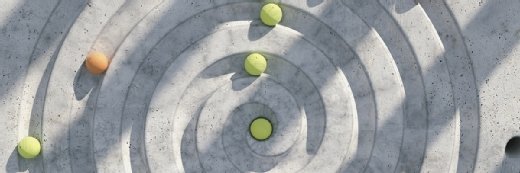
Getty Images/iStockphoto
Safeguards Needed for Generative AI EHR Data Summarization
A viewpoint published in JAMA notes that EHR data summarization LLMs could perpetuate bias, underscoring the need for FDA oversight.
While generative AI shows promise for EHR data summarization, using large language models (LLMs) to summarize clinical data brings risks that are not clearly covered by existing Food and Drug Administration (FDA) safeguards, according to a viewpoint published in JAMA.
A little over a year after ChatGPT’s public release, the healthcare industry is advancing use cases for generative AI and LLMs that summarize patient data.
“Current EHRs were built for documentation and billing and have inefficient information access and lengthy cut-and-pasted content,” Katherine E. Goodman, JD, PhD, assistant professor of epidemiology and public health at the University of Maryland School of Medicine, wrote in the article.
“This poor design contributes to physician burnout and clinical errors,” she noted. “If implemented well, LLM-generated summaries therefore offer impressive advantages and could eventually replace many point-and-click EHR interactions.”
However, Goodman pointed out the potential for patient harm because LLMs performing summarization are unlikely to fall under FDA medical device oversight.
“Indeed, FDA final guidance for clinical decision support software—published two months before ChatGPT’s release—provides an unintentional ‘roadmap’ for how LLMs could avoid FDA regulation,” she said.
Even LLMs performing summarization tasks would not clearly qualify as devices because they provide language-based outputs rather than predictions or numeric estimates of disease, Goodman acknowledged.
“Currently, there are no comprehensive standards for LLM-generated clinical summaries beyond the general recognition that summaries should be consistently accurate and concise,” she wrote.
However, there are many ways to summarize clinical data accurately. Differences in summary length, organization, and tone could all influence clinician interpretations and subsequent decision-making processes.
To illustrate these challenges, Goodman prompted ChatGPT-4 to summarize a small sample of deidentified clinical documents.
Running identical prompts on identical discharge documents, LLM summaries varied in patient conditions listed and in clinical history elements emphasized.
“These differences have important clinical implications because it is well documented that how information is organized and framed can change clinical decision-making,” Goodman said. “Evaluating the impact of varied summaries on patient care requires clinical studies.”
Additionally, even small differences between prompts can impact output. For instance, LLMs can exhibit “sycophancy” bias, she explained. Sycophancy occurs when LLMs tailor responses to perceived user expectations.
For instance, when prompted to summarize previous admissions for a hypothetical patient, summaries varied in clinically meaningful ways depending on whether there was concern for myocardial infarction or pneumonia.
What’s more, summaries that appear accurate could include small errors with clinical significance.
“These errors are less like full-blown hallucinations than mental glitches, but they could induce faulty decision-making when they complete a clinical narrative or mental heuristic,” Goodman wrote.
For example, a chest radiography report noted indications of chills and nonproductive cough, but the LLM summary added “fever.” Although the word “fever” is a one-word mistake, it could lead a provider to a pneumonia diagnosis when they might not have reached that conclusion otherwise, the article noted.
“Absent statutory changes from Congress, the FDA will not have clear legal authority to regulate most LLMs generating clinical summaries,” Goodman explained. “However, regulatory clarifications, coupled with robust voluntary actions, will go a long way toward protecting patients while preserving LLMs’ benefits.”
First, the industry needs comprehensive standards for LLM-generated summaries, with domains that go beyond accuracy and include stress testing for sycophancy and small but clinically important errors, Goodman said.
“These standards should reflect scientific and clinical consensus, with input beyond the few large technology companies developing healthcare LLMs,” she emphasized.
Second, LLMs performing clinical summarization should be clinically tested to quantify clinical harms and benefits before widespread availability.
“Third, the highest-risk—but likely most useful—summarization LLMs will permit more open-ended clinician prompting, and we encourage the FDA to clarify regulatory criteria preemptively,” Goodman wrote.
These clarifications should indicate that some prompts, such as “summarize my patient’s history relevant to the risk of heart failure,” cause LLMs to function as medical devices despite semantically restricting to summarization, she added.
“The FDA could offer these statements in new guidance or as updates to existing guidance to recognize that the world has changed meaningfully since the clinical decision support guidance’s original release in late 2022,” Goodman concluded.