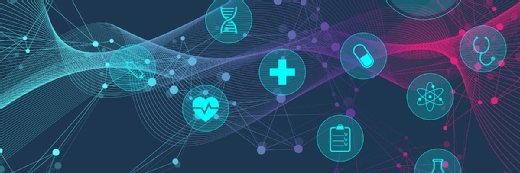
berya113/istock via Getty Images
Framework to help detect healthcare AI hallucinations
New research demonstrates the potential of an approach to address faithfulness hallucinations in artificial intelligence-generated medical summaries.
Researchers from the University of Massachusetts Amherst and healthcare AI company Mendel have published a framework for hallucination detection in AI-generated medical summaries.
As AI continues to generate hype in the healthcare industry, stakeholders are pursuing ways to improve the accuracy, safety and efficiency of these tools.
Technologies like generative AI -- including large language models (LLMs) -- have shown promise in streamlining nursing documentation and generating medical summaries. Proponents of AI integration in healthcare assert that such use cases highlight the tools' potential to reduce administrative burdens on clinicians, but others hold that additional steps must be taken to ensure the reliability and safety of AI prior to deployment.
One of the major hurdles to AI adoption in healthcare is the phenomenon of AI hallucination, which occurs when a model generates false or misleading information. LLMs are particularly susceptible to hallucination, which creates significant risks for their use in high-stakes clinical settings.
To mitigate these risks, the research team set out to develop a hallucination detection framework that could be applied to LLMs tasked with generating medical summaries.
To test the framework's ability to systematically identify and categorize hallucinations, the researchers applied it to a group of 100 medical summaries generated by GPT-4o and Llama 3.
The resulting analysis revealed that hallucinations were present in responses from both models across five categories of medical event inconsistency: patient information, patient history, diagnosis and procedures, medicine-related instructions and follow-up. Further, hallucinations related to chronological inconsistency and incorrect reasoning were also reported.
In general, GPT-4o generated longer summaries, averaging over 500 words, and made two-step reasoning statements. These longer summaries, which made extensive inferences, led to 21 medical event inconsistencies, 44 cases of incorrect reasoning and two chronological inconsistencies.
Llama-3's summaries were shorter, with less inferences, but this led to a drop in quality when compared to GPT-4o's responses. Overall, Llama-3 produced responses that contained 18 medical event inconsistencies, 26 cases of incorrect reasoning and one chronological inconsistency.
"Our findings highlight the critical risks posed by hallucinations in AI-generated medical summaries," said Andrew McCallum, Ph.D., professor of computer science at the University of Massachusetts Amherst, in a press release. "Ensuring the accuracy of these models is paramount to preventing potential misdiagnoses and inappropriate treatments in healthcare."
In addition to these findings, the research team also investigated the capacity of Mendel's Hypercube system to automate the annotation of hallucinations, as human hallucination annotation is a time-consuming and expensive process.
The tool utilizes medical knowledge bases, natural language processing and symbolic reasoning to represent patient documents. In doing so, Hypercube is designed to address overlapping and potentially conflicting information in EHRs, consolidating them into sets of properties and events.
The study found that by consolidating information in this way, the tool showed promise in improving the initial hallucination detection step prior to human expert review.
The team indicated that future research in this area should work to improve automatic hallucination detection systems to reduce the costs of human annotation and mitigate faithfulness hallucinations in healthcare AI models.
Shania Kennedy has been covering news related to health IT and analytics since 2022.