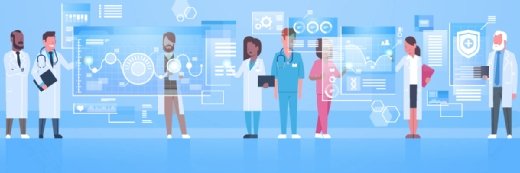
mast3r - stock.adobe.com
Predictive Models May Negatively Impact the Performance of Future Tools
The impact of predictive modeling on patient outcomes may negatively affect the predictive performance of subsequent artificial intelligence tools.
Researchers from the Mount Sinai Icahn School of Medicine and the University of Michigan have found that the deployment of predictive analytics tools may influence the predictive capabilities of current and future models.
The study, published this week in the Annals of Internal Medicine, aimed to assess how the implementation of predictive modeling in the clinical setting would impact patient outcomes and the predictive ability of subsequent models.
The research team demonstrated that leveraging the models’ insights can lead to adjustments in care delivery in an effort to improve outcomes. These patient outcomes are then captured in the electronic health record (EHR), which can establish a new “baseline” for model training and alter model performance over time.
The difference between the training data and the data fed to the model during deployment as patient characteristics shift is known as data drift, which can negatively impact the performance of machine learning models over time.
“We wanted to explore what happens when a machine learning model is deployed in a hospital and allowed to influence physician decisions for the overall benefit of patients,” explained first and corresponding author Akhil Vaid, MD, clinical instructor of Data-Driven and Digital Medicine (D3M), part of the Department of Medicine at Icahn Mount Sinai, in the press release.
“For example, we sought to understand the broader consequences when a patient is spared from adverse outcomes like kidney damage or mortality,” Vaid continued. “AI models possess the capacity to learn and establish correlations between incoming patient data and corresponding outcomes, but use of these models, by definition, can alter these relationships. Problems arise when these altered relationships are captured back into medical records.”
To explore these changes, the researchers simulated three scenarios using data from 130,000 critical care patients at Mount Sinai Health System and Beth Israel Deaconess Medical Center.
In the first scenario, the research team modeled what would happen if a predictive tool was retrained following deployment. Retraining is often recommended to address concerns about a model’s performance degradation over time, but the researchers found that the approach improved performance initially only to lead to further performance degradation.
This occurs because the model adapts to changing conditions at first, but then its performance degrades because the relationship between patient characteristics and outcomes that the model ‘learned’ shifts as a result of retraining.
The second scenario involved developing a new model after another had already been deployed.
The researchers indicated that a model trained to predict a condition like sepsis will be used to prevent sepsis and its associated adverse outcomes wherever possible. In this way, a tool to help prevent sepsis may also be used to prevent sepsis-related death.
However, experts may not be able to define the exact relationships between outcomes, which means that patients who previously received machine learning-guided care had improved outcomes, but their data is no longer appropriate to use in model training.
Here, any model implemented following an existing one trained for the same predictive task will be subject to data drift if it is trained using data from patients treated using the original tool.
The final scenario used two predictive models concurrently.
However, this approach showed that if those models make simultaneous predictions, using one set of predictions to guide clinical decision-making will make the other unnecessary.
In this case, the researchers noted that predictions should then be generated using freshly collected data, but doing so is often too impractical or costly to be viable.
These issues highlight some of the difficulties associated with utilizing predictive analytics in the clinical setting.
“Model performance can fall dramatically if patient populations change in their makeup. However, agreed-upon corrective measures may fall apart completely if we do not pay attention to what the models are doing—or more properly, what they are learning from,” said co-senior author Karandeep Singh, MD, associate professor of Learning Health Sciences, Internal Medicine, Urology, and Information at the University of Michigan.
However, the research team indicated that predictive models should not be viewed as unreliable. Instead, regular monitoring and maintenance of these tools is needed to ensure their effectiveness.
“We recommend that health systems promptly implement a system to track individuals impacted by machine learning predictions, and that the relevant governmental agencies issue guidelines,” Vaid stated. “These findings are equally applicable outside of health care settings and extend to predictive models in general. As such, we live in a model-eat-model world where any naively deployed model can disrupt the function of current and future models, and eventually render itself useless.”