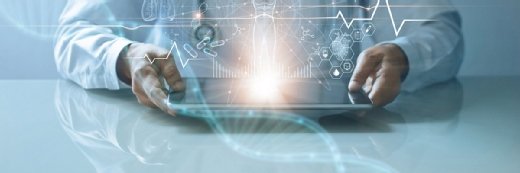
Getty Images/iStockphoto
AI Precision Medicine Approaches Disorganized, Slowed by Gaps
A Rutgers analysis shows that artificial intelligence approaches to precision medicine are advancing rapidly, but many suffer from disorganization and gaps.
Researchers at Rutgers have concluded that artificial intelligence (AI)-based approaches to precision medicine are limited or slowed by various structural issues, such as disorganization, data standardization, and gaps in approaches used.
The analysis evaluated and compared 32 of the most prevalent AI approaches to study preventive treatments for multiple diseases, including obesity, Alzheimer’s, inflammatory bowel disease, breast cancer and major depressive disorder. The researchers found that out of all the approaches evaluated, none could be used for all treatments, and programs that could evaluate multiple treatments were limited.
These findings, the authors note, indicate that the field of AI-based precision medicine is advancing rapidly, but suffers from significant disorganization, which has the potential to hamper future progress. Because AI and precision medicine both incorporate a wealth of different data and approaches, many different computing approaches have cropped up.
“Precision medicine is one of the most trending subjects in basic and medical science today,” said Zeeshan Ahmed, PhD, lead author on the study and an assistant professor of medicine at Rutgers Robert Wood Johnson Medical School, in the press release. “Major reasons include its potential to provide predictive diagnostics and personalized treatment to variable known and rare disorders. However, until now, there has been very little effort exerted in organizing and understanding the many computing approaches to this field. We want to pave the way for a new data-centric era of discovery in health care.”
The research team also argues that genetics is “arguably the most data-rich and complex component of precision medicine,” underscoring the importance of evaluating the objectives, methodologies, data sources, ethics, and gaps in approaches used for any precision medicine research or models.
To address the issues presented in the analysis, the researchers suggest that the scientific community embrace the major challenges, like improved data standardization and enhanced protection of personal identifying information, while also being vigilant for technical issues, such as needing to correct errors in genomic and clinical data.
“AI has the potential to play a vital role to achieve significant improvements in providing better individualized and population healthcare at lower costs,” Ahmed said. “We need to strive to address possible challenges that continue to slow the advancements of this breakthrough treatment approach.”
Limitations and challenges in AI approaches also limit advances in other areas.
In a recent interview with HealthITAnalytics, Rosalind Picard, founder and director of the Affective Computing Research Group at the Massachusetts Institute of Technology (MIT) Media Lab and principal investigator at MIT’s Jameel Clinic, outlined how limitations in AI and wearables negatively impact depression research.
Bias in AI is also a major hurdle slowing its uptake in healthcare, but strategies for avoiding algorithmic bias and research-based equitable healthcare algorithm frameworks are part of a growing effort to address these problems and promote health equity.