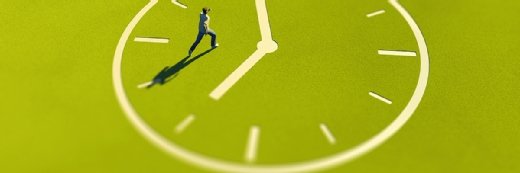
Getty Images
How to Effectively Integrate AI into Clinical Trials
PharmaNewsIntelligence interviews Tobias Guennel on effective AI integration, safety, and validation in clinical trials.
Since its inception, artificial intelligence (AI) has continually disrupted practices across all industries. As more and more healthcare researchers and clinicians adopt AI, experts have noted the impact of AI on clinical research and trial management. PharmaNewsIntelligence sat down with Tobias Guennel, PhD, SVP of Product Innovation and Chief Architect, QuartzBio, part of Precision for Medicine, to discuss AI integration, validation, and security in clinical trials.
AI Applications in Clinical Trials
The applications of AI in clinical trials are vast and can take on multiple forms. From identifying drug targets to recruiting eligible clinical trial candidates to data analysis, AI has the potential to streamline and speed up the clinical research process. AI applications in clinical trials include the following.
- Preclinical research: AI and machine learning (ML) algorithms can sift through the vast amounts of clinical trials and data already available before a clinical trial begins. Researchers can use the technology to extrapolate data that helps them determine the appropriate patient population for their study and other factors that may guide clinical research.
- Drug discovery and repurposing: Similar to preclinical research applications, AI can play a crucial role in drug discovery by analyzing existing data on particular compounds or identifying patterns that reveal how a drug with one indication can be repurposed to treat another condition.
- Clinical trial design: AI can provide insights into a study's best clinical trial designs by evaluating large data sets, including published research.
- Patient recruitment: AI can screen potential clinical trial participants by analyzing patient data in electronic health records and determining who would be a good candidate.
- Monitoring: Researchers can use AI-powered remote patient monitoring tools to collect and analyze real-time patient data.
- Data analysis: After a clinical study is completed, researchers can use AI algorithms to analyze patient data, track patient outcomes, and draw conclusions.
Throughout his discussion with PharmaNewsIntelligence, Guennel focused on two components of AI application in clinical research settings.
The first component refers to the historical approach. “AI is not a new concept; it's been around for a while,” Guennel explained. “Typically, it's been used to support core research activities, especially around drug target identification, validation, and screening.”
Guennel explains that novel approaches have enabled models to surpass current applications and synthesize information from the public domain.
“For example, by going through vast amounts of unstructured data, pulling out key information through generative AI technology, and synthesizing that information into usable information, we can inform research and development,” he explained.
The second component of AI applications in clinical research is supporting data management by integrating diverse datasets.
Impact
PharmaNewsIntelligence asked Guennel to explain how integrating AI early in the clinical trial process impacts workflow and the success of clinical trials.
“[Clinical trials] can leverage AI during the actual trial planning phase. That's more in the realm of the traditional approaches of identifying the right patient populations and drug targets with the highest likelihood of success,” he responded.
This has been used to improve clinical trial design and early decision-making. Guennel explained that over the past five years, advancements in AI and data processing models have enabled researchers to run more significant, complex models, allowing them to integrate more information. Researchers may be able to generate synthetic data to mimic potential trials and assess the probability of a drug’s success before designing or launching a clinical trial.
Beyond clinical trial planning, AI is applied in the early phases of drug development, preclinical research, and phase one and two clinical trials. This early integration may minimize clinical trials or research and development spending by identifying good drug targets early in the process.
Additionally, Guennel highlights that researchers can generate large data sets with new technology; however, AI integration provides an easy way to analyze data and pull out valuable information, improving data analytics efficiency.
He emphasizes that AI transforms and accelerates the time from generating a research question to collecting data to pulling out valuable insights from the dataset.
Challenges
Although AI can play a meaningful role in clinical trials and data analysis, some people still hesitate to adopt these new strategies.
“The hesitancy and challenges are like any new, disruptive technology getting its foot in the door with healthcare and life sciences,” Guennel notes. “The reality is we're working with highly sensitive data, sensitive in the sense it's IP for sponsors. It is highly sensitive patient information data, and the first thing that everybody's always worried about is data privacy and security.”
He explained that most healthcare companies' practical approach is to secure the infrastructure used to run the AI algorithms or technology. Ensuring that the model is not exposed to the public and securing it like any other technology used in healthcare settings, facilities, researchers, and companies integrating AI can ensure that the data remains secure.
In addition to privacy and security, another challenge is validating models to ensure they produce accurate information.
“In the general sense, you hear a lot of times about AI hallucinating and making up information,” he explained, referencing general rhetoric about AI fabricating data or generating unvalidated or inappropriate responses.
Considering the risk associated with AI and information fabrication, Guennel underscores the importance of a robust validation protocol and strategy to ensure that the models give reliable information.
Validation has two main components. First, having a high-quality data set is critical because no applicable information can be generated from unreliable or poor-quality data sets.
Part of having a high-quality dataset is including diverse data that minimizes bias.
“Bias in clinical research can come in various areas,” remarked Guennel.
Bias can favor specific patient populations if the data used is based on a uniform patient population. Guennel emphasizes that to avoid bias in AI models, experts need to focus on the kind of data used to train the models. Ultimately, a broad representation in the data is the best way to ensure that models are trained to assess a more diverse patient population with limited bias.
To minimize bias, the people developing and training the models must ensure that all training and validation steps account for various use cases, data elements, and end users.
“It's difficult to always get a completely unbiased approach, but researchers want to ensure that they minimize it as much as possible,” he repeated.
Even with a high-quality data set, Guennel notes that the second component is ensuring that models are trained with sufficient subject matter expertise and domain knowledge. The AI model should understand the scientific terms end-users are asking to respond with valid information.
Regulation
The rapidly changing pace of AI and technology has sparked discussion about how regulatory environments will or should change in the face of new privacy and security issues. Guennel that there are two general thought processes about regulatory changes.
“The first one is that yes, adjustments are being made on how maybe policies are being implemented, but the general concepts and guidance that are being provided are not drastically changing,” he explained, noting that regulatory agencies still expect researchers to adhere to good clinical practices and good data practices.
For example, applying a strong software development lifecycle when integrating AI into platforms and workflows is a standard good data practice in data privacy and security.
However, traditional software validation is very transactional with clear regulatory guidelines, but the advancements of AI and widespread applications make it difficult to have explicit protocols.
“That paradigm can shift simply because the realm of possibilities that an AI model could cover is much larger, and they cannot test every single edge case in that paradigm.”
Instead, regulatory agencies use risk-based approaches that depend on how and where AI is integrated to determine whether a particular company or researcher follows good clinical practice. With AI's complicated and intricate nature, different regulatory agencies have developed task forces to dialogue challenges in regulation.
“Regulatory agencies say researchers still have to abide by the best principles. However, these new paradigms are maybe pushing the boundaries of how these best practices have typically been implemented, and they want to have an open dialogue to see how those existing processes may have to be adjusted to support those new technologies,” stated Guennel.
Future Predictions
As AI continues to advance and develop, industry leaders must learn to accommodate and optimize their use of the technology while ensuring patient safety and privacy.
“[The industry] likely will see that there's a continued focus on optimizing the learning capabilities of AI models to empower insights and outcomes and allow researchers to deliver more value and more speed and accuracy of that information being generated to support drug development,” predicted Guennel.