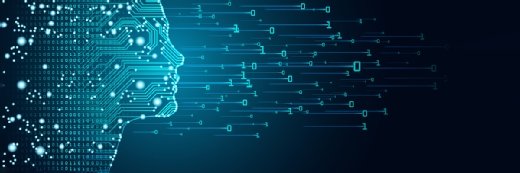
Getty Images
How Big Data Analytics Models Can Impact Healthcare Decision-Making
Big data analytics models can help policymakers make more informed healthcare decisions, contributing to better public and population health.
In healthcare, providers and lawmakers are faced with the task of making the best possible decisions for patients and the industry as a whole. From choosing the best treatments, to determining the most effective ways to utilize resources, leaders are making decisions every day that can impact health outcomes and costs.
With all of this information to consider, it’s no wonder that big data analytics tools have played an increasingly significant role in healthcare decision-making.
Researchers, providers, and policymakers are turning to big data analytics models to help improve care delivery, allocation of resources, and preventive health measures.
As the industry continues to innovate and refine these tools, data-driven decisions will soon become standard, leading to more proactive, successful healthcare operations.
Providing guidelines for navigating a healthcare crisis
The current COVID-19 pandemic is perhaps the most notable example of organizations leveraging big data analytics models to inform decision-making.
In the months since the virus has entered and spread throughout the US, entities from all sectors of the healthcare industry have moved quickly to leverage their big data assets and better understand how to respond to COVID-19.
Recently, researchers from the University of Washington received a $33,000 grant to develop a model that uses local data to generate policy recommendations that could reduce the spread of COVID-19 in King County. The model will help decision-makers answer important questions related to coronavirus, including when and how to reopen businesses and schools, and how to distribute a vaccine when one becomes available.
"We will be simulating the impact of various interventions — including social distancing measures, school closure policies, testing capacity, contact-tracing strategies and mask wearing — on population health outcomes," said lead researcher Shan Liu, a UW associate professor of industrial and systems engineering.
"Once a vaccine becomes available, we plan to expand the model to simulate vaccination rollout and coverage, and optimize for the best delivery configuration, such as vaccination priority if supply is limited."
Researchers have also utilized big data analytics tools to forecast possible constraints on hospital capacity and resources. A team from Cedars-Sinai recently developed a machine learning model that can predict data points related to the COVID-19 pandemic and predict staffing needs.
The platform tracks local hospitalization volumes and the rate of confirmed COVID-19 cases, running multiple forecasting models to help anticipate and prepare for increasing COVID-19 patient volumes with 85 to 95 percent degree of accuracy.
“Our goal is to have the capacity and the right care available every day to treat the patients who need us, which fluctuates on a daily basis,” said Michael Thompson, executive director of Enterprise Data Intelligence at Cedars-Sinai, which developed the platform and runs the forecasts. “We need to match that daily demand with the necessary resources: beds, staff, PPE and other supplies.”
Enabling advanced chronic disease prevention
In addition to monitoring and preparing for COVID-19 surges, healthcare researchers have increasingly turned to big data analytics tools to improve chronic disease detection and treatment.
In developing these models, investigators seek to evaluate specific clinical and non-clinical factors that may contribute to a person’s risk of developing a chronic disease.
At the College of Information Sciences and Technology at Penn State, researchers have developed an artificial intelligence algorithm to predict susceptibility to substance use disorder among young homeless individuals. The model could help inform officials about personalized rehabilitation programs for highly susceptible young people.
“To assist policymakers in devising effective programs and policies in a principled manner, it would be beneficial to develop AI and machine learning solutions which can automatically uncover a comprehensive set of factors associated with substance use disorder among homeless youth,” said Maryam Tabar, a doctoral student in informatics and lead author on the project paper.
The group identified environmental, psychological and behavioral factors associated with substance abuse among 1,400 homeless youth, including criminal history, victimization experiences, and mental health characteristics.
“By looking at what the model has learned, we can effectively find out factors which may play a correlational role with people suffering from substance abuse disorder,” said Amulya Yadav, assistant professor of information sciences and technology and principal investigator on the project.
“And once we know these factors, we are much more accurately able to predict whether somebody suffers from substance use. So if a policy planner or interventionist were to develop programs that aim to reduce the prevalence of substance abuse disorder, this could provide useful guidelines.”
Researchers are using big data analytics tools to better prevent other chronic diseases as well. In March 2020, a group from Mount Sinai created new predictive analytics tools and identified environmental factors that could lead to a new understanding of what triggers Crohn’s disease.
The project offered new ways to identify individuals with a high risk of developing the disease, and could result in more effective prevention efforts.
“Early identification of individuals at high risk for disease development could allow for close monitoring and interventions to delay, attenuate, or even halt disease initiation,” said Jean-Frederic Colombel, MD, Professor of Medicine (Gastroenterology) at the Icahn School of Medicine at Mount Sinai and Co-Director of Mount Sinai’s Susan and Leonard Feinstein Inflammatory Bowel Disease Clinical Center.
“This is highly relevant as we seek to predict and prevent IBD, which continues to sharply increase in numbers across the globe. In the absence of a cure, our clinical strategy will center on aggressive and innovative mechanisms to predict and prevent the disease.”
Identifying care disparities for improved population health
Big data analytics tools also have the potential to uncover disparities in healthcare. Last July, the Boston University School of Public Health announced a five-year partnership with Sharecare to mine social determinants of health data and enhance patient outcomes across the country.
The organizations aimed to build a Community Well-Being Index (CWI) using health data from Sharecare and social determinants data from SPH. The SPH Biostatistics and Epidemiology Data Analytics Center (BEDAC) will mine the information and help researchers generate actionable conclusions.
With CWI, investigators will have the ability to make connections between non-clinical and clinical factors, allowing them to identify and improve possible disparities in care.
“We are able to look at their data and integrate it with our data on social determinants of health,” said BEDAC executive director Kimberly Ann Dukes, an SPH research associate professor of biostatistics. “You are more a product of the community where you live, work, and play than you think you are.”
The University of Virginia (UVA) Health System is also leveraging data analytics models to reduce health disparities. The organization recently implemented a real-time artificial intelligence platform that integrates directly with EHRs and extracts social determinants and clinical risk factors from unstructured notes for more timely interventions.
“A lot of my work is around health equity and identifying potential disparities in testing access – individuals who may have met testing criteria based on their symptoms, but maybe didn’t receive testing,” Amy Salerno, MD, MHS, Director of Community Health and Well-Being at the University of Virginia (UVA) Health System, told HealthITAnalytics.
“We’ve been able to look for care access disparities and outcomes disparities in the ICU, admissions, and mortalities. And that allows us to think about changing our processes and look at the data to see if these changes improved our disparities.”
With the industry working to push through the COVID-19 pandemic and on to a brighter future, big data analytics tools will surely feature largely in day-to-day decision-making. These models will likely have a significant impact on all care decisions going forward.