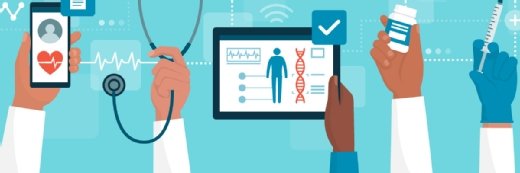
elenabs/istock via getty images
Models Classify Osteoarthritis Subgroups Based on Pain, Disease Severity
Researchers have developed algorithms to identify osteoarthritis patient subgroups that experience more comorbidities and lower quality of life than their peers.
In a recent study published in BMC Medical Research Methodology, researchers developed multiple algorithms capable of identifying important osteoarthritis (OA) patient subgroups, such as those experiencing moderate-to-severe disease or inadequate response to pain treatments, using EMR data.
The study authors indicated that currently, no algorithms to classify these subgroups exist, which may be due to a lack of relevant measures included in common data sources or the complexity of defining each subgroup’s characteristics.
OA is the most common form of arthritis, with the Centers for Disease Control and Prevention estimating that the condition impacts 32.5 million adults in the United States. The disease is degenerative, slowly breaking down joints over time, which can lead to pain, reduced function, and disability.
The study notes that these adverse effects are more likely to be experienced by those with moderate-to-severe OA and those whose current pain-related therapies are inadequate. These patients are disproportionately affected by higher rates of comorbidities, medication use, and dissatisfaction with their medications, which lead to lower quality of life.
Characterizing and understanding patients in these groups is key to addressing these issues, leading the research team to develop algorithms to flag these individuals.
To do so, the researchers pulled claims, EMR, and chart data from two integrated delivery networks. Chart data were leveraged to identify whether or not three relevant OA-related characteristics were present: OA of the hip and/or knee, moderate-to-severe disease, and inadequate/intolerable response to at least two pain-related medications.
Classifications based on these characteristics were then used as a benchmark for algorithm validation. Two sets of case-identification models were developed.
The first was a set of predefined algorithms based on a literature review and clinician input, while the second was a set of machine learning (ML) models using logistic regression, classification and regression tree, and random forest algorithms.
The patient classifications generated by these algorithms were then compared and validated against the original chart data.
The analysis included 571 adult patients. Of these, 519 had OA of hip and/or knee, 489 had moderate-to-severe OA, and 431 had inadequate response to at least two pain medications.
In terms of performance, the predefined algorithms demonstrated high positive predictive values (PPV) of at least 0.83 for identifying each of the three relevant OA characteristics. However, these models also had low negative predictive values between 0.16 and 0.54. The algorithms sometimes also had low sensitivity, with their sensitivity and specificity for identifying patients with all three characteristics reaching 0.95 and 0.26, respectively.
Conversely, the ML models achieved higher performance in identifying these patient subgroups, with sensitivity ranging from 0.77–0.86, specificity from 0.66–0.75, PPV from 0.88–0.92, and NPV from 0.47–0.62.
These findings led the researchers to conclude that while predefined algorithms adequately classified these OA subgroups, ML models were better able to differentiate patients in these groups. The study authors noted that the use of algorithms like these may enable real-world data to more effectively research this patient population and improve care.
Other researchers are also investigating how artificial intelligence (AI) could be used to tackle OA.
In 2020, a research team at the University of Pittsburgh School of Medicine and Carnegie Mellon University College of Engineering created an AI-based method to predict OA years before its onset.
The approach uses MRI scans to detect subtle signs of OA that aren’t visible to the naked eye, which the researchers noted may help providers in the future prevent the condition with drugs, rather than treat it with surgery.