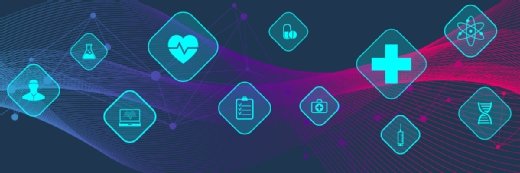
BAIVECTOR - stock.adobe.com
Real-Time Clinical Decision Support Tool Helps Reduce Mortality
A real-time clinical decision support system analyzes EHR data to identify patients at risk of deteriorating.
A real-time clinical decision support system issues alerts to specially trained nurses when patients are at risk of deteriorating, potentially leading to lower mortality rates, according to a study published in the New England Journal of Medicine.
The Advance Alert Monitor (AAM) uses machine learning algorithms and data from more than 1.5 million patients to predict the probability that a hospitalized patient is likely to decline, require transfer to the intensive care unit or emergency resuscitation, and benefit from interventions.
The model uses severity-of-illness and longitudinal comorbidity scores, vital signs and vital signs trends, neurological status checks, and laboratory tests. The alert system scans hospitals’ EHRs on an hourly basis. If a patient’s score is above threshold, which indicates a significant risk of decline over the next 12 hours, the system issues an alert.
The alert is initially reviewed by a regional team of specially trained registered nurses that evaluates the alert using information from the patient’s medical record to determine if on-site intervention is necessary.
The nurses then contact a rapid response team on that hospital unit, which performs a structured assessment and works with the patient’s physician to determine further action.
Researchers first tested the system in 2013 and implemented the model in all 21 Kaiser Permanente Northern California hospitals between 2016 and 2019. The current study compared patients with and without AAM in place, and found that the system was associated with better outcomes within 30 days of an alert.
The results showed that patients in the intervention cohort had lower ICU admission rates (17.7 percent) than patients without AAM in place (20.9 percent). Additionally, intervention cohort patients had shorter hospital length of stay compared to patients without AAM (6.7 days versus 7.5 days).
Patients with AAM also had lower mortality within 30 days of an alert, at 15.8 percent compared to 20.4 percent of patients without AAM. Moreover, patients with an AAM alert were less likely to die without a palliative care referral.
Researchers noted that these results were made possible not just by cutting edge technology, but also system integration, workflow development, and collaborations among physicians, nurses, and other caregivers.
"Along with saving lives, the Advance Alert Monitor has demonstrated that it is possible to integrate predictive models into day-to-day operations in our medical centers," said lead author Gabriel Escobar, MD, a research scientist with the Kaiser Permanente Division of Research and regional director for Kaiser Permanente Northern California hospital operations research.
AAM is different from many other clinical decision support systems in that it leverages machine learning and predictive analytics techniques to evaluate many patient status factors. The system is also automated, so it doesn’t require manual risk calculation by hospital staff. And because the alerts are curated by trained nurses off-site, bedside caregivers don’t get unnecessary interruptions.
"Predictive analytics and machine learning are unlocking new frontiers in the use of complex patient data to improve our care in real time. They augment our clinicians' practice by finding signals hidden within the electronic health record," said coauthor Vincent Liu, MD, MS, a practicing intensivist and research scientist with the Division of Research, and regional director for Kaiser Permanente Northern California hospital advanced analytics.
The study’s results demonstrate the potential for this clinical decision support tool to provide clinicians with early warnings of deterioration or decline, leading to earlier interventions and better patient outcomes.
"The Advance Alert Monitor program is a wonderful example of how we combine high-tech and high-touch in caring for hospitalized patients," said Stephen Parodi, MD, national infectious disease lead for Kaiser Permanente.
"This study's findings support an intervention employing both cutting-edge data analysis and the judgment of our top-notch professional nursing staff to identify patients who need immediate attention."