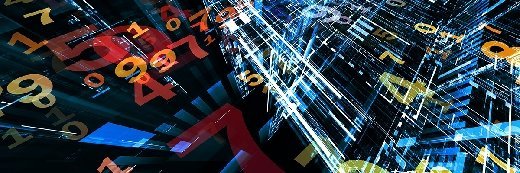
agsandrew - Fotolia
Cloud cost implications of the 5 V's of big data
Moving analytics to the cloud opens a lot of doors for users, but only if they keep costs in check and understand the 5 V's of big data -- volume, variety, velocity, veracity and value.
Enterprises increasingly use the cloud for big data analytics. These platforms provide scalable alternatives that can save money compared to on-premises systems, but only if they're used properly.
Cost controls can be a problem for companies of all sizes and experience levels, even those well versed in cloud computing. That's why it's essential for users to understand Cloud analytics and the five V's of big data: volume, variety, velocity, veracity and value. From there, they must learn to spend wisely to maximize the ROI.
The 5 V's and cloud analytics
Taking data and analytics to the cloud gives the user new options for handling analytics if it fits within the five V's of big data:
Volume
As the name implies, big data is all about the enormous size. The cloud provides virtually limitless storage capacity, which is why it's becoming an attractive option for businesses and government agencies with the ever growing data volumes.
Moving data and analytics to the cloud plays well in managing volume since it gives users the flexibility and scalability to meet peak demands. However, enterprises should still use discretion and shouldn't become data pack rats when it comes to cloud storage. Costs can add up quickly if users don't utilize lower-cost storage tiers when possible, or if they put too much unnecessary data in the cloud.
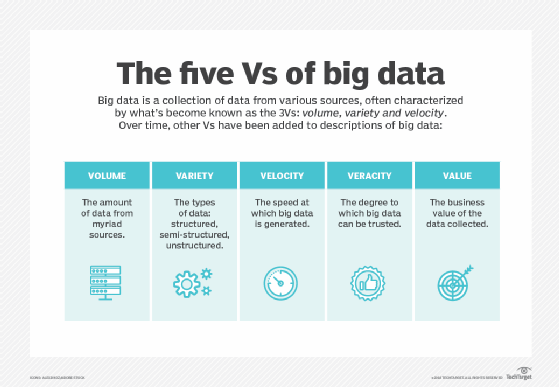
Variety
Variety, as a data science term, refers to heterogeneous sources, such as structured, semi-structured and unstructured data.
For example, an IT department might need to analyze semi-structured data from its back office and SaaS applications, while the accounting department wants to analyze structured data in the form of reports. Meanwhile, marketing wants to analyze pictures, videos, SMS texts and other data that doesn't fit into traditional database rows and columns.
Enterprises can use cloud-based data lakes to accommodate all of those analysis types and more.
Velocity
Velocity, in relation to big data in the cloud, is the high-speed accumulation of information that comes from SaaS apps, cloud platforms, IoT devices, social networks and any other data points relevant to a business. Velocity becomes more complicated as organizations attempt to add sufficient processing power to handle the massive and continuous flow of data being generated.
Cloud platforms can scale to meet the need for actionable data as an organization's systems grow.
Veracity
There will always be inconsistencies and uncertainties in an organization's data, regardless of where that data resides. But, the cloud can give users more room to get messy and further compromise the quality and accuracy of information.
A move to cloud analytics shouldn't come without a review and potential overhaul of internal data preparation, governance and management processes.
Value
There's little to no value to the bulk of the data an organization collects, unless the IT team can turn it into something actionable and provide the business with an edge.
With self-service, cloud-based analytics, in-house data scientists can focus on more strategic projects, while business users get the dashboards, reporting and a UI needed to interact with data themselves.
Cost implications of cloud storage
Of course, all that business value can quickly be negated if organizations don't control costs. But that can be difficult to get ahead of since cloud cost optimization for storage and analytics doesn't align with traditional cloud cost optimization exercises. Cloud analytics and consumption models can be unpredictable, and users often lack a frame of reference for the resources they'll need. Also, cloud management tools remain a work in progress in terms of their ability to govern analytics.
There are two main pricing models from cloud providers available to end users -- an ingestion model and a pay-per-use model. The ingestion model calculates the amount of data being stored in the service. Examples include Azure Stream Analytics and Google BigQuery.
With pay-per-use services such as Azure Data Lake Analytics and Amazon Kinesis Data Analytics, providers charge an hourly rate based on the average number of processing units an application needs to run the stream processing. It should be noted, capacity requirements can rise depending on the complexity of the queries being run.
Specific details about the models -- such as potential pricing discounts for active and long-term usage -- vary depending on the chosen cloud provider. A cost versus performance tradeoff with storage to analytics should be expected.
Take Amazon S3, for example. It's massively scalable and ideal for data lakes. However, you must put up with slower access as you move data to higher performing -- and more expensive -- storage, such as Amazon Elastic Block Store.
IT teams should review and follow their provider's documentation to estimate the economics of an analytics job. Then, create a financial model to forecast usage and prevent surprises.
They should also know their spending history and cost management practices prior to moving analytics to the cloud. The more users know about their historical and baseline data, the better they can track overspending.
And while self-service analytics is a draw for organizations, it can lead to even more billing surprises if users over indulge and restrictions aren't in place. Cloud-native and third-party tools can be used to monitor workloads as they're rolled out.
The future of big data in the cloud
The recent Salesforce acquisition of Tableau and Google's acquisition of Looker point to an exciting future for analytics in the cloud. SaaS vendors -- such as Salesforce -- have the infrastructure, subscription-based pricing expertise and marketing and sales channels to introduce cloud analytics into new and existing accounts. The major cloud providers will bring their technology, IP and expertise in-house to compete against their new SaaS analytics competitors.
In addition, the two main pricing models previously mentioned are ripe for change. Google already offers the choice between pay-per-use and flat rates, while third-party vendors like SAP offer more traditional subscription models. The major public cloud providers may get more aggressive with their pricing and offer more alternatives beyond existing efforts with channel partners and discount programs.