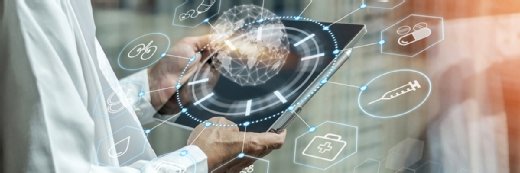
Chinnapong - stock.adobe.com
Artificial Intelligence Falls Short in Detecting Diabetic Eye Disease
Of seven artificial intelligence algorithms tested, only one performed better than human clinicians in detecting diabetic eye diseases.
Artificial intelligence algorithms designed to detect diabetic eye disease may not perform as well as developers claim, according to a study published in Diabetes Care.
Diabetes is the leading cause of new cases of blindness among adults in the US, researchers stated. The current shortage of eye-care providers would make it impossible to keep up with demand to provide the requisite annual screenings for this population. And because current approaches of treating retinopathy are most effective when the condition is caught early, eye doctors need an accurate way to quickly identify patients who need treatment.
To overcome this issue, researchers and vendors have developed artificial intelligence algorithms to help accurately detect diabetic retinopathy. Researchers set out to test the effectiveness of seven AI-based screening algorithms to diagnose diabetic retinopathy against the diagnostic expertise of retina specialists.
Five companies produced the algorithms tested in the study – two in the US, one in China, one in Portugal, and one in France. While many of these companies report excellent results in clinical trials, their performance in real-world settings was unknown.
Researchers used the algorithm-based technologies on retinal images from nearly 24,000 veterans who sought diabetic retinopathy screening at the Veterans Affairs Puget Sound Healthcare System and the Atlanta VA Healthcare System from 2006 to 2018.
The team conducted a test in which the performance of each algorithm and the performance of the human screeners who work in the VA teleretinal screening system were all compared to the diagnoses that expert ophthalmologists gave when looking at the same images.
The results showed that the algorithms don’t perform as well as human clinicians. Three of the algorithms performed reasonably well when compared to the physicians’ diagnoses and one did worse, with a sensitivity of 74.42 percent.
Just one algorithm performed as well as human screeners in the test, achieving a comparable sensitivity of 80.47 percent and specificity of 81.28 percent.
Researchers also found that the algorithms’ performance varied when analyzing images from patient populations in Seattle and Atlanta care settings – indicating that algorithms may need to be trained with a wider range of images.
“It’s alarming that some of these algorithms are not performing consistently since they are being used somewhere in the world," said lead researcher Aaron Lee, assistant professor of ophthalmology at the University of Washington School of Medicine.
The team noted that differences in camera equipment and technique could be one explanation, and that their study demonstrates how critical it is for practices to test AI screeners and follow the guidelines about how to properly obtain images of patients’ eyes, because the algorithms are designed to work with a minimum quality of images.
While many studies highlight the potential for AI and machine learning to enhance the work of healthcare professionals, the findings of this research show that the technology is still very much in its infancy. Additionally, the results suggest that while these algorithms may have a high degree of accuracy and sensitivity on their own in the research realm, they may benefit from human input when being used in real-world clinical settings.
Separate studies have found that advanced analytics tools are most effective when combined with the expertise of human providers. In October 2019, a team from NYU School of Medicine and the NYU Center for Data Science showed that combining AI with analysis from human radiologists significantly improved breast cancer detection.
“Our study found that AI identified cancer-related patterns in the data that radiologists could not, and vice versa,” said senior study author Krzysztof J. Geras, PhD, assistant professor in the Department of Radiology at NYU Langone.
“AI detected pixel-level changes in tissue invisible to the human eye, while humans used forms of reasoning not available to AI. The ultimate goal of our work is to augment, not replace, human radiologists,” added Geras, who is also an affiliated faculty member at the NYU Center for Data Science.
In order to ensure humans aren’t left out of the equation, some researchers are working to develop algorithms that have the option to defer clinical decisions to human experts. A machine learning tool recently designed by MIT’s Computer Science and Artificial Intelligence Lab (CSAIL) is able to adapt when and how often it defers to human experts based on factors such as the expert’s availability and level of experience.
“Our algorithms allow you to optimize for whatever choice you want, whether that’s the specific prediction accuracy or the cost of the expert’s time and effort,” said David Sontag, the Von Helmholtz Associate Professor of Medical Engineering in the Department of Electrical Engineering and Computer Science.
“Moreover, by interpreting the learned rejector, the system provides insights into how experts make decisions, and in which settings AI may be more appropriate, or vice-versa.”