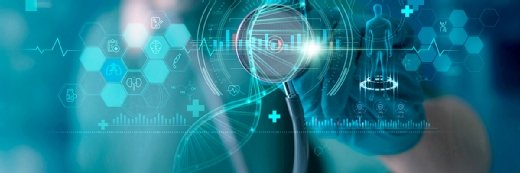
ipopba/istock via Getty Images
Predictive Analytics Determines Outcomes in ED Patients with COVID-19
Predictive analytics can accurately forecast whether ED patients with COVID-19 symptoms will experience severe disease or death.
A predictive analytics platform was able to accurately determine the probability of death or need for critical care within seven days for emergency department patients with COVID-19 symptoms, according to a study published in the American Journal of Emergency Medicine.
For more coronavirus updates, visit our resource page, updated twice daily by Xtelligent Healthcare Media.
The tool can help ensure that patients get the right care when they need it, researchers stated. Additionally, the platform could be particularly helpful to providers deciding whether to hospitalize or discharge patients with COVID-19 symptoms at the time of an emergency department visit.
“As the pandemic surges again across the country and hospitalizations are increasing, front-line physicians often must make quick decisions to hospitalize or discharge patients with COVID-19 symptoms,” said Adam Sharp, MD, an emergency medicine physician who also is a researcher for the Kaiser Permanente Southern California Department of Research & Evaluation.
“We created a risk assessment that looks at many different patient variables that might predict a critical poor outcome — without any lab or diagnostic testing.”
Because of the unique clinical features of COVID-19, there is a significant need for a novel risk score using data available at the time of the ED encounter, the team stated. Previous studies have focused on hospitalized patients, with little information about those treated outside the inpatient setting.
Researchers set out to examine ED patients with suspected COVID-19 and provide helpful information to frontline physicians and inform care decisions, particularly during surges when hospital capacity is limited.
The team evaluated 26,600 emergency department visits by adults with COVID-19 symptoms between March 1, 2020 and April 30, 2020 – a period when significant community spread of COVID-19 was prevalent in southern California but immediate COVID-19 test results were not.
Researchers developed a predictive analytics tool that looked at patients’ comorbidities, obesity, vital signs, age, and sex – called the COVAS score – to guide clinical decisions in emergency departments and urgent care centers.
The results showed that the tool achieved an AUC of 0.891 in the derivation cohort, and an AUC of 0.895 in the validation cohort. The COVAS score was also able to accurately predict the probability of death or need for critical respiratory care within seven days for patients seen at Kaiser Permanente emergency departments in southern California.
The results can inform frontline health professionals and health systems about which patients with COVID-19 symptoms are at greater risk of death or respiratory decompensation that would require ventilation or high-flow oxygen.
“I have used this tool successfully with my own patients in the emergency department and it helps provide necessary information to make informed decisions with patients,” Sharp said. “It can help to reassure low-risk patients, and alternatively can encourage those at high risk to receive hospital-based care that may speed their recovery.”
The team noted that their study builds on previous research about COVID-19 outcomes and risk factors by focusing on patients in the emergency department.
“A strength of our study is the use of a heterogeneous sample which is representative of the types of patients that frontline emergency, urgent care and primary care physicians are currently evaluating, and the seven-day timeline is more relevant than longer periods for acute care decisions,” researchers concluded.
“Our study also avoids the current challenges related to the availability of SARS CoV-2 testing, and with the uncertain accuracy of different testing strategies, especially at a time when it is challenging to distinguish between COVID-19 and other infections. Targeting an ED patient population adds to the previous reports from hospitalized patient cohorts.”
Artificial intelligence tools have increasingly helped researchers accurately forecast COVID-19 outcomes. A team from Mount Sinai recently developed machine learning models that can predict the likelihood of critical illness or mortality in COVID-19 patients, which could help clinicians better care for and manage individuals infected with the virus.
The models performed best when predicting a critical event or mortality seven days ahead of time, when the tools were able to correctly flag the most critical events while returning the fewest false positives.
“We have created high-performing predictive models using machine learning to improve the care of our patients at Mount Sinai,” said Girish Nadkarni, MD, Assistant Professor of Medicine (Nephrology) at the Icahn School of Medicine, Clinical Director of the Hasso Plattner Institute for Digital Health at Mount Sinai, and Co-Chair of MSCIC.
“More importantly, we have created a method that identifies important health markers that drive likelihood estimates for acute care prognosis and can be used by health institutions across the world to improve care decisions, at both the physician and hospital level, and more effectively manage patients with COVID-19.”