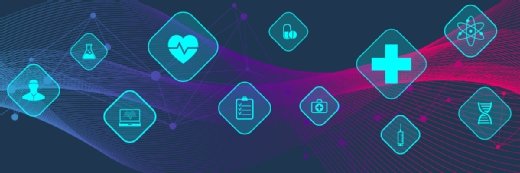
BAIVECTOR - stock.adobe.com
Machine Learning Detects Biomarkers of Autism Spectrum Disorder
Machine learning tools were able to identify biomarkers in blood that could enable earlier diagnosis of children with autism spectrum disorder.
Machine learning tools analyzed hundreds of proteins and identified blood biomarkers that could speed the diagnosis of autism spectrum disorder (ASD), according to a study published in PLOS One.
ASD impacts at least one out of every 59 children in the US, researchers noted. The condition is also associated with significant personal, family, and societal costs. Efforts to determine the underlying biology of ASD, as well as ASD prevention, early diagnosis, and effective treatment, are public health priorities.
Being able to identify children with autism when they’re toddlers could make a big difference, the team stated. Currently, the average age of a child diagnosed with ASD in the US is four years old.
Diagnosis before the age of four means that a child is more likely to receive an effective, evidence-based treatment, including therapies directed at core ASD symptoms like inflexible behaviors and lack of communication skills.
Researchers have investigated many blood-based biomarker candidates, including neurotransmitters, cytokines, and markers of mitochondrial dysfunction, oxidative stress, and impaired methylation. However, because ASD is so prevalent, using machine learning to incorporate demographic and clinical data into the analysis could more powerfully examine disease status and symptom severity.
For the study, the team examined serum samples from 76 boys with ASD and 78 from typically developing boys aged between 18 months and eight years. The results showed that all nine proteins in the biomarker panel were significantly different in boys with ASD compared with typically developing boys. Researchers found that each of the nine serum proteins correlated with symptom severity.
Researchers evaluated more than 1,100 proteins using the SomaLogic protein analysis platform. The group identified a panel of nine proteins as optimal for predicting ASD using three computational methods. Researchers then evaluated the biomarker panel for quality using machine learning methods.
“The more significantly affected the child is, the higher or lower than normal the blood biomarker is,” said Dwight German, PhD, professor of psychiatry at UT Southwestern and senior author of the study.
“Ideally, there will be a day when a child is identified using blood biomarkers as being at risk for developing ASD and therapies can be started immediately. That would help the child develop skills to optimize their communication and learning.”
The team noted that future studies will need to fully validate the present findings.
“Although the sample size is acceptable for a discovery study, the data presented here are preliminary, and a larger validation study is needed to be certain of the value of the biomarker panel. Due to the increased prevalence of ASD in boys, this study only enrolled boys, which does not allow for an investigation of gender-specific differences,” researchers noted.
The researchers expect that the study will pave the way for earlier diagnosis of autism.
“The earlier we can identify children with autism, the more understanding we can gain on ways to provide support and therapies that will improve their quality of life,” said Laura Hewitson, PhD, at The Johnson Center for Child Health & Development, a multidisciplinary treatment center in Austin, Texas, that uses a unique combination of clinical care, research, and education to further the understanding of ASD and related developmental disorders.
Researchers have previously turned to AI and data analytics techniques to enable earlier autism diagnosis. A study recently published in Nature Medicine showed that a precision medicine method enabled by artificial intelligence could lead to the first biomedical screening tool for a subtype of autism.
“Our study is the first precision medicine approach to overlay an array of research and healthcare data—including genetic mutation data, sexually different gene expression patterns, animal model data, EHR data, and health insurance claims data—and then use an AI-enhanced precision medicine approach to attempt to define one of the world's most complex inheritable disorders,” said Yuan Luo, associate professor of preventive medicine: health and biomedical informatics at the Northwestern University Feinberg School of Medicine.