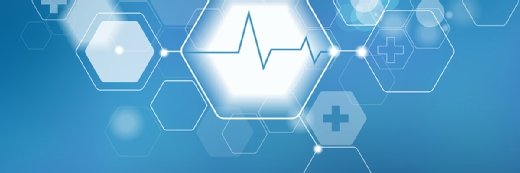
AlfaOlga/istock via Getty Images
How Machine Learning Enables Clinical Forecasting, Visualization
At Carnegie Mellon University, researchers have developed a reproducible machine learning tool to facilitate clinical predictions and data visualization.
At first glance, mining clinical data to extract meaningful insights seems like a task well-suited for machine learning algorithms. The principal aim of advanced analytics tools is to evaluate multiple sources of information and discover patterns and trends, and machine learning has proven valuable in accomplishing this task.
However, upon closer inspection, it’s clear that applying machine learning models to health records can be very challenging. Because this information is neither static nor regularly collected, it’s difficult to develop algorithms that are both transparent and reproducible.
Researchers from Carnegie Mellon University (CMU) recognized the need to build algorithms that would better facilitate the analysis of health information. The team designed Temporal Learning Lite (TL-Lite), a visualization and forecasting tool that aims to bridge the gap between clinical visualization and machine learning analysis.
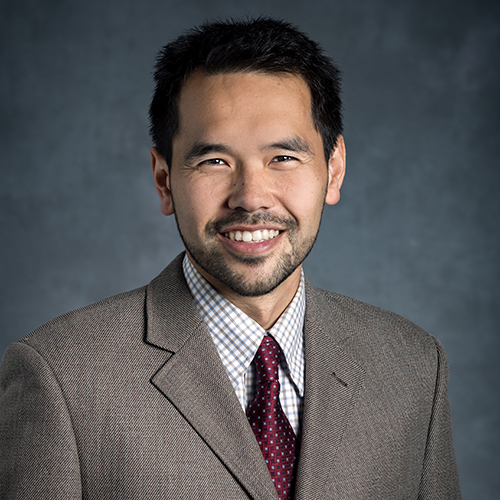
“TL-Lite is a visualization tool designed to bring healthcare experts closer to machine learning,” said Jeremy Weiss, assistant professor of health informatics at CMU’s Heinz College.
“The current paradigm for using machine learning in healthcare comes in two forms. The first is where teams of healthcare experts work with teams of data scientists, and they try to develop predictive algorithms to stratify patients. In the second form, machine learning researchers develop new algorithms and want to apply them to healthcare, but don’t have the necessary expertise to address specific, significant issues.”
Both of these processes are relatively slower than the method provided by the TL-Lite tool, Weiss noted.
“A healthcare expert can use TL-Lite to build a machine learning model for their cohort directly, without having to rely on others. By not requiring a team to be the intermediaries, and being able to run machine learning, healthcare experts can quickly and efficiently create the cohort and then build the models for interpretation themselves,” he said.
“The alternative to using a tool like TL-Lite is to use the research findings from other institutions at your own local institution, but there are several problems with that. For example, the data types may not look quite the same, or the patient populations might be slightly different. There are questions about the validity of the findings in studies conducted elsewhere.
The new model helps ease the process of clinical forecasting, resulting in well-specified and well-crafted predictions.
“This tool allows researchers to run that analysis at their own local sites, so that they get a better estimate of what's likely to happen in their patient population,” Weiss said.
TL-Lite begins with visualizations of information from databases and ends with visual risk assessments of a temporal model. Along the way, users can see the effects of their design choices through visual summaries at the levels of individuals as well as groups.
“The main problem with using machine learning in clinical care – and being able to make changes therein – is that there are many preprocessing design decisions that will affect the performance of the model. With this tool, healthcare experts are able to select those at their own location so that when they go to train these models, they're focused on the very specific task at hand,” said Weiss.
By seeing the impact of their design choices, users can understand their data more completely and adjust machine learning settings for their analysis. The tool allows healthcare experts to develop algorithms tailored to their patients and organizations.
“If you were to use a risk scoring system from another site, they might have defined the population based on the patient data that were available at entry and at the beginning of the hospitalization. But then the physician might want to have a risk score for a little bit later in treatment, maybe the first or second day after they've entered and they've already been stabilized,” Weiss explained.
“The outside model will not be tailored to that population and could give misleading predictions. Using TL-Lite, the physician can quickly train a model with the risk profiles for the particular population they’re interested in evaluating.”
While the tool has proven to be a valuable asset for researchers and providers, the team will continue to improve TL-Lite in future work.
“The tool uses a classic machine learning paradigm. However, the underlying infrastructure is able to use more sophisticated models, which may improve performance and lead to further insights. The tool is also a proof of concept, so it can be further developed to be easier to use,” said Weiss.
“Thirdly, the tool is used currently in collaborations of my group and certain healthcare professionals. Being able to get the physicians comfortable with using the tool is a work in progress.”
The team expects that their new method could support and enhance the implementation of machine learning in healthcare.
“The adoption of machine learning tools has primarily occurred in computer vision areas. We're now seeing more advanced machine learning algorithms that can take in other data types while maintaining properties that are desirable for interpretability and intelligibility. There's great promise for machine learning algorithms for forecasting and risk prediction,” Weiss concluded.
“TL-Lite is a way to help smooth that process and enable healthcare professionals to feel more comfortable with using machine learning, so that they can use the insights from these models in their day-to-day practice.”