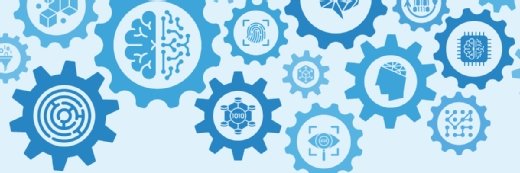
Getty Images
3 Ways Healthcare is Using Predictive Analytics to Combat COVID-19
Predictive analytics tools are helping healthcare organizations stay ahead of poor outcomes, resource shortages, and other impacts of COVID-19.
Throughout the COVID-19 pandemic, predictive analytics tools have played an integral part in healthcare organizations’ response to and defense against the virus.
For more coronavirus updates, visit our resource page, updated twice daily by Xtelligent Healthcare Media.
The enormous amount of data the pandemic has generated has given researchers and providers the opportunity to analyze trends, monitor patient populations, and begin to rectify longstanding issues in the healthcare industry. Having the ability to anticipate future events is critical in the midst of a healthcare crisis, and predictive analytics tools can help healthcare entities do just that.
Organizations are increasingly turning to predictive models to better understand which patients are at risk, where resources are most needed, and where the disease is likely to spike next.
Forecasting disease severity, risk
Determining which patients are most at risk for contracting the virus – as well as which individuals are likely to experience poor outcomes from COVID-19 – is perhaps the most important use case for predictive analytics during the pandemic.
Researchers have worked to uncover the factors that may influence disease susceptibility and severity, aiming to get ahead of surges in cases, hospitalizations, and deaths.
A team from Mount Sinai recently created a predictive analytics model based on three clinical features: age, minimum oxygen saturation, and type of patient encounter. The results showed that these three features can accurately classify COVID-19 patients as likely to live or die.
“Applying machine learning approaches to data from a large cohort of patients with COVID-19 resulted in the identification of accurate and parsimonious prediction models of mortality,” researchers said.
“After extensive validation in other datasets and health systems, these data-driven findings might help clinicians better recognize and prioritize the care of patients at greatest risk of death.”
In June 2020, Cleveland Clinic researchers set out to achieve a similar endeavor. The group developed a predictive analytics model to determine an individual patient’s likelihood of testing positive for COVID-19, as well as their potential outcomes from the disease.
Researchers on this study found that patients who had received the pneumococcal polysaccharide vaccine (PPSV23) and the flu vaccine are less likely to test positive for COVID-19 than those who haven’t received the vaccinations.
Additionally, individuals actively taking melatonin (a sleep aid), carvedilol (high blood pressure or heart failure medication), or paroxetine (an anti-depressant) are less likely to test positive than patients not taking these drugs.
The team also found that patients of low socioeconomic status, as measured by zip code in this study, are more likely to test positive than patients of greater economic means. The study showed that patients of Asian descent are less likely to test positive than Caucasian patients as well.
“The ability to accurately predict whether or not a patient is likely to test positive for COVID-19, as well as potential outcomes including disease severity and hospitalization, will be paramount in effectively managing our resources and triaging care,” said Lara Jehi, MD, Cleveland Clinic's Chief Research Information Officer and corresponding author on the study.
“As we continue to battle this pandemic and prepare for a potential second wave, understanding a person's risk is the first step in potential care and treatment planning.”
A team from Johns Hopkins University School of Medicine have sought to use analytics tools to predict COVID-19 outcomes as well. Researchers recently developed a predictive analytics model that could forecast how likely a patient’s condition is to worsen while in the hospital. Among the risk factors included were a patient’s age, body mass index, lung health and chronic disease, and vital signs.
Planning for hospital constraints, demands
With the rapid spread of COVID-19, many hospitals and health systems were faced with the possibility of sudden surges in patient volume, resulting in limited resources and increased burden on staff.
To better plan for these potential surges, organizations have implemented predictive tools that can help allocate resources.
In late April 2020, Cleveland Clinic researchers created a predictive model that can forecast patient volume, bed capacity, ventilator availability, and other metrics. The model provides timely, reliable information to optimize care delivery for COVID-19 and other patients.
Predicting the needs of hospital staff is also a critical aspect of the pandemic. A team from Cedars-Sinai developed a machine learning tool that can forecast data points related to the COVID-19 pandemic and predict staffing needs.
The platform tracks local hospitalization volumes and the rate of confirmed COVID-19 cases, running multiple forecasting models to help anticipate and prepare for increasing COVID-19 patient volumes with an 85 percent to 95 percent degree of accuracy.
“Our goal is to have the capacity and the right care available every day to treat the patients who need us, which fluctuates on a daily basis,” said Michael Thompson, executive director of Enterprise Data Intelligence at Cedars-Sinai, which developed the platform and runs the forecasts. “We need to match that daily demand with the necessary resources: beds, staff, PPE and other supplies.”
The threat posed by COVID-19 has also caused many providers to defer surgeries and other procedures for unknown periods of time. For patients with complex, serious conditions like cancer, this is an especially challenging time, as doctors have to compare the long-term risk of care postponements with the risk of patients potentially contracting COVID-19.
To better prioritize cancer care, data scientists and oncologists from the University of Michigan Cancer Center and the U-M School of Public Health have teamed up to develop a free, web-based application that helps assess the risk form of delayed treatment versus immediate treatment, based on patients’ individual characteristics.
“We noticed that we had these tiered systems that go by disease site and stage, but they don’t really consider anything else about the patient – factors like their age or their location,” Holly Hartman, lead researcher for the project and a doctoral student in biostatistics at U-M, told HealthITAnalytics.
“We wanted to take a more nuanced approach to deciding treatment for these cancer patients. Especially since we don’t know how long this pandemic will be going on, we need to better understand how delaying treatment is going to impact these patients. We developed this app to address that need.”
Mapping the spread of the virus
In a situation as unpredictable as a global health crisis, having some idea of which areas will be most impacted can help public health officials and providers get ahead of poor outcomes.
Using analytics tools, researchers can find patterns and trends in different places that can inform social distancing guidelines and other measures.
A team from Binghamton University, State University of New York recently developed several predictive analytics models to examine COVID-19 trends and patterns around the world.
The machine learning algorithms analyze trends from the 50 countries that have the highest COVID-19 rates, including the US, and can often predict within a ten percent margin of error what will happen for the next three days based on data from the past 14 days.
“We believe that the past data encodes all of the necessary information,” said Anand Seetharam, assistant professor in the department of computer science at Binghamton University.
“These infections have spread because of measures that have been implemented or not implemented, and also because how some people have been adhering to restrictions or not. Different countries around the world have different levels of restrictions and socio-economic status.”
Predictive models could also demonstrate the importance of other COVID-19 regulations, such as wearing masks. A study published in the journal Physics of Fluids leveraged predictive models to show that without masks, six feet of social distance may not be enough to keep one person’s respiratory droplets from reaching someone else, which could contribute to the spread of the virus.
“The basic fundamental form of a chemical reaction is two molecules are colliding. How frequently they’re colliding will give you how fast the reaction progresses,” said Abhishek Saha, a professor of mechanical engineering at the University of California San Diego, and one of the authors of the paper. “It’s exactly the same here; how frequently healthy people are coming in contact with an infected droplet cloud can be a measure of how fast the disease can spread.”
As the pandemic continues on, predictive analytics will continue to play a significant role in monitoring the impact of the virus, from patient outcomes to areas of increased disease spread. The global health crisis has only further highlighted the importance of predictive analytics in healthcare, and could accelerate the use of these tools in standard care going forward.