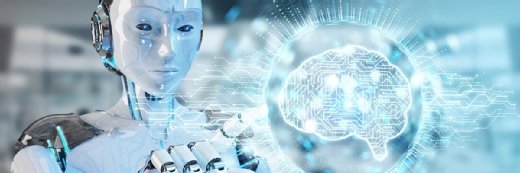
sdecoret - stock.adobe.com
5 use cases for generative AI in healthcare documentation
GenAI in healthcare offers promise for tasks such as clinical documentation, but clearly defined regulations and standards are needed to maximize benefits and minimize risks.
Healthcare documentation captures and preserves data generated by healthcare providers during patient care, including medical histories, clinical notes, medical images, diagnostic test results, treatment plans, prescriptions, appointments and billing information.
Studies have shown that healthcare professionals spend more time documenting patient information than interacting with patients -- a significant factor for clinician burnout. Using generative AI (GenAI) in a healthcare information management setting can shift this balance by automating electronic health record (EHR) data entry and generating drafts of medical documentation that clinicians can review and finalize.
Near-term adoption of GenAI in healthcare is focused on administrative use cases that improve the quality and efficiency of healthcare documentation, according to a survey published in February 2025 by the American Medical Association (AMA). GenAI also has the benefit of being measurable. For example, Microsoft reported its Dax Copilot augmented AI assistant saves clinicians an average of five minutes per patient encounter. Oracle has determined that its Oracle Health Clinical AI Agent reduces documentation time by nearly 30%.
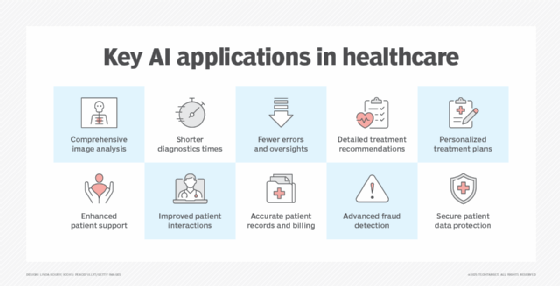
GenAI drives positive outcomes for patients and healthcare providers in the following five ways.
1. Streamline workflows
A quality improvement study published in the March 2024 issue of JAMA Network Open evaluated the adoption and usability of AI-generated draft replies to patient messages at an academic medical center. After five weeks, improvements in provider task load and emotional exhaustion scores suggested high expectations for the technology's ability to streamline clinical documentation workflows.
The authors proposed that using GenAI to create the first draft of healthcare documents can minimize after-hours charting. The technology can also be used for the following:
- Automate clinical note generation. Ambient AI medical scribe technology can transcribe patient encounters in real time and provide clinicians with draft notes for review.
- Customize clinical note templates. Clinicians can use GenAI to customize Simple Object Access Protocol (SOAP) and behavior, intervention, response and plan (BIRP) templates for specific medical specialties, patient populations and languages.
- Summarize telehealth interactions. GenAI can draft structured visit notes summarizing patient concerns and remote exam findings after a telemedicine appointment.
- Generate shift reports. In clinical settings, GenAI can help ensure continuity of care by generating drafts that summarize key patient information, such as vital signs and medication changes.
- Draft referral letters and lab work requests. When vendors integrate GenAI into EHRs, AI can extract relevant information and create drafts for personalized referral letters or lab work requests.
2. Improve data accuracy
GenAI models can process vast amounts of medical data from EHRs to identify missing information. The large language models (LLMs) that support GenAI can also be used for the following:
- Assist with data entry. GenAI can automatically populate EHR fields and help with record completeness by issuing prompts when required documentation is missing.
- Automate prior authorization and billing documentation. GenAI can extract relevant patient data from EHRs and clinical notes and use the information to pre-populate authorization and billing forms with patient data. An automated workflow lets clinicians quickly verify pre-filled data rather than manually entering all details.
- Validate insurance codes. GenAI can cross-reference patient records with medical codes and payer rules to reduce the risk of claim denials. This use case has the potential to minimize the manual data entry errors that often lead to claim rejections.
3. Optimize medical data
About 80% of healthcare data is unstructured data that doesn't fit neatly into traditional database fields, according to industry observers. GenAI has the potential to significantly enhance the efficiency and effectiveness of EHRs by transforming unstructured healthcare data into usable formats. LLMs trained on multimodal data sets can also be used for the following:
- Reformat data for compliance with specific standards. LLMs can be trained on the formats required by Health Level Seven International (HL7), Fast Healthcare Interoperability Resources (FHIR) and International Classification of Diseases, 10th Revision (ICD-10) to ensure all documentation meets regulatory standards.
- Standardize radiology, pathology and laboratory report formats. GenAI can normalize report structures from different labs and imaging centers. This can make it easier and faster for physicians to locate critical information, regardless of the original report's structure.
