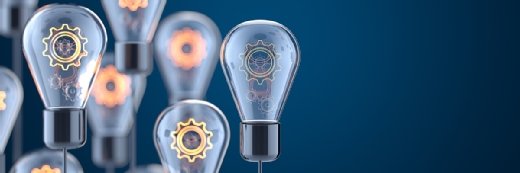
Getty Images/iStockphoto
Predictive Analytics Model Underperforms in Black Populations
A predictive analytics model does not accurately identify black patients at high risk of lung cancer.
A commonly used predictive analytics model that forecasts lung cancer risk underperforms in Black populations, suggesting the need for improved screenings and guidelines.
In a study published in JAMA Network Open, researchers noted that lung cancer is the third most common cancer in the US and the leading cause of cancer death. About 80 percent of the total 154,000 lung cancer deaths recorded each year are caused by cigarette smoking.
Black men are more likely to develop and die from lung cancer than individuals from any other racial and ethnic group, showing significant racial disparities in outcomes of the disease. According to the team, research has shown that Black patients are less likely to receive early diagnosis and treatments for lung cancer.
"Black individuals develop lung cancer at younger ages and with less intense smoking histories compared to white individuals," said senior author Julia Barta, MD, Assistant Professor of Medicine in the Division of Pulmonary and Critical Care Medicine at Thomas Jefferson University, and researcher at the Jane and Leonard Korman Respiratory Institute.
"Updated guidelines now recommend screening eligible patients beginning at age 50, but could still potentially exclude higher-risk Black patients. We are interested in finding methods that could help identify at-risk patients who are under-screened."
Screening for lung cancer involves a yearly CT scan to detect lung cancer in otherwise healthy people with a high risk of developing the disease. Current guidelines do not require a risk score for screening eligibility, but some researchers believe risk models could improve care. Existing risk prediction models are derived from screening data that only include five percent or fewer African American individuals.
"What makes our study unique is that our screening cohort included more than 40 percent Black individuals," said Barta, who is also a member of Sidney Kimmel Cancer Center - Jefferson Health.
"To our knowledge, our study is the first to examine lung cancer risk in a diverse screening program and aims to strengthen the argument for more inclusive guidelines for screening eligibility."
The most well-validated model used in screening research is the Prostate, Lung, Colorectal and Ovarian Cancer Screening Trial modified logistic regression model (PLCOm2012).
"It uses ten to 12 risk factors that include age, race, smoking history, as well as some socioeconomic factors like education to calculate a risk score," said Christine Shusted, MPH, first author of the study and research data analyst for Jefferson’s Lung Cancer Screening Program through the Korman Respiratory Institute at Thomas Jefferson University.
"The higher the score, the higher the risk of developing lung cancer. We wanted to see how well this model identifies patients with the highest risk of lung cancer in this diverse patient population."
Researchers conducted a cross-sectional, retrospective study in 1,276 Black and white patients who enrolled in the Jefferson Lung Cancer Screening Program between January 2018 and September 2020.
From this screening cohort, researchers detected lung cancer in 32 patients, 44 percent of whom were Black. These patients made up the cancer cohort. The team then calculated risk scores using the PLCOm2012 model.
The results showed that in the screening cohort, more Black patients than white patients were in high-risk groups, indicating that Black patients in this cohort had a higher risk of developing lung cancer. White patients with screen-detected lung cancer generally had high lung cancer risk scores.
"Among Black patients, we would have expected to see a similar trend," said Barta. "However, we saw that despite having a lung cancer diagnosis through screening, Black patients were actually defined as lower risk. This indicates that the model is not accurately predicting risk of lung cancer in Black patients."
The study points to a larger issue of the potential for bias and exacerbated disparities in data analytics models.
"These findings allowed us to identify weaknesses in this model for risk calculation for lung cancer," said Shusted.
"It indicates that we need to not only expand criteria for lung cancer screening so that more diverse populations are included, but that these prediction models need to include factors, like environmental contributors, access to healthcare, and other social determinants of health."
The results of this study come on the heels of similar findings by a team from Kaiser Permanente.
In a report published in JAMA Psychiatry, Kaiser Permanente researchers demonstrated that suicide risk prediction models that perform well in the general population may not be as accurate for Black, American Indian, and Alaska Native people.
The studies both show that the increased use of data analytics models in healthcare should be met with caution by researchers, developers, and provider organizations.
The Thomas Jefferson University team plans to continue to build on these findings, and ultimately wants to define comprehensive risk factors and improve lung cancer screening uptake and adherence – particularly among vulnerable populations.
“This work is an important step to reducing disparities in the screening and early detection of lung cancer, and making sure we can trust our models to predict those individuals at the highest risk," said Barta.