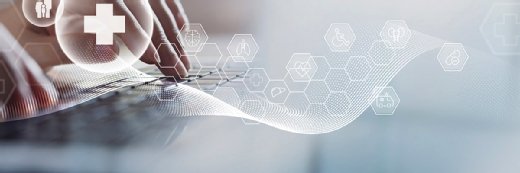
tippapatt - stock.adobe.com
Machine Learning Shows How Quarantine Measures Impact COVID-19 Rates
A machine learning model revealed a direct link between the number of people infected with COVID-19 and how effectively a state maintains quarantine measures.
Machine learning can help inform public policies around quarantine measures to reduce the spread of COVID-19, according to a study conducted by MIT researchers.
As COVID-19 cases continue to increase across the country, some states are beginning to tighten restrictions and re-implement quarantine measures to slow the spread of the virus.
The machine learning model is a modification of an epidemiological model used to predict the way a disease spreads, based on the number of people who are susceptible, infectious, or recovered. Researchers enhanced the epidemiological model with a neural network trained to process real COVID-19 data.
The newly enhanced model learns to identify patterns in data of infected and recovered cases, and from these data it quantifies the number of infected individuals who are not spreading the virus to others. Researchers labeled this value as quarantine strength, which reflects how successful a region is in quarantining an infected individual. The model can process data over time to see how a region’s quarantine strength evolves.
Since developing the model in early February, the research team has applied it to COVID-19 data from over 70 countries. The tool has accurately simulated the real-life quarantine situation in European, South American, and Asian countries that were initially significantly impacted by the virus.
“When we look at these countries to see when quarantines were instituted, and we compare that with results for the trained quarantine strength signal, we see a very strong correlation,” said co-author Christopher Rackauckas, an applied mathematics instructor at MIT.
“The quarantine strength in our model changes a day or two after policies are instituted, among all countries. Those results validated the model.”
The team published these country-level results in November. In the current study, researchers applied the model to individual states in the US to discover not only how a state’s quarantine measures evolved over time, but also how the number of infections would change if a state adjusted its quarantine strength.
Researchers focused on calculating the quarantine strength of Arizona, Florida, Louisiana, Nevada, Oklahoma, South Carolina, Tennessee, Texas, and Utah, all of which reopened before May 15. The group also modeled states that delayed reopening until late May or early June, including New York, New Jersey, and Illinois.
The team gave the model the number of infected and recovered individuals reported for each state, starting from when the 500th infection was reported in each state, up until mid-July. Researchers also noted the day on which each state’s stay-at-home order was lifted, signaling the state’s reopening.
The results showed that in every state, quarantine strength declined soon after reopening. The steepness of this decline, as well as the subsequent rise in infections, was strongly related to a state’s reopening. States that reopened early, like South Carolina or Tennessee, experienced a steeper drop in quarantine strength and a higher daily rate of cases.
“If you look at these numbers, simple actions on an individual level can lead to huge reductions in the number of infections and can massively influence the global statistics of this pandemic,” said lead author Raj Dandekar, a graduate in MIT's Department of Civil and Environmental Engineering.
“Instead of just saying that reopening early is bad, we are actually quantifying here how bad it was.”
Conversely, states that delayed reopening like New York and New Jersey managed to keep a steady quarantine strength, with no sharp rise in infections.
Researchers then reversed the model to quantify the number of infections that would have occurred if a given state maintained a steady quarantine strength even after reopening. The group saw that in this situation, over 40 percent of infections could have been avoided in each state they studied.
The findings demonstrate the effectiveness of consistent pandemic measures and guidelines in slowing the spread of the virus, the team noted.
“Now that we can give a measure of quarantine strength that matches reality, we can say, ‘What if we kept everything constant? How much difference would the southern states have had in their outlook?’” Rackauckas said.
“What I think we have learned quantitatively is, jumping around from hyper-quarantine to no quarantine and back to hyper-quarantine definitely doesn’t work. Instead, good consistent application of policy would have been a much more effective tool.”
The team expects that policymakers could use the model to calculate the quarantine strength necessary to keep a state’s current infections below a particular number. They could then look through the data to a point in time where the state displayed this same value, and look back at the type of restrictions that were in place at the time. This could help inform new policies and measures as the pandemic continues.
“What is the rate of growth of the disease that we’re comfortable with, and what would be the quarantine policies that would get us there?” Rackauckas said.
“Is it everyone holing up in their houses, or is it everyone allowed to go to restaurants, but once a week? That’s what the model can kind of tell us. It can give us more of a refined quantitative view of that question.”