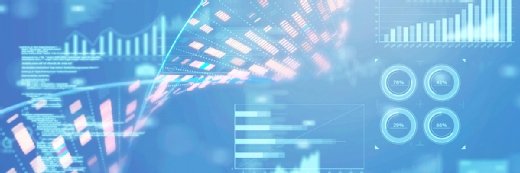
Yuichiro Chino/Moment via Getty
Using Machine Learning to Study Changes in Tumors After Immunotherapy
Engineers are developing a machine learning method to understand changes in tumors after immunotherapy.
By combining detailed mapping of the biochemical composition of tumors with machine learning, Johns Hopkins University engineers created the first non-invasive optical probe to understand the complex changes in tumors after immunotherapy.
“Immunotherapy really works like magic and has fundamentally changed the way we view how cancer can be managed,” a Johns Hopkins associate professor in mechanical engineering and co-author of the study, Ishan Barman, said in a press release.
“However, only around 25 percent of patients derive benefit from it, so there’s an urgent need to identify predictive biomarkers to determine who should receive the treatment.”
The study, which was conducted in collaboration with colleagues at the University of Arkansas, used a technique called Raman spectroscopy. With this technique, which uses light to identify the molecular composition of materials, the team probed colon cancer tumors in mice treated with the two types of immune checkpoint inhibitors used in immunotherapy.
“This is the first study that shows the ability of this optical technique to identify early response or resistance to immunotherapy,” said Santosh Paidi, one of the lead authors who worked on the research as a mechanical engineering PhD student at Johns Hopkins.
According to Paidi, one of the many benefits of Raman spectroscopy is it produces excellent molecular specificity. Additionally, the method is well-suited for discovering the compositional changes of the tumor microenvironment.
“Rather than homing in on a few suspected molecules, we’re interested in getting a more holistic picture of the tumor microenvironment. That’s because the tumor is not just the malignant cell. The microenvironment contains a complex combination of the tumor stroma, blood vessels, infiltrating inflammatory cells, and a variety of associated tissue cells,” said Barman.
“Our idea is to take this approach and systematize it so it can be used by doctors to determine whether immunotherapy will be beneficial for the patient.”
To train the algorithm to identify the range of features induced by immunotherapy, the team used about 7,500 spectral data points from 25 tumors.
“Our question was can we differentiate between the three groups, and then what are the specific spectral features that are allowing us to differentiate between them,” said Barman.
To build and test the machine learning method, the team used data from different mice. The goal was to mimic the biological variability the algorithm would encounter when facing new data.
“You need to prove beyond a doubt that the differences that you’re seeing are immune checkpoint inhibitor-induced as opposed to just differences between two individuals,” said Barman.
According to the team, the tests yielded positive results. The differences were subtle but statistically significant and consistent with proteomics analysis performed on the samples. While more research is necessary, the team indicated their work could pave the way to develop a method of predictive analytics to determine how a patient will respond to immunotherapy.
“Combined with machine learning, Raman spectroscopy has the potential to transform clinical methods for predicting therapy response,” said Paidi.