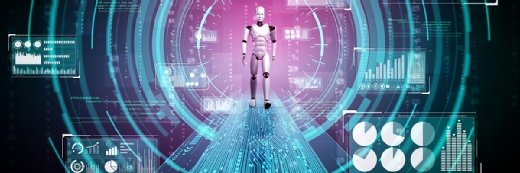
Getty Images
Machine Learning Reveals Traits of High Mental Health Utilizers
Machine learning tools analyzed EHR data and identified characteristics of high utilizers for mental health services.
After applying machine learning to EHR data, researchers found that dropping out of high school, having schizophrenia, or being diagnosed with a co-occurring personality disorder increases the likelihood of someone becoming a high utilizer of mental health services.
The study, published in the Journal of Health Care for the Poor and Underserved, is the first of its kind to examine high utilizer trends in an academic safety net psychiatric hospital in a large, diverse region.
A high utilizer is someone who has been admitted to an inpatient psychiatric hospital three or more times within one year. According to the research team, evidence suggests that high utilizers of the healthcare system are more likely to have mental illness, to be from socially disadvantaged groups, and to have limited access to community-based services.
“Many people don’t realize that half of all healthcare expenses in the US are incurred by five percent of the population,” said Jane Hamilton, PhD, MPH, assistant professor in the Louis A. Faillace, MD, Department of Psychiatry and Behavioral Sciences at McGovern Medical School at UTHealth and first author of the study.
“These high utilizers are a very small number of individuals who are consuming a high number of resources. We need to figure out why they keep coming back so we can put supports around them to stop the trend.”
Researchers used machine learning to analyze deidentified EHR data from 9,840 patients admitted to UTHealth Harris County Psychiatric Center from January 2014 to December 2016. The machine learning algorithm helped the team analyze all factors at once, rather than examining each factor’s independent relationship to utilization.
“A machine learning algorithm called the ‘elastic net’ was able to predict utilization by including all of the predictors in the model at the same time,” said Robert Suchting, PhD, assistant professor in the Faillace Department of Psychiatry and Behavioral Sciences and study co-author.
“Traditionally, including all of the predictors at the same time can lead to unstable estimates of the strength of each predictor’s relationship. The elastic net quantifies each predictor’s relationship to the outcome, making it much easier to determine which predictors are strongest.”
Researchers identified years of education, a schizophrenia diagnosis, and a co-occurring personality disorder diagnosis (being diagnosed with a personality disorder and another psychiatric condition simultaneously) as the top predictors of high utilization.
After identifying these characteristics, the group was able to highlight suggestions for each predictor. For example, when working with psychiatric patients with limited education, researchers recommended that mental health providers routinely assess for mental health literacy. Providers should also aim to connect patients with educational support programs to improve health outcomes.
For patients who are experiencing a first episode of psychosis or schizophrenia, researchers stated that providers should leverage enhanced efforts and prioritization for better identification and treatment at this stage of illness.
The group also noted that the routine assessment and treatment for co-occurring personality disorders should be integrated into community-based psychiatric treatment.
“Both schizophrenia and personality disorders can be difficult to treat, and many patients with these diagnoses are disadvantaged and vulnerable to health disparities. We really need evidence-based treatments in place to help these patients avoid repeat hospitalizations, and this study is a great first step in helping to identify the appropriate outpatient resources to help these patients remain stable in the community and avoid repeat hospitalizations,” Hamilton said.
Going forward, the research team will strategize how to use the study’s results to improve care transitions for these patients as they move from inpatient to outpatient care.
“Rehospitalization in a psychiatric hospital affects patient care and adds financial burden to our current healthcare system,” said Lokesh Shahani, MD, MPH, an assistant professor in the Faillace Department of Psychiatry and Behavioral Sciences.
“Using results from this current study, we will be able to better identify patients at high risk for rehospitalization. This would help us design and provide new treatment modalities to reduce their likelihood of future hospitalization.”