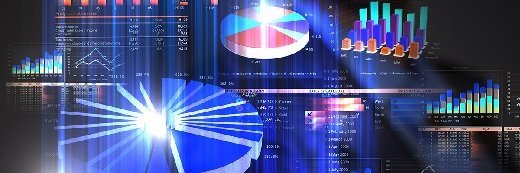
Sergey Nivens - Fotolia
Data analytics projects need structure, says former Avis analytics exec
In this Q&A, Ashok Kumar made one thing clear for CIOs: Before they touch an AI project, they need to get their data house in order.
When Ashok Kumar was helping lay the groundwork for an enterprise AI practice at Avis Budget Group, he started with the basics, which include self-assessment and a call for a "holistic process" to all data analytics projects.
As a department of one, Kumar, former director of business intelligence and analytics, described the analytics practice at Avis as "fragmented." He said one way to change that is to establish a center of excellence that can tackle data analytics projects and assist business units in productionalizing and scaling efforts that are important to a company.
Ahead of Kumar's keynote talk at EGG NYC 2018, an artificial intelligence conference hosted by software company Dataiku, he sat down to talk about why it's important for CIOs to get their data and analytics houses in order before they tackle AI.
Editor's note: This interview has been edited for brevity and clarity.
You recommend a holistic process for data analytics projects. Why is that so important?
Ashok Kumar: Once you have an insight, sometimes it's not easy to put that inside of an action, especially when it comes to things like operational considerations and privacy considerations. So, you've done this project and you've got this great insight, and now you don't know how to use it. Also, data is an issue -- getting the right amount of data, understanding how to get the data together before doing any deep analytics.
We need to look at all of those things before we get started to get a full picture of what it's going to take. So, we need to ask: What kind of insight are you looking for? What data do you need to get to that insight? And, most importantly, how do you put that insight into action?
Who owned analytics at Avis?
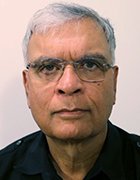
Kumar: We had a bit of what I would call fragmented analytics. Much of it is embedded in the business, and there are certain areas where the business drives analytics. At the same time, we're trying to build a center of excellence, which is going to be housed in IT.
This is one of the challenges. The business [units] have created their own analytics silos. They want to do analytics; they don't want to wait for IT. At the same time, they need support from IT to scale [out] and productionalize things. So, how do we create that overall structure? The center of excellence would establish a process on how to execute analytics projects with appropriate involvement from the business and from IT.
What would that structure look like?
Kumar: There are a couple of options: One is to create a central organization where data scientists are embedded with business units. Another is to have a fully decentralized model, which is where we are right now.
Or, we could maybe create a fully centralized organization but use a kind of federated model, where you have businesses that can do their data science projects with support from IT, but IT can also provide data science capabilities to business units on certain bases.
Centers of excellence can be a hard sell. Any tips on how to do this right?
Kumar: I would tend to agree with you that a lot of times when you start saying things like center of excellence, there's this tendency for the eyes to glaze over. Regardless of what we call it, we need to be clear that there's heavy involvement from business, and IT doesn't come across as dictating what the business needs to do.
Also, I find that if we go out there and start talking about, say, machine learning algorithms from day one, the business gets a little ticked off that we're selling another technology versus trying to help solve a problem.
Let's talk about the data architecture you helped put in place in order to enable your data analytics projects. For example, I understand you're using AWS. Why?
Kumar: Before AWS, we were actually with [Microsoft] Azure. This was almost four years ago. We had an immediate need to start doing some BI [business intelligence] work using Tableau, and we chose Azure simply because we had a relationship with Microsoft at the time. We were using Azure primarily as an infrastructure as a service at that time, so we weren't taking advantage of all of the tooling that Microsoft has been working on, and we found it to be expensive and not very scalable.
Over time, we decided to explore AWS. We did a fair bit of analysis as to what rearchitecting in AWS would that look like. And we felt that AWS could provide not only a cost advantage, but that it could also give us the scale we were looking for with its elastic computing model and additional services. And we're pretty happy with it.
You also helped the company establish a data lake. Where does it live and what's in it?
Kumar: In AWS. It lives in [Elastic Map Reduce]. ... The genesis of our data lake was to use enterprise data first. Our initial data lake was mostly sourced from our data warehouse simply because we wanted to have a scale and BI capabilities that we could not get from our warehouse.
Now, we are augmenting that with all the user data and all the other nonenterprise data sources. So, it was a little bit in the reverse order than you typically might see. We don't have all of the enterprise data in the data lake, but do we have a fair bit, and we are expanding as we need it.