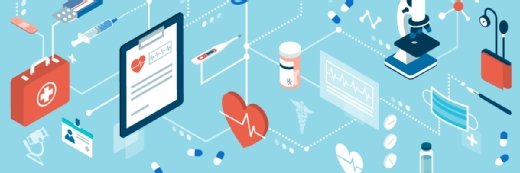
Getty Images
Personalized Predictive Models for Kidney Injury Outperform Traditional Methods
Researchers have developed personalized models for acute kidney injury risk estimation, which outperform standard models across diverse patient populations.
A new study published in JAMA Network Open earlier this month showed that personalized artificial intelligence (AI) models for acute kidney injury (AKI) risk prediction, which use common EHR variables, yielded improved and more equitable estimations than traditional models.
AKI occurs suddenly when the kidneys cannot filter waste products from the blood and can quickly become life-threatening, according to Mayo Clinic. The condition is most common in hospitalized patients, especially those critically ill or in the intensive care unit (ICU). Though AKI is severe and can be fatal, it can also be reversed depending on the patient’s overall health and other factors. Thus, risk estimation and effective intervention are critical.
The rise of personalized medicine has spurred an interest in individualized modeling and risk estimation, particularly for conditions common in hospitals and inpatient settings, the researchers noted. However, diseases with various causes, such as AKI, provide additional challenges for risk estimation. In this study, the researchers aimed to develop a personalized AKI risk estimation model and examine whether the model was more effective than traditional global and subgroup models.
The researchers began by analyzing EHR data from 76,957 inpatient encounters at one tertiary care hospital from Nov. 1, 2007, to Dec. 31, 2016. Patients over 18 years who had been hospitalized for two or more days during the study period were included in the analysis. Any patients with moderate or severe kidney dysfunction at admission were excluded.
The data was used to develop and validate global, subgroup, and personalized AKI risk estimation models. AKI was defined using the Kidney Disease: Improving Global Outcomes serum creatinine criteria, and the models were tasked with estimating AKI in general, high-risk, and low-risk patient cohorts.
AKI occurred in 7,259 patients. The personalized model outperformed both the global and subgroup models across all patient cohorts based on the area under the receiver operating characteristic curve (AUROC), a common metric used to evaluate the performance of AI models. The personalized model achieved an AUROC of 0.78 compared to the global model, which achieved an AUROC of 0.76 in general inpatients.
Similarly, the personalized model achieved an AUROC of 0.79 compared to 0.75 for the high-risk inpatients; and 0.74 compared to 0.71 for low-risk inpatients.
The personalized model achieved similar results compared to the subgroup models. It attained a mean AUROC increase of 0.05 over the subgroup models.
The researchers also evaluated the performance of the personalized model in detecting patients with AKI. They found that the personalized model can identify 11.97 percent to 20.95 percent more AKI patients than the global models and 7.18 percent to 13.45 percent more than the subgroup models.
These findings indicate that personalized modeling offers an improved AKI risk estimation approach that can be used across diverse patient subgroups, the researchers stated. However, more research is needed to further advance clinical and personalized risk estimation in this area.