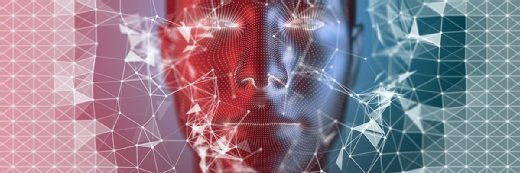
Getty Images
Machine Learning Tool Predicts Mortality During Transfers of Care
A new web-based tool developed using machine learning predicts mortality rates based on hospital transfers, leading to better patient outcomes.
Researchers at UPMC and the University of Pittsburgh School of Medicine have leveraged machine learning to develop a tool that predicts mortality for patients facing transfer between hospitals in order to access higher-acuity care, according to a study published in PLOS One.
Every year, almost 1.6 million patients—or 3.5 percent of all inpatient admissions—are transferred from one hospital to another to access specialized care.
"Securing these services often requires burdensome travel and reduced community support for patients and their families,” said Daniel E. Hall, MD, corresponding author of the study, medical director of high-risk populations and outcomes at the UPMC Wolff Center, and associate professor of surgery at Pitt's School of Medicine.
“While some may recover from the acute illness, many others realize little benefit in terms of improved outcomes," Hall continued.
Using data from approximately 21,000 patients ages 18 and older who were transferred to a UPMC hospital during a 12-month period, the researchers developed and certified a mortality risk-assessment tool called "SafeNET" (Safe Nonelective Emergent Transfers).
The new technology could help avoid unnecessary transfers. When a clinician uses the tool to predict mortality outcomes for patients at the time of hospital transfer, she may be able to determine whether it is best to transfer the patient or keep the patient in the current facility.
Hall and his team put together a list of 70 independent variables featured in one or more mortality risk models currently used in hospital settings. These factors included patient demographics, vital signs, lab tests, and other measures.
Next, the researchers analyzed UPMC billing data and inpatient EHRs to determine if these variables were recorded by the receiving hospital. Hall and his team focused only on the information the hospital received within three hours of transfer. That brought the list from 70 variables to 54.
Ultimately, the team constructed the SafeNET algorithm made up of 14 variables that reliably and rapidly predict in-hospital, 30-day, and 90-day mortality for transferred patients. The researchers used guided machine learning on both training and test sets of the data to develop the tool.
In comparison to similar tools for infection patients, SafeNET's predictive ability was not only more accurate, but it could also be used across a wider patient population.
"Our overarching goal was to provide much-needed information to front-line physicians to trigger and inform shared decisions about the highest risk patients," said Hall. "This tool could help to direct additional resources to these patients to ensure that the plan of care is consistent with the patient's values and goals.
“Frequently, transferred patients and their families don't understand the severity of the illness they are facing and have unrealistic expectations about the outcomes that a transfer for higher-level care will produce,” Hall explained.
SafeNET guides users to enter as many of the variables that are available to the individual. Then, the tool generates a predicted mortality risk. The researchers found that even when at least one variable was missing, which occurred more than half the time, the tool accurately predicted mortality outcomes.
Hall noted that further research is needed before widely implementing SafeNET because the data were restricted to a single, multi-hospital system. Additionally, more investigation is needed to ensure the information that feeds the algorithm is not subject to bias.
SafeNET is being piloted in three hospitals within the UPMC system to build care pathways that direct resources to patients at risk of poor outcomes.
For example, the web-based tool is being used in studies to initiate goal-clarification conferences among clinicians contemplating a transfer between hospitals. Additionally, the tool can be used to deliver remote, video-conferenced palliative care services to patients being considered for transfer.
"SafeNET is not meant to supersede clinical judgement," Hall noted. "Instead, its intent is to trigger a 'pause' so that clinicians are better prepared to inform and guide patients and families, preferably before a critically ill patient is transferred.
“We know that patients or their surrogates who participate in conversations with physicians about their values tend to receive care that is consistent with their preferences. SafeNET may be an effective and inexpensive tool for facilitating those important conversations,” he continued.