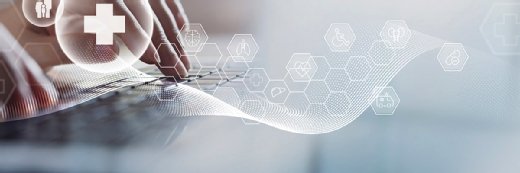
tippapatt - stock.adobe.com
Machine Learning, Natural Language Processing Addresses Bias
Researchers used machine learning and natural language processing to find bias in opioid misuse classifiers.
Rush University Medical Center researchers conducted a study using machine learning to determine bias within natural language processing (NLP) regarding opioid misuse classifiers.
Over the last 20 years, intelligent computing and machine learning have become important tools in patient-centered healthcare. Machine learning has demonstrated it can outperform clinical judgment at the population level by reducing screening burdens and accessing inequalities in chronic diseases and conditions.
With any data, analytics can contain bias, including sample, measurement, representative, and historical bias. Bias can impact machine learning in every step of model development, testing, and implementation, leading to algorithmic bias and feedback loops.
Bias can significantly impact population health and create disparities in disadvantaged groups.
“When natural language processing (NLP) classifiers are developed with biased or imbalanced datasets, disparities across subgroups may be codified, perpetuated, and exacerbated if biases are not assessed, identified, mitigated, or eliminated. In healthcare settings, these biases and disparities can create multiple layers of harm,” the researcher wrote in the study.
According to the researchers, United States medical institutions and pharmaceutical companies have significantly impacted the opioid overdoes epidemic. Between the 1990s and 2011, opioid prescriptions tripled and deaths due to pharmaceutical opioids more than tripled.
As opioid consumption shifted to pharmaceuticals and patients with pains, opioid misuse expanded from the criminalization and abstinence models that had primarily targeted urban Black and Brown men to a disease or addition model. However, studies of substance misuse screening programs and treatment services illustrate how medicine maintained racial bias and access inequalities.
With the structural history of how race impacts clinical data regarding substance misuse, researchers operationalized principles of fairness, accountability, transparency, and ethics (FATE) to examine NLP opioid misuse classifier’s predictions.
“The identification of bias and fairness in screening tools is critical to plan for mitigation or elimination prior to deployment. In this article, we first apply techniques to audit our classifier’s fairness and bias by adapting a bias toolkit and then attempt to correct bias with post-hoc methods,” the researchers explained.
The researchers then examined face validity by running Local Interpretable Model-Agnostic Explanations (LIME) across all predictions using average features to look for differences in features between race/ethnicity groups.
Two experiments were directed using electronic health records data. Bias was then examined through testing for differences in type II error rates across racial/ethnic subgroups (Black, Hispanic/Latinx, White, Other) using bootstrapped 95 percent confidence intervals.
The researchers identified bias in the false-negative rate (FNR = 0.32) of the Black subgroup compared to the FNR (0.17) of the White subgroup. The top features were “heroin” and “substance abuse” across subgroups.
“Post-hoc recalibrations eliminated bias in FNR with minimal changes in other subgroup error metrics. The Black FNR subgroup had higher risk scores for readmission and mortality than the White FNR subgroup, and a higher mortality risk score than the Black true positive subgroup (P < .05),” the researchers wrote.
Researchers found that the Black FNR subgroup had the highest severity of disease and risk for poor outcomes. Similar features were also reflected between subgroups for predicting opioid misuse; however, inequalities were present.
Post-hoc mitigation techniques mitigated bias in type II error rates without producing substantial type I error rates. Bias and data disadvantages should be systematically addressed throughout the process. The researchers concluded that standardized and transparent bias assessments are necessary to improve trust in clinical machine learning models.