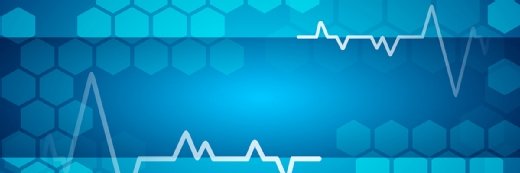
Ace2020/istock via Getty Images
Machine Learning Network Determines Severity of Lung Cancer
A machine learning tool analyzed CT scans to offer information about lung cancer severity and guide treatment options.
Researchers at Stanford University have developed a machine learning tool that can improve the interpretation of CT lung cancer images and determine the severity of the disease.
Lung cancer is the most common fatal malignancy in adults worldwide. CT imaging is a critical diagnostic tool to measure the location, extent, size, and shape of lung lesions to guide therapeutic decisions for patients with lung cancer. However, analysis of CT images is limited to what is visible to the human eye, which can result in differences in clinical care across different oncology centers.
“Quantitative image analysis has demonstrated that radiological images, such as CT scans of patients with lung cancer, contain more minable information than what is observed by radiologists,” said Olivier Gevaert, assistant professor of Medicine in Biomedical Informatics Research at Stanford.
“Using CT image datasets from several different oncology clinics, we set out to determine whether our neural network could be trained to accurately and reproducibly analyze the scans and deliver consistent, useful clinical information.”
To get consistent, accurate, and fast information from lung CT scans, a multidisciplinary group of experts created a machine learning neural network called LungNet. The team worked with scans from adults with non-small cell lung cancer (NSCLC), which accounts for 85 percent of lung cancer diagnoses.
The team trained and evaluated the algorithm on four independent cohorts of patients with NSCLC from four medical centers, with each center averaging several hundred patients. The LungNet analysis accurately predicted overall survival in all four patient groups, as well as classified benign versus malignant nodules. The tool was also able to further stratify nodules regarding cancer progression.
“LungNet demonstrates the benefits of designing and training machine learning tools directly on medical images from patients,” said Qi Duan, PhD, director of the NIBIB Program in Image Processing, Visual Perception and Display. “This is an outstanding example of how machine learning technology can be a cost-effective approach to advance disease detection, diagnosis, and treatment.”
Researchers expect that this tool will be valuable for classifying benign versus malignant tumors, and for stratifying patients into low, medium, and high-risk groups. This will enable intensified treatment for patients in the high-risk group and reduce unnecessary treatment for patients in the low-risk group.
As machine learning and AI continue to evolve and become embedded in the healthcare industry, more research teams are seeking to use these technologies to improve image analysis.
Recently, a team from Mount Sinai used artificial intelligence to quickly detect COVID-19 based on CT scans of the chest and patients’ clinical data. Because CT scans alone have limited predictive value, there is a significant need to incorporate clinical data into the diagnosis process – an ideal use case for artificial intelligence.
“AI has huge potential for analyzing large amounts of data quickly, an attribute that can have a big impact in a situation such as a pandemic,” said Zahi Fayad, PhD, Director of the BioMedical Engineering and Imaging Institute (BMEII) at the Icahn School of Medicine at Mount Sinai.
“At Mount Sinai, we recognized this early and were able to mobilize the expertise of our faculty and our international collaborations to work on implementing a novel AI model using CT data from coronavirus patients in Chinese medical centers.”
The algorithm was able to improve the detection of COVID-19-positive patients who had negative CT scans, demonstrating the potential for AI to advance medical imaging analysis.
“We were able to show that the AI model was as accurate as an experienced radiologist in diagnosing the disease, and even better in some cases where there was no clear sign of lung disease on CT,” said Fayad, who is also a Professor of Diagnostic, Molecular and Interventional Radiology at the Icahn School of Medicine at Mount Sinai.
“We're now working on how to use this at home and share our findings with others—this toolkit can easily be deployed worldwide to other hospitals, either online or integrated into their own systems.”