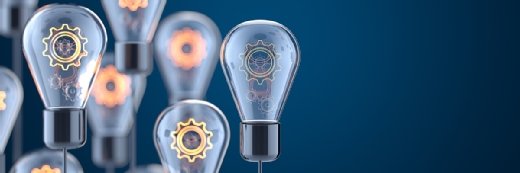
Getty Images/iStockphoto
Audits, Diversifying Data Can Help Payers Address Machine Learning Biases
While bias can develop in machine-learning practices, experts explain how health insurers can address potential inequalities by using diverse data and regularly auditing algorithms.
As health insurers and providers increasingly turn to machine-learning algorithms to enhance care, there is a growing concern among experts regarding equity, fairness, and bias in how the technology is developed and implemented.
In an analysis article, researchers from several insurance companies and university research centers presented a guide highlighting where bias can arise when using machine learning.
“We suggest mechanisms for identifying and dealing with bias and discuss challenges and opportunities to increase fairness through analytics in the health insurance industry,” the analysis stated.
Health insurers will often use predictive modeling to identify members with complex health needs for care coordination and condition management. To identify and prioritize these members for outreach, health plans will use a combination of risk scores, data from one or more predictive models, and strategic plans.
Due to risk-based prioritization strategies driving the allocation of health resources, researchers recommend that algorithms undergo regular audits to search for potential biases.
Predictive models can often produce biases when assessing chronic diseases. According to the CDC, six in 10 US adults have a chronic illness, and four in 10 have two or more chronic conditions. Chronic illnesses can lead to death, disability, and reduced quality of life.
Additionally, chronic disease accounts for trillions of dollars in annual healthcare costs. Despite this, many chronic diseases can be effectively managed through smoking cessation, nutrition counseling, and medication adherence programs. As a result, health insurers commonly use models to predict the likelihood of chronic disease among their members.
But a fundamental source of bias that could occur when a predictive model is being developed is the initial selection of the chronic condition.
“Models are less common for diseases that tend to affect smaller or minority segments of the member population (such as sickle cell anemia) or that might not have well-defined or easily scalable interventions," the analysis explained. "Yet targeting such conditions could greatly impact morbidity, mortality, and healthcare costs for those with the condition.”
Biases can also be detected when predicting the likelihood of hospitalization due to racial and socioeconomic disparities.
“Disparities in access and use mean that some subpopulations are underrepresented in the target population and in the data used to predict the outcome of interest," according to the analysis. "Thus, the resulting model output may reflect those systemic biases, and interventions or policy decisions based on the model outputs risk reinforcing and exacerbating existing inequities.”
Health insurers also use predictive models to assist member outreach strategies for improving medication adherence.
These models are developed using regression or classification approaches. Regression-based approaches typically predict the proportion of days covered, defined as the proportion of days during a calendar year that a member has access to their medications. The target threshold is for members to have access to their medication for more than 80 percent of the calendar year.
Machine learning can help identify patients at risk for being non-compliant with a new medication regimen or falling below an optimal level of adherence. However, the researchers explain that health plans and other organizations that develop and use medication adherence models must recognize how systemic biases in access to pharmacies and prescription drugs impact communities of color.
Despite the potential of biases in machine-learning practices, the research team detailed steps to avoid unjust practices. A critical step is understanding that not all healthcare challenges will have a one-size-fits-all solution. Before carrying out machine-learning strategies, it is essential to ensure that the technology uses diverse data representative of various population subgroups.
Additionally, researchers should continuously assess the machine-learning algorithms as new data is presented.
“Opportunities exist to ensure that machine learning is fair, not only on ethical grounds but also on strong operational and business grounds,” the researchers concluded.