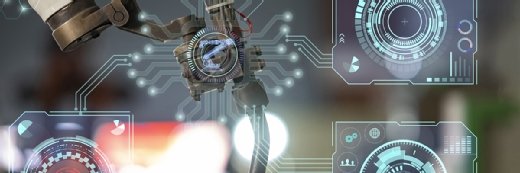
Getty Images/
NLP Framework Could Improve Medical Summarization Tools
Researchers have developed a framework to fine-tune the natural language processing models used in medical text summarization tools.
Researchers from Pennsylvania State University (PSU) have developed a natural language processing (NLP) framework to improve the efficiency and reliability of artificial intelligence (AI)-driven medical text summarization tools.
The medical summarization process is key in helping to condense patient information into accessible summaries, which can be used in electronic health records, insurance claims, and at the point of care. AI can be used to generate these summaries, but the research team underscored that doing so can lead to concerns about the reliability of said summaries.
“There is a faithfulness issue with the current NLP tools and machine learning algorithms used in medical summarization,” explained first author of the study Nan Zhang, a graduate student in the PSU College of Information Sciences and Technology (IST), in the news release. “To ensure records of doctor-patient interactions are reliable, a medical summarization model should remain 100% consistent with the reports and conversations they are documenting.”
Current medical summarization models utilize human supervision to prevent the tools from creating ‘unfaithful’ summaries that could lead to patient harm, but the researchers noted that studying the sources of unfaithfulness in these models is crucial to ensuring efficiency and safety.
To investigate model unfaithfulness, the researchers analyzed three datasets generated by existing tools in the realms of radiology report summarization, medical dialogue summarization, and online health question summarization.
Between 100 and 200 summaries were randomly selected from each dataset and manually compared to the original medical reports from which they were derived. Summaries were then categorized based on their faithfulness to the source text, with unfaithful summaries being sorted into error categories.
“There are various types of errors that can occur with models that generate text,” Zhang noted. “The model may miss a medical term or change it to something else. Summarization that is untrue or not consistent with source inputs can potentially cause harm to a patient.”
This analysis revealed that a significant portion of summaries contained errors of some kind. A portion of these summaries was found to be contradictory to the original medical reports, and a few presented signs of “hallucination,” a phenomenon in which the summaries contained additional information not supported by the medical reports used to generate them.
To address these issues, the research team developed the Faithfulness for Medical Summarization (FaMeSumm) framework.
The framework was designed using sets of contrastive summaries – medical summaries that were either ‘faithful’ and error-free, or ‘unfaithful’ and containing errors – and annotated medical terms to help improve existing medical text summarization tools.
Using the framework, the researchers fine-tuned pre-trained language models to help them address errors, rather than simply mimicking words contained in medical reports.
“Medical summarization models are trained to pay more attention to medical terms,” Zhang indicated. “But it’s important that those medical terms be summarized precisely as intended, which means including non-medical words like no, not or none. We don’t want the model to make modifications near or around those words, or the error is likely to be higher.”
The analysis further demonstrated that FaMeSumm can help effectively summarize information sourced from various training datasets, including those containing clinicians’ notes and complex questions from patients.
“Our method works on various kinds of datasets involving medical terms and for the mainstream, pre-trained language models we tested,” Zhang said. “It delivered a consistent improvement in faithfulness, which was confirmed by the medical doctors who checked our work.”
The research also highlighted the potential for fine-tuned large language models (LLMs) in healthcare.
“We did compare one of our fine-tuned models against GPT-3… We found that our model reached significantly better performance in terms of faithfulness and showed the strong capability of our method, which is promising for its use on LLMs,” stated Zhang.
“Maybe, in the near future, AI will be trained to generate medical summaries as templates,” he continued. “Doctors could simply doublecheck the output and make minor edits, which could significantly reduce the amount of time it takes to create the summaries.”
This study is one of many seeking to assess the potential of generative AI and LLMs in healthcare.
Last week, a research team from the New York Eye and Ear Infirmary of Mount Sinai (NYEE) described how OpenAI’s Generative Pre-Training–Model 4 (GPT-4) is capable of matching or outperforming ophthalmologists in glaucoma and retina management.
Ophthalmology is a high-utilization specialty, presenting a unique opportunity for AI-driven clinical decision support tools to help improve patient care. Glaucoma and retinal conditions often result in a high volume of complex patients, making streamlined case management a high priority for providers.
The researchers found that GPT-4 was proficient in handling both types of cases, often matching or exceeding the accuracy and completeness of case management suggestions offered by ophthalmologists.