How to carefully plan a database migration to the cloud
Plenty of organizations decide to migrate databases to the cloud. Is it the right move? Consider application type, provider lock-in and the appeal of cloud-specific features.
Enterprises continue to march their applications and workloads into the cloud, but, for many, a database migration to the cloud feels like a bridge too far.
Gartner predicts 75% of databases will run on cloud database platforms by 2023. Most of this growth in database migration to the cloud will come from apps used by small to medium-sized businesses, which tend to be more nimble than large organizations. Enterprise adoption, however, will be a bit slower, particularly for transaction-processing apps with numerous integrations to other enterprise apps, said Gartner analyst Donald Feinberg.
"Most larger organizations have huge applications and a huge amount of data. So, even if they commit 100% to the cloud, it would take them five or more years to completely migrate," Feinberg said.
Organizations must decide whether to lift and shift existing workloads to the cloud, such as simply hosting their database on an AWS EC2 instance, or offload some infrastructure maintenance by moving it to a managed database, such as Azure SQL Database. A lift-and-shift migration is often a steppingstone; once data is in the cloud it's easier to rearchitect applications later. In theory, this involves only copying existing databases to similar databases that run on IaaS offerings. For smaller organizations, this process is straightforward.
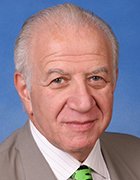
For a large enterprise, however, the conversion from its existing practice to cloud services is more complicated. "When you go to the cloud, all of your internal procedures change, which requires new training," Feinberg said.
A large organization ready to proceed with a database migration to the cloud might also struggle to integrate applications to run across hybrid infrastructure during the transition.
Distributed apps ease cloud lock-in fears
Businesses that don't want to get locked into a cloud platform may balk at a database migration, but newer distributed architectures help to ease those worries. Modern distributed applications, based on a set of microservices, often run on top of a Kubernetes cluster that can be hosted in a corporate data center or on AWS, Google Cloud, Microsoft Azure or any other public cloud.
"This new breed of application no longer relies on permanently mapped block storage and traditional SQL databases, but it requires a centralized backbone for all of these loosely coupled and often geographically distributed microservices," said Torsten Volk, managing research director at Enterprise Management Associates.
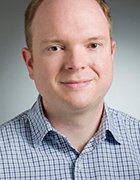
A cloud database provides this backbone -- but doesn't require a business to rip and replace its current database infrastructure. Microservices-based apps can look up and save their state, exchange data, authorize users, access logs, subscribe to content feeds, map interdependencies, look up or provide operations data or simply share a bunch of binaries.
In addition, cloud-based database offerings can absorb longer and difficult-to-predict latencies of application requests that originate from different geographical locations, and minimize the risk of diminished data integrity. "All this goes way beyond the job description of your average [database] admin," Volk said.
Cloud databases also offer the latest capabilities that application developers demand, such as publish/subscribe, asynchronous messaging, data streaming, NoSQL, key value stores and graph database functions.
Ultimately, the benefits of any managed cloud database come at the cost of tighter coupling between applications and cloud provider. "You want to make sure you at the very least understand the degree of lock-in you are creating," Volk said.
Four keys to plan a cloud database migration
Any cloud migration requires a lot of planning, but there are even more key decisions to be made in a database migration to the cloud. Carl Olofson, an analyst at IDC, identified four key choices.
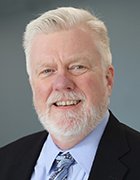
Choose your DBMS. First and foremost, an organization must decide what to do with the database management system (DBMS). Should you move to the cloud version of the same DBMS currently in use? That would allow the staff to continue to use its existing skillset. Instead, it may be more economical to go with a database service native to the chosen cloud, such as Aurora on AWS, Cloud Spanner on GCP or Azure SQL Database. You could also look for a third-party cloud database offering.
Determine your support preference. Native cloud databases come with a full range of support tools and utilities. They simplify troubleshooting, since there is just one support organization to call whether a problem arises from the database or the infrastructure. However, this could also create problems if different parts of the organization use different cloud platforms.
Rethink apps and analytics. A business that's ready to move to the cloud should take the opportunity to rethink how its applications work, Olofson said. Consider additional analytic features, such as those that support AI and machine learning, timeseries and graph analysis.
Be open to change. Those features also might make you rethink your database structure itself. "Instead of continuing with the same sort of database system currently in use, one may want to look at some that provide better benefit for some of these advanced capabilities," Olofson said. Instead of a lift-and-shift database migration, an organization could adopt a cloud database service such as Amazon Aurora or Azure Cosmos DB -- or climb even further up the chain with vendors' serverless services.