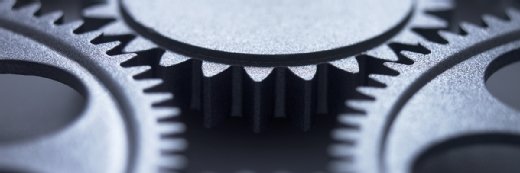
Getty Images
Researchers Develop eCQMs for Cancer Diagnostic Process, Follow-Up
Healthcare organizations could use eCQMs to incentivize cancer diagnostic performance improvement and develop interventions to reduce missed follow-up opportunities.
Electronic clinical quality measures (eCQMs) to assess quality of cancer diagnosis could help improve diagnostic performance, according to a study published in JAMIA.
Researchers developed and implemented two eCQMs to automate the measurement of diagnostic performance in cancer at two large health systems.
One eCQM measured missed opportunities in diagnostic evaluation for colorectal and lung cancer, while another quantified follow-up after abnormal test results suspicious for cancer.
At one site, the researchers could only implement one of the measures because of a lack of radiology codes indicating suspicion of malignancy.
“The inability to implement the lung cancer measure at one site and low positive predictive value (PPV) at the other site highlights the challenges in working with unstructured EHR data,” the authors wrote.
“Because this measure relies on identifying abnormal imaging results categorized as suspicious for malignancy by radiologists, it cannot function in settings where this categorization does not occur, such as at site two,” they explained.
Additionally, the authors noted that lung nodules suspicious for malignancy often have low risk features in patients with no risk factors, requiring more flexible timeframes for follow-up than the eCQM measure allowed.
“Discerning these differences in follow-up requires radiologists to categorize levels of urgency while interpreting images,” the researchers pointed out.
The American College of Radiology’s Lung-RADS system offers a common lexicon that provides this capability. Use of the system is growing but not yet widespread, the researchers noted.
“Alternatively, methods such as natural language processing could convert radiologist-dictated findings into expected follow-up timeframes, which could be useful for future measures,” they added. “While some efforts to apply such advanced algorithms have shown early success, implementation currently requires specialized data mining knowledge and may not be immediately available at many organizations.”
The study demonstrates a successful first step in developing quality measures for cancer diagnostic performance. Healthcare organizations could use such measures to assist quality improvement teams in developing interventions to reduce missed follow-up opportunities.
“While our quality measures were implemented at the organizational level, measures could also be focused on a particular clinic or clinician or to recognize high-functioning practices with robust follow-up pathways,” the authors wrote.
“Rather than be used as interruptive clinical decision support or EHR notifications to clinicians, which may get ignored, these eCQMs can facilitate monitoring procedures at the practice or organizational level and help create lists of patients who did not receive follow-up,” they continued.
The study emphasized that future efforts are needed to expand the types of diagnostic performance measures available and understand the effect of eCQM implementation on cancer outcomes.
The researchers acknowledged several limitations that may impact the generalizability of their study.
First, the study implemented eCQMs at two large healthcare systems where patients receive most of their care. Measures may not be generalizable to smaller practices or practices where data from other healthcare organizations may not be easily accessible.
However, the growing use of health information exchanges (HIEs) boosts the likelihood that data necessary for such measures will be accessible to practices of all sizes in the future, the authors said.
The study is also limited because the researchers performed validation based on chart reviews, which do not always represent care delivered.
“However, clinical documentation is currently the most commonly used method to assess care quality,” they wrote.