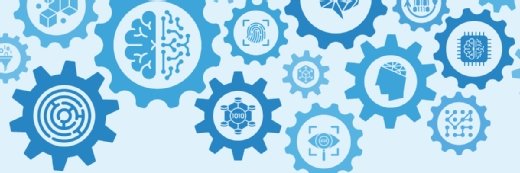
Getty Images
Deep Learning Identifies Best Treatments for Lung Cancer Patients
A deep learning technique could help providers choose the best possible treatment options for patients with lung cancer.
Combining a deep learning model with medical imaging techniques could help determine the best treatment options for patients with non-small cell lung cancer, according to a study published in Nature Communications.
Non-small cell lung cancer is the most common subtype of lung cancer and the leading cause of cancer-related deaths around the world, the researchers noted. Two major treatment strategies have emerged for patients with non-small cell lung cancer: tyrosine kinase inhibitors and immune checkpoint inhibitors.
However, choosing between these two treatment options is a challenging task. Biomarkers can change during therapy, making that treatment ineffective.
Researchers at the Moffitt Cancer Center set out to overcome this challenge. The team developed a noninvasive, accurate deep learning method to help providers determine the best course of treatment for these patients.
The model uses PET/CT imaging with the radiotracer 18F-Fluorodeoxyglucose, a type of sugar molecule. Imaging with 18F-FDG PET/CT can identify sites of abnormal glucose metabolism and help accurately characterize tumors.
"This type of imaging, 18F-FDG PET/CT, is widely used in determining the staging of patients with non-small cell lung cancer. The glucose radiotracer used is also known to be affected by EGFR activation and inflammation," said Matthew Schabath, PhD, associate member of the Cancer Epidemiology Department.
"EGFR, or epidermal growth factor receptor, is a common mutation found in non-small cell lung cancer patients. EGFR mutation status can be a predictor for treatment, as patients with an active EGFR mutation have better response to tyrosine kinase inhibitor treatment."
The team developed the deep learning model using retrospective data from non-small cell lung cancer patients at two institutions. The model classifies EGFR mutation status by generating an EGFR deep learning score for each patient. Once the model was built, researchers validated it with additional data from two more institutions.
The results showed that the deep learning model was able to predict EGFR mutation status with AUCs of 0.86, 0.83, and 0.81 in the training, validation, and independent test cohorts. While other studies have developed innovative techniques to improve treatment decisions for lung cancer patients, researchers noted that their approach is unique in its degree of accuracy.
"Prior studies have utilized radiomics as a noninvasive approach to predict EGFR mutation," said Wei Mu, PhD, study first author and postdoctoral fellow in the Cancer Physiology Department. "However, compared to other studies, our analysis yielded among the highest accuracy to predict EGFR and had many advantages, including training, validating and testing the deep learning score with multiple cohorts from four institutions, which increased its generalizability."
The study’s findings show the ability for deep learning technology to serve as reliable clinical decision support for oncologists treating non-small cell lung cancer.
"We found that the EGFR deep learning score was positively associated with longer progression free survival in patients treated with tyrosine kinase inhibitors, and negatively associated with durable clinical benefit and longer progression free survival in patients being treated with immune checkpoint inhibitor immunotherapy," said Robert Gillies, PhD, chair of the Cancer Physiology Department.
"We would like to perform further studies but believe this model could serve as a clinical decision support tool for different treatments."
Analytics techniques are increasingly playing a larger role in cancer diagnostics. A team from the University of California, Irvine, recently created a biochip to differentiate between cancers and healthy tissues at the single-cell level.
The study demonstrated the potential for AI tools to accurately classify multiple cell types.
“The World Health Organization says that nearly 60 percent of deaths from breast cancer happen because of a lack of early detection programs in countries with meager resources,” said senior author Rahim Esfandyarpour, UCI assistant professor of electrical engineering & computer science as well as biomedical engineering.
“Our work has potential applications in single-cell studies, in tumor heterogeneity studies and, perhaps, in point-of-care cancer diagnostics – especially in developing nations where cost, constrained infrastructure and limited access to medical technologies are of the utmost importance.”