- Facilitate digital transformation. Some GenAI models can use optical character recognition to quickly and accurately extract text from scanned documents and create digital versions of paper files for human-in-the-loop review.
- Convert raw patient data into meaningful narratives. Multimodal LLMs can aggregate structured and unstructured data from multiple sources and summarize the information in a format that helps clinicians make informed decisions faster.
4. Reduce healthcare provider stress and burnout
Physician and clinician burnout is a growing crisis, and documentation burdens are a major contributor. GenAI has the potential to significantly reduce stress and burnout by enabling clinicians to do the following:
- Focus on patient care. By automating documentation tasks, clinicians can spend more time interacting with patients and less time on paperwork. This shift could increase job satisfaction and foster a renewed sense of purpose.
- Reduce after-hours work. Clinicians can use GenAI to complete their documentation during patient encounters and minimize the need to stay late or work from home to catch up on notes.
- Enhance decision-making. Clinicians can make data-driven decisions faster using GenAI to access relevant information in real time, rather than spending time manually searching through databases.
- Facilitate better communication. Communication among various healthcare providers can be improved by using GenAI to translate medical jargon and abbreviations into clear, understandable language.
5. Enhance patient engagement
Perhaps one of the most significant uses for GenAI in healthcare documentation is reducing clinician screen time during patient interactions. A 2024 study by the University of California's San Diego School of Medicine suggested that GenAI can improve patient engagement by helping healthcare professionals give patients their undivided attention, potentially leading to better health outcomes.
The technology can also be used for the following:
- Personalize care plans. Clinicians can use GenAI to analyze patient data and develop care plans tailored to individual health conditions, preferences and histories. A personalized approach can lead to better patient outcomes and satisfaction.
- Improve post-visit education. GenAI can explain complex medical concepts and give patients follow-up instructions in their native languages.
- Automate responses to patient messages in healthcare portals. Healthcare professionals can use GenAI to draft, review and edit responses to patient messages.
- Track progress in wellness or disease management programs. GenAI can draft reports summarizing patient progress, highlighting successes and identifying improvement areas.
- Improve access to information. AI-powered healthcare chatbots can offer patients general support outside of clinic hours by answering questions about office hours, office locations and payment options. Some chatbots allow patients to schedule, change or cancel appointments.
Best practices using GenAI in healthcare documentation
For GenAI to reach its full potential in healthcare documentation, clinicians and patients must understand that the technology's purpose is to augment human intelligence, not replace it. Human-in-the-loop oversight is essential, and AI-generated content should constantly be reviewed to ensure it's accurate and complete.
The following best practices can help healthcare professionals trust AI-assisted documentation:
- Encourage adoption of GenAI technology in small trials.
- Train clinicians and staff on how to review, edit and validate AI-generated medical content.
- Notify patients when AI is used for medical record-keeping.
- Require AI systems to encrypt patient data and de-identify protected health information (PHI).
- Maintain audit logs for all AI-generated documentation.
- Audit AI-generated clinical documentation regularly to assess its accuracy, completeness and fairness.
GenAI documentation challenges
While GenAI can potentially improve efficiency and reduce clinician burnout, the technology is still evolving. In addition, a lack of standards has created challenges in areas such as data privacy, regulatory compliance and liability.
Until there are standards or legislation for GenAI's use in healthcare documentation, frameworks like the World Health Organization's "Ethics & Governance of Artificial Intelligence for Health" report and the Coalition for Health AI (CHAI) Assurance Standards Guide can be valuable tools for ensuring that use cases and implementations comply with current regulations on PHI.
Healthcare providers can also develop a framework to address the specific challenges of their organization. Ideally, a robust AI governance framework for healthcare documentation should mitigate risks and maximize benefits by including the following:
- Guidelines for the responsible use of AI documentation.
- Security protocols to protect GenAI systems and data from cyberattacks and breaches.
- Procedures to ensure compliance with HIPAA, GDPR and other relevant regulations.
- Strategies to monitor and evaluate GenAI effectiveness.
- Guidance on how and when AI-generated documentation can be used with other IT systems.
- Instructions on how to report and correct AI mistakes.
Future of GenAI in healthcare documentation
GenAI promises to transform various aspects of AI medical documentation and mitigate clinician burnout. To be used effectively, leadership should focus on identifying practical use cases for GenAI tools and creating guidelines for using LLMs to draft various types of documentation.
As the LLMs that power GenAI become more multimodal, the technology is expected to become more adaptive and learn from each clinician's documentation style, specialty and workflow. However, stakeholders must work toward creating comprehensive standards and regulatory clarity to maximize the benefits of GenAI while minimizing risks.
Editor's note: This article was updated in April 2025 to include additional use cases, update survey data and improve the reader experience.
Margaret Rouse is a technical writer who has covered enterprise information technology and market trends for the past 20 years.
Hannah Nelson has been covering news related to health information technology and health data interoperability since 2020.