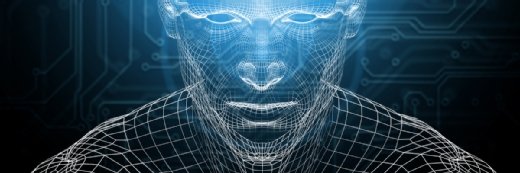
Getty Images
Predictive Analytics Tool Helps Fill Gaps in COVID-19 Data
Predictive analytics models can help public health officials complete missing demographic information, leading to more comprehensive COVID-19 data.
A predictive analytics tool has helped public health leaders in Chicago improve the quality of COVID-19 data, reducing the category of “unknown” race in tests from 47 percent to 11 percent.
For more coronavirus updates, visit our resource page, updated twice daily by Xtelligent Healthcare Media.
While thousands of people are being tested for COVID-19 every day, collecting complete demographic information can be difficult. This presents a critical issue for the healthcare industry, as incomplete race and ethnicity data can cast a shadow over the disparities minority and underrepresented populations are experiencing during the pandemic.
“This information is essential for understanding inequities with COVID-19,” said Fernando De Maio, professor of sociology and founding co-director of the Center for Community Health Equity at DePaul University.
“Everyone is struggling with missing data, but from what is already available, we know that the burden has been carried in disproportionate ways by minoritized and marginalized communities.”
Researchers at DePaul used a predictive analytics algorithm to analyze US census data and available. The team is able to predict a Chicago individual’s race and ethnicity with 81 percent accuracy. Researchers also developed a mobile app to help city officials easily and securely input the data with missing values.
The results of the data imputation process enabled public health officials to get a clearer view of the racial and ethnic inequities occurring during the COVID-19 pandemic.
“By filling in the missing race/ethnicity data of those testing for COVID-19, CDPH and the city’s Racial Equity Rapid Response Team will be able to better pinpoint and prioritize testing, PPE distribution, community education and stakeholder engagement in our overall COVID response. This was not strictly an epidemiological exercise,” said Margarita Reina, a senior epidemiologist at the Chicago Department of Public Health.
Deep-rooted racial segregation was partially what made the predictive analytics model so successful, researchers noted.
“We had someone’s last name and we had the address of their residence. With just those two pieces of information, we can predict their race and ethnicity with a very high degree of accuracy,” De Maio said.
The team used an analytics method that was able to predict patients’ unreported racial information based on their surname and geocoding. Researchers first tested the tool’s accuracy by using data for which the race and ethnicity of an individual was known. The group then gave the app over to public health officials to make sure it worked for them.
“We worked together to see how sensitive the model was at various geographic scales,” said C. Scott Smith, assistant director of the Chaddick Institute for Metropolitan Development at DePaul.
“City epidemiologists and public health officials know the zip codes very well, and could confirm that the process made sense. The collaboration also led to conversations about how this new information could change what we know about how the virus is moving through the city.”
By partnering with experts in sectors besides healthcare, leaders now have a better understanding of how the spread of the virus is influenced by non-clinical factors.
“The pandemic is affecting many different sectors and facets of our lives, including urban planning. We now see that transportation has played a key role in coronavirus-related health outcomes, from access to testing facilities to how urban design impacts probabilities of transmission. That's something we're looking at now,” Smith said.
Going forward, the researchers are seeking to expand the application so it can be used in other cities.
“We’re happy to help and it’s good that we can, but it’s also a sign of our nation’s under-funded public health system,” said De Maio.
“And while we at DePaul have come up with a very practical solution, it doesn't fix the underlying problem. We need to do a better job of funding critical public health infrastructure. And we need to do more to make sure that equity-focused data analysis is always a priority.”