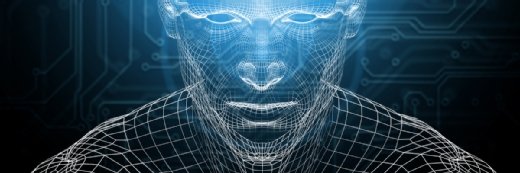
Getty Images
Predictive Analytics Model Could Reduce Rates of MRI No-Shows
A predictive analytics algorithm was able to cut down on MRI outpatient appointment no-shows, leading to improved efficiency.
Using a limited amount of data and basic feature engineering, a predictive analytics model could effectively reduce the rate of MRI appointment no-shows, according to a study published in the American Journal of Roentgenology (AJR).
Outpatient appointment no-shows are a prevalent problem, the researchers noted. Appointment no-shows are associated with substantial reimbursement losses and can create significant administration and workflow issues. With predictive analytics, clinicians can potentially implement targeted interventions that can improve efficiency.
To train the predictive analytics model, researchers extracted records from 32,957 MRI appointments scheduled between January 2016 and December 2018 from their institution’s radiology information system. The team also acquired a further holdout test set of 1,080 records from January 2019. Overall, the no-show rate was 17.4 percent.
Researchers then deployed a predictive analytics algorithm that identified patients with the highest risk of appointment no-shows. The team used the intervention method of telephone call reminders for patients with the top 25 percent highest risk of an appointment no-show as predicted by the model, and used this approach over six months.
Six months after deployment, the no-show rate of the predictive model was 15.9 percent, compared with 19.3 percent in the preceding 12-month preintervention period. This percentage corresponded to a 17.2 percent improvement from the baseline no-show rate. The no-shows of contactable patients at high risk of appointment no-shows as predicted by the model were 17.5 percent, while no-shows of noncontactable patients were 40.3 percent.
The findings show that providers can cut down on appointment no-shows using a modest amount of data and basic technology, researchers stated.
“Such data may be readily retrievable from frontline information technology systems commonly used in most hospital radiology departments, and they can be readily incorporated into routine workflow practice to improve the efficiency and quality of health care delivery,” said lead author Le Roy Chong of Singapore’s Changi General Hospital.
Predictive models like these could significantly improve clinical workflows, resulting in fewer appointment no-shows and increased efficiency.
“We believe that the main strength of the present study lies in its empirical approach, given the lack of published literature quantifying the impact of actual workflow implementation, with previous studies postulating the potential benefits of applying machine learning techniques to this problem,” the researchers concluded.
“The aim of our study was not to produce a highly complex model but, rather, to produce one that could be developed relatively quickly, would require minimal data processing, and would be readily deployable in workflow practice for quality improvement.”
Previous studies have demonstrated the benefit of using predictive models to reduce the rate of appointment no-shows. In 2018, a team from Duke University used predictive analytics and EHR data to capture 4819 previously unidentified patient no-shows within the Duke health system. This could maximize the use of clinician hours and allow care sites to operate more efficiently.
Similar to the team in the AJR study, Duke researchers emphasized that using data from smaller, more specific patient populations could increase the accuracy of appointment no-show predictions.
“Many EHR vendors are offering prediction models out of the box without requiring or recommending clients to do any retraining with local data,” the researchers stated.
“The heterogeneity seen within our dataset suggests that local validation and recalibration would lead to better performance and should be part of the implementation of these off-the-shelf models.”