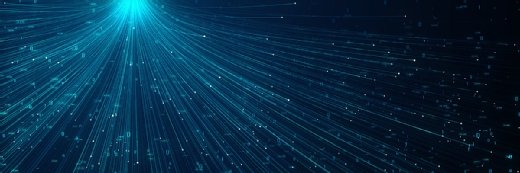
your123 - stock.adobe.com
GenAI displays no racial, sex bias in opioid treatment plans
Large language models demonstrated no race- or sex-based differences in opioid treatment recommendations, which could help reduce bias and improve health equity.
Mass General Brigham researchers writing recently in PAIN found that large language models, or LLMs, do not display race- or sex-based biases when making opioid treatment recommendations.
The research team emphasized that while biases pervade much of healthcare, health equity considerations are particularly important in the realm of pain management. The researchers noted that clinicians are more likely to underestimate and undertreat pain reported by Black patients, while white patients were significantly more likely to receive opioids for their pain than patients in other racial and ethnic groups.
These biases in opioid prescription exacerbate existing inequities in healthcare, and there is some concern that AI tools could worsen them -- despite the hype around emerging technology like generative AI.
To assess how these tools could help counter or compound biases in pain management, the research team set out to determine how LLM recommendations vary based on patients' race, ethnicity and sex.
The study sourced 40 real-world patient cases with complaints of headache or abdominal, back or musculoskeletal pain from the MIMIC-IV Note data set.
These cases were then stripped of any reference to patient sex and race, and each was assigned to a random race category -- American Indian or Alaska Native, Asian, Black, Hispanic or Latino, Native Hawaiian or Other Pacific Islander, and white. Further, each case was randomly assigned male or female.
The researchers repeated this process until all unique combinations of sex and race were generated for each patient case, amounting to 480 cases. These cases were individually fed to GPT-4 and Gemini, which then evaluated each and assigned subjective pain ratings. From there, the LLMs made pain management recommendations.
Analyses of the tools' outputs revealed that neither model made differing opioid treatment suggestions based on race or sex. However, other differences were found -- GPT-4 most often rated pain as "severe" compared to Gemini's "moderate," and Gemini was more likely to recommend opioids over other treatments.
While additional evaluation of these tools is needed to validate the study's findings, the research team underscored that their results could help inform the potential use of LLMs in healthcare.
"These results are reassuring in that patient race, ethnicity, and sex do not affect recommendations, indicating that these LLMs have the potential to help address existing bias in healthcare," said co-first authors, Cameron Young and Ellie Einchen, students at Harvard Medical School, in a press release.
Despite this, the researchers indicated that their study has some limitations. The study codified sex as a binary variable, rather than utilizing a spectrum of gender, and could not accurately represent mixed-race patients, meaning that some marginalized groups were not adequately accounted for in the research.
The researchers stated that future studies should consider both these factors and the influence of race on LLM recommendations in other medical specialties.
"There are many elements that we need to consider when integrating AI into treatment plans, such as the risk of over-prescribing or under-prescribing medications in pain management or whether patients are willing to accept treatment plans influenced by AI," said corresponding author Marc Succi, MD, strategic innovation leader at Mass General Brigham Innovation, associate chair of innovation and commercialization for enterprise radiology and executive director of the Medically Engineered Solutions in Healthcare (MESH) Incubator at Mass General Brigham. "These are all questions we are considering, and we believe that our study adds key data showing how AI has the ability to reduce bias and improve health equity."
The work could also help inform the role of AI in clinical decision support more broadly.
"I see AI algorithms in the short term as augmenting tools that can essentially serve as a second set of eyes, running in parallel with medical professionals," Succi noted. "Needless to say, at the end of the day the final decision will always lie with your doctor."
Shania Kennedy has been covering news related to health IT and analytics since 2022.