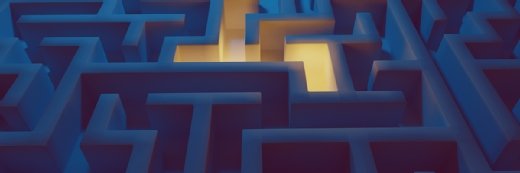
Getty Images/iStockphoto
EHR Sepsis Alert Volume Raises Clinician Burden Concerns
While hospital census declined during COVID-19, EHR sepsis alert volume increased by 43 percent, highlighting clinician burden concerns.
Healthcare organizations that leverage the Epic Sepsis Model (ESM) experienced a 43 percent increase in EHR alerts during COVID-19, according to a study published in JAMA Network Open that raises clinician burden concerns.
Sepsis early warning systems aim to provide timely clinical decision support for recognizing and treating sepsis. Traditionally, these early warning systems have relied on simple clinical rules, like systemic inflammatory response syndrome criteria, to identify patients with possible sepsis. However, these systems have not been shown to reliably improve patient outcomes.
Systems like the ESM aim to improve sepsis detection through artificial intelligence algorithms. However, concerns have arisen recently regarding the potential for sepsis models to overwhelm clinicians with EHR alerts.
The University of Michigan paused ESM alerts in April 2020, about three to four weeks after its first COVID-19 hospitalization, when nurses reported that the system was over-alerting them and causing fatigue.
Researchers quantified the number of alerts generated by the ESM at 24 hospitals in the months before and during COVID-19 to evaluate the extent to which nursing reports of sepsis over-alerting were linked to alert volume.
The study revealed that in the three weeks before and after the first case of COVID-19 at health systems included in the study, the share of patients generating sepsis alerts per day more than doubled from 9 percent to 21 percent.
Additionally, while the total hospital census declined by 35 percent over the study period, the total number of EHR alerts per day increased by 43 percent.
The researchers noted that larger hospitals generally experienced an increase in the proportion of patients generating sepsis alerts, while the change in the alerting proportion across smaller hospitals was more varied.
The study authors suggested that the increase in alerting could have resulted from dataset shift, a phenomenon in which AI model performance deteriorates due to changes in the case mix. In this instance, the influx of COVID-19 data could have affected the model’s performance and lead to inaccurate alerts.
However, even accurate alerts can be disruptive in the absence of sufficient resources. For example, more than a quarter of hospitalized patients in the first wave of the COVID-19 pandemic in 2020 died or required a transfer to an intensive care unit.
“Although the increase in the proportion of patients generating sepsis alerts in this study can be explained by the cancellation of elective surgeries and a higher average acuity among the remaining hospitalized patients, the 43 percent increase in total alerts illustrates the increased alerting burden imposed by COVID-19 on a sepsis model,” the study authors wrote.
The researchers explained that their study was limited because they did not evaluate the sepsis model’s accuracy. However, they noted that even if the system alerts were accurate, many existing sepsis workflows are built around bacterial sepsis and may not be appropriate in the context of COVID-19.
Health systems using AI to support clinical care hold a responsibility to assess and disable AI-based alerts to ensure they are not resulting in clinician burden, the researchers said.
“Given the susceptibility of AI-based systems to changes in alerting patterns, clinical AI governance within health systems may play a role in monitoring and supporting deployed AI systems,” they concluded.