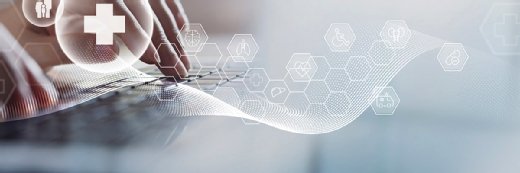
tippapatt - stock.adobe.com
Predictive Analytics Model Forecasts COVID-19 Risk, Outcomes
The tool is the world’s first predictive analytics model to forecast the risk of testing positive for COVID-19.
Cleveland Clinic researchers have developed a predictive analytics model to determine an individual patient’s likelihood of testing positive for COVID-19, as well as their potential outcomes from the disease.
For more coronavirus updates, visit our resource page, updated twice daily by Xtelligent Healthcare Media.
In a study published in CHEST, the researchers noted that the tool could provide a more scientific approach to testing, which will be essential as the healthcare industry faces increased demands for testing and limited resources.
"The ability to accurately predict whether or not a patient is likely to test positive for COVID-19, as well as potential outcomes including disease severity and hospitalization, will be paramount in effectively managing our resources and triaging care," said Lara Jehi, MD, Cleveland Clinic's Chief Research Information Officer and corresponding author on the study.
"As we continue to battle this pandemic and prepare for a potential second wave, understanding a person's risk is the first step in potential care and treatment planning."
Researchers developed the predictive analytics model, called a nomogram, using data from nearly 12,000 patients enrolled in Cleveland Clinic’s COVID-19 Registry, which includes all individuals tested at Cleveland Clinic for the disease – not just those that test positive.
Data scientists used statistical algorithms to transform data from registry patients’ electronic medical records into the first-of-its-kind nomogram.
The study revealed several key findings about disease risk. Researchers determined that people who have received the pneumococcal polysaccharide vaccine (PPSV23) and the flu vaccine are less likely to test positive for COVID-19 than those who haven’t received the vaccinations.
Additionally, individuals actively taking melatonin (a sleep aid), carvedilol (high blood pressure or heart failure medication), or paroxetine (an anti-depressant) are less likely to test positive than patients not taking these drugs.
The team also found that patients of low socioeconomic status, as measured by zip code in this study, are more likely to test positive than patients of greater economic means. The study showed that patients of Asian descent are less likely to test positive than Caucasian patients as well.
"Our findings corroborated several risk factors already reported in existing literature - including that being male and of advancing age both increase the likelihood of testing positive for COVID-19 - but we also put forth some new associations," said Jehi. "Further validation and research are needed into these initial insights but these correlations are extremely intriguing."
In a previous study, researchers from Lerner Research Institute showed that 16 drugs – including melatonin, carvedilol, and paroxetine – and three drug combinations were identified as candidates for repurposing as potential COVID-19 treatments.
While these findings indicate an association between taking these medications and a reduced risk of testing positive for COVID-19, more research is needed to evaluate how these drugs may impact disease progression.
"The data suggest some interesting correlations but do not confer cause and effect," said Michael Kattan, Ph.D., Chair of Lerner Research Institute's Department of Quantitative Health Sciences.
"For example, our data do not prove that melatonin reduces your risk of testing positive for COVID-19. There may be something else about patients who take melatonin that is indeed responsible for their apparent reduced risk, and we don't know what that is. Consumers should not change anything about their behavior based on our findings."
The team developed the nomogram using data from patients tested for COVID-19 at Cleveland Clinic before April 2, 2020. The model showed good performance and reliability when used in a different geographic area and over time. This suggests that the predictors and patterns identified in the model are consistent across regions and communities, and could potentially be adopted for clinical practice in healthcare systems across the country.
The team expects that the model can help healthcare providers predict patient risk and tailor care delivery to specific individuals.
"This nomogram will bring precision medicine to the COVID-19 pandemic, helping to enable researchers and physicians to predict an individual's risk of testing positive," said Kattan.
"Additionally, while testing solutions continue to be needed, it is so important to make sure we are responsibly and optimally dispatching our resources ¬- including clinical personnel, personal protective equipment and hospital beds. Our risk prediction model stands to greatly assist hospital systems in this planning."