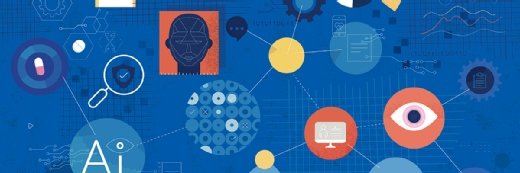
DrAfter123/DigitalVision Vectors
MRI-based machine learning approach predicts breast cancer metastasis
A convolutional neural network successfully flagged 95% of breast cancer patients with axillary metastasis, demonstrating potential to reduce unnecessary biopsies.
A magnetic resonance imaging (MRI)-based machine learning approach developed by researchers at UT Southwestern Medical Center could improve the detection of breast cancer metastasis and reduce the need for biopsies.
The tool, a four-dimensional convolutional neural network, is designed to incorporate dynamic contrast-enhanced (DCE) breast images with clinical data to detect axillary metastasis – a phenomenon in which cancer cells are found in the lymph nodes under the arms.
“Most breast cancer deaths are due to metastatic disease, and the first site is usually an axillary lymph node,” said study lead Basak Dogan, MD, Professor of Radiology, Director of Breast Imaging Research and member of the Harold C. Simmons Comprehensive Cancer Center at UT Southwestern, in the news release. “Determining nodal status is critical in guiding treatment decisions, but traditional imaging techniques alone do not have enough sensitivity to rule out axillary metastasis. That often requires patients to undergo invasive procedures that involve radioisotope and dye injection followed by surgery to remove and test whether the axillary nodes harbor cancer cells.”
The analysis relied on data and medical images from 350 newly diagnosed breast cancer patients with known nodal status at UT Southwestern and the Moody Center for Breast Health. When fed this information and tasked with identifying axillary metastasis, the tool achieved an area under the receiver operating characteristic curve (AUC) of 0.87, a sensitivity of 89% and a specificity of 76%.
The model performed similarly on two independent datasets: one from a safety-net hospital and one from a university hospital. When trained on data from the safety-net hospital, the tool reached an AUC of 0.84, but performance dipped slightly to 0.81 AUC when the model was trained on university hospital data.
Further, the tool accurately identified 95% of patients with axillary metastasis while demonstrating its potential to help avoid unnecessary surgical sentinel node biopsies.
“That’s an important advancement because surgical biopsies have side effects and risks, despite having a low probability of a positive result confirming the presence of cancer cells,” Dogan explained. “Improving our ability to rule out axillary metastasis during a routine MRI – using this model – can reduce that risk while enhancing clinical outcomes.”
“Patients with benign findings from traditional MRI exams or needle biopsies are often subjected to sentinel lymph node biopsy because those tests can miss a significant proportion of metastasis,” she continued. “Our research demonstrates that it’s possible to identify – with a high degree of accuracy – patients who are nonmetastatic, which benefits the patient and also allows the physician to tailor treatment.”
The study builds on previous UT Southwestern research aimed at developing predictive tools for breast cancer metastases.
“The development and validation of AI models for medical imaging holds great promise in helping us in the fight against breast and other cancers, and this new tool is a significant step forward,” Dogan stated.
This work is the latest to apply AI in an effort to improve cancer care.
In March, researchers from Washington University School of Medicine in St. Louis developed a deep learning tool to predict which non-small cell lung cancer (NSCLC) patients were likely to experience brain metastasis.
Once the cancer reaches the brain, aggressive treatments like immunotherapy, chemotherapy, radiation therapy and targeted drug therapy are required, but methods to identify at-risk patients remain elusive.
To bridge this gap, the research team built a deep learning tool to predict brain metastasis risk using histopathology images of the lung. The tool makes its predictions by flagging abnormal features within a biopsy image that a pathologist might miss, and it was shown to outperform clinicians.