analytics tool, called the Analytic for Hemodynamic Instability (AHI), which uses real-time streaming data to alert providers which patients are at high risk of deteriorating.
“The need to develop a tool like this has always existed,” Ashwin Belle, PhD, former analytics architect at MCIRCC and chief analytics officer and cofounder at Fifth Eye, told HealthITAnalytics.
“There’s typically a nurse-to-patient ratio when it comes to monitoring patients, but that ratio can be quite lopsided – especially in situations like COVID-19. It can be very difficult to make sure that all patients are stable and trending in the right direction, and to identify patients who are deteriorating.”
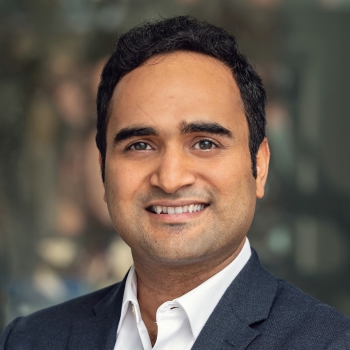
The noninvasive clinical tool established an automated way of monitoring patients for hemodynamic instability, one of the most common causes of death for critically ill or injured patients.
“Hemodynamic instability can happen irrespective of a patient’s condition. Whether a patient is hemorrhaging because of an injury, or a patient is septic or has some sort of infection, these kinds of things can lead to hemodynamic instability,” Belle said.
“Today, clinicians have very few tools in their hand to be able to see this coming. It can come on really quickly for some patients, while it takes much longer for others. To be able to see that this is happening at the onset is extremely important.”
The current method of forecasting hemodynamic instability requires clinicians to sporadically check a patient’s heart rate and blood pressure. But this isn’t done consistently, and it’s done more often for patients who seem to be sicker than others -- resulting in missed signs of instability.
With AHI, researchers sought to provide clinicians with a more efficient and effective way of monitoring patients for impending adverse events. The team also aimed to develop a solution that would lighten providers’ workload – not add to it.
“There are many early warning systems out there that tend to use a lot of different vital signs and different inputs from the EHR to identify a patient's deterioration. But the problem with those kinds of systems is that they rely heavily on data that is collected manually and infrequently,” Belle noted.
“From the very beginning, we wanted to try and see if we could develop an early warning system without adding any burden to the clinician's workflow. With that in mind, we started looking at a variety of continuous physiological monitoring systems that already exist.”
When the researchers looked at the different patient signals that clinicians typically collect, they realized that not all of them are informative of hemodynamic status.
“We started focusing more and more on identifying the simplest, most informative signal that we could get. In that effort, we landed on the single-lead of ECG, particularly lead 2 ECG,” Belle said.
“We realized that if we analyzed it a certain way, and if we trained the machine learning models to catch this but understand the differences in different stations, we would be able to recognize emerging signs of hemodynamic instability.”
Focusing on data from a single source significantly contributed to the success of the predictive analytics tool, the researchers found.
“There is tremendous enthusiasm around collecting large datasets from patients and trying to see if we can find the needle in the haystack, so to speak. But healthcare data is notorious for being noisy and imperfect. That’s because it comes from the real world, from patients of different walks of life who have different types of issues,” Belle said.
“When you're trying to recognize a pattern or theme that is consistent across variety of data coming from a variety of patients, it's not going to be very fruitful. We’ve seen that play out over and over again with a lot of analytics tools that are being developed right now and used in hospitals where they’re working inconsistently.”
The team also involved providers in the development process, ensuring the tool would fit seamlessly into clinical workflows.
“Just as healthcare data scientists and researchers are trying to collect a lot of data and solve a lot of problems, clinicians are also getting thrown a lot of data. They’re often inundated by the amount of information they have to consume in order to treat patients. We did not want to become another alarm contributing to that fatigue,” said Belle.
“We were very lucky to be able to design this with clinicians. In fact, the majority of this design and development happened alongside a variety of clinicians because it was embedded within the Department of Emergency Medicine.”
The team found that the predictive tool can distinguish hemodynamic instability with 100 percent accuracy, and it can do so with a median lead time of 9.5 hours before it became obvious that bedside clinicians needed to call the rapid response team.
“These rapid response teams are usually a multidisciplinary team of doctors and nurses with a variety of unique skillsets who try and rescue the patient and then make sure they're prepped and ready for whatever needs to be done next,” Belle said.
“These cases can be very expensive and very drastic, so it’s important to be able to identify emerging cases of hemodynamic instability that lead to rapid response team calls.”
The team expects that the AHI tool could help improve outcomes in real-world critical care units across the country.
“This analysis gave us a tremendous amount of optimism. Going forward, we’re going to see how we can actually apply the technology in clinical settings to identify drastic patient deterioration, which can sometimes go unnoticed,” Belle concluded.