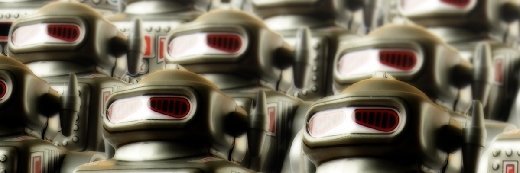
Graeme Dawes - Fotolia
Philip Tully: AI cyberattacks, AI arms race are coming
Malicious actors are working on AI cyberattacks and other ways to augment threat activity with AI. Philip Tully discusses how that can work and if enterprise security can keep pace.
Artificial intelligence and machine learning are hot topics in cybersecurity, but any technology that's available to infosec professionals is also available to threat actors.
How AI cyberattacks can work and how malicious actors may use AI to automate threat activity is still somewhat theoretical, but Philip Tully, principal data scientist at ZeroFox, discussed how it could work and whether the AI arms race can fall in favor of infosec professionals or threat actors. He explained how threat actors can use AI to improve reconnaissance for certain types of cyberattacks and also detailed his own experiences with recon tools and data collection.
In part one of the discussion with SearchSecurity at RSA Conference 2018, Tully explained the challenges for enterprise adoption of AI models. Here, he details how threat actors could stage AI cyberattacks with relative ease.
How are threat actors using machine learning and AI to bolster their own activity or launch AI cyberattacks?
Philip Tully: I haven't observed any data that provided strong evidence that an AI based-attack has ever been waged in the wild by a bad actor. On top of that, I'm not sure that one could ever prove that ever occurred. You can prove that the attack can be attributed to an automated system because of the speed at which it occurs, but attribution is so hard that there's no way [to prove it's AI], unless you had access to the model that was producing the data and you could query it and generate an output from it. I think it's going to be really hard to ever prove, 'OK. This attacker was an AI-based system.'
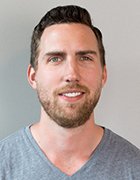
I definitely foresee this happening. The bars are being lowered for entrance into this field, data science; it's becoming more popular. Back in the day, you couldn't train accurate models on your local computer. Nowadays, you can spin up a box in the cloud -- in Amazon or Google -- and you can train huge cumbersome and complex models. You can have access to a GPU cluster that paralyzes all these different neural nets that you have. And they're actually designing software now, both Google and Amazon SageMaker and AutoML, whose sole intended purpose is to make machine learning algorithms as easily accessible as possible. They're basically trying to take a guy like me out of the equation. They're trying to take the data scientist out of it and take away the expertise that's needed.
On top of that, there's just so much more access to educational resources and it's becoming so much more part of the zeitgeist now. I think in five years, you'll have machine learning being taught in high schools, if not already. It's going to become a second nature because it's going to become so ... ubiquitous. So there are trends like that that are going to lower the bar for an attacker. And as soon as there's financial incentive and as soon as they can find a way to bypass all that previous knowledge that you need to have and use these tools to make money or to achieve their end goal, for sure they're going to engage in this type of activity.
How far away is that or has it happened yet? I would disagree that it's happened yet. I don't think it's super far away. I think it's still abstract at this point.
Another advantage the attacker has is that you could see the whole vendor landscape is extremely segmented. Everyone is competing with each other. And a lot of times the researchers in an organization can't share data they've seen with other researchers from other organizations.
These are easy to demonstrate because they are published benchmarks, there is published data, [and] there are things to compare with. And due to several reasons, cybersecurity as a field doesn't have that luxury because if I had a customer and they suffer an attack or they get targeted, I'm not at liberty to share that data outside my organization, let alone outside my team. It's extremely sensitive data that the customer is going to want basically privileged access to. They might want to do forensics and follow up on that or have us do it, but there's no way I'm going to be able to take that incident and give it to a competitor or someone else in field.
What you end up with is hundreds and thousands of different, unique solutions. And the attacker can kind of take advantage of that segmented and fragmented landscape in cybersecurity where there's no single model that's like the 'God model.' There is just a ton of advertised incredible models. In reality, who knows how good they are because no one is sharing anything with each other. So there are systematic advantages that the attacker has. That being said, I think we're still some time off from seeing this as an actual profitable and effective technique.
Would a threat actor be able to train its own AI model in a similar way to a security company and use that to launch AI cyberattacks?
Tully: I would argue it's easier for them do this. We've had a simulation that was presented a few years ago at a conference that showed how easy it was to target people over Twitter and using their open source timeline data to microtarget them with a tweet and an '@' mention followed by a machine generated tweet and a short link to kind of obfuscate the eventual redirect path and to scale that generation of a targeted tweet up to an arbitrary number of users using machine learning.
The idea is, 'OK, I can use data and data-driven techniques to build up a defense. Is there any way I can leverage data-driven techniques that often as well?' The answer is yes.
It was a tool called SNAP_R [Social Network Automated Phishing with Reconnaissance] ... a mouthful. Basically, we took a bunch of tweets that we didn't have to label as benign or malicious. All we wanted the model to do is to understand what it meant to be a tweet. We actually extracted data from verified users at random because verified users are more likely to not post absolute nonsense like bot-generated crap.
And so this model now, after you train it on this data, can spit a tweet out and generate a tweet, or some text that looks like a tweet, based on this data it's already seen. And then with a neural network, you're able to see the model to give it a starting point. And if I wanted to go up and target you with this model, I could read dynamically from your timeline and know you're posting about San Francisco or cybersecurity or journalism or whatever it may be and seed the model with that and then point that model at you.
The idea is that you're more likely to click at a link that I serve up to you, as it's based on and catered to your interests, than a random question out of the blue from a random user. It combines the shotgun approach of a Nigerian Prince scam -- which is like the same email to everybody but you distribute it like millions of times and it only works with a sliver of people, but that's enough if you're profitable.
This is an automated spear phishing AI cyberattack.
Tully: Exactly. It combines the scalability of that attack with the reconnaissance and the manual labor it takes to do upfront research on a target that might be a high-value target, a CEO or whatever, like a whaling attack or a spear phishing attack.
We show that you can use AI to do this in an offensive way. You combine the accuracy of that spear phishing attack with the scalability of a normal phishing attack and then get up to, like, 30% to 35% click-through rates from people just by microtargeting them based on their open source data.
We weren't serving out malicious links to the public; we were serving out benign links, but we were measuring click-through. The purpose was to raise awareness and show how easy it would be to generate automated social engineering text based on someone's timeline.
Do you expect AI cyberattacks like this to be more prevalent on social media?
Tully: I think especially in terms of social media, because that's the domain that we operate in, people tend to put their trust in the social networks like Facebook, Twitter, LinkedIn and Instagram because they see their friends on it and they associate the platform with safety and they associate their friends with safety.
We want to get likes. We're incentivized; dopamine is kicking at our brain, every retweet that we get is like a rush. This kind of behavior is contrary to common, operational security. You're not going to get in trouble if you post a selfie at the Golden Gate Bridge, but if you started talking about your address or, in an extreme sense, your social security or credit card numbers [then you're in trouble]. Not a lot of people are doing this, but you'd be surprised the number of people who take a selfie with their credit card on the photo.
I would encourage everyone to be just as private as possible, especially when it comes to their personal data when it comes to social media. I recently did an exercise where I went back through my direct message history and I've been on Facebook for over a decade. If you look at the private conversations you're having and if you ever had your account taken over, could that be a leveraged against you? Could that personal data, personal conversations, be used by someone blackmail you?
It's happening a lot to famous people because they're getting a lot of attention and there's monetary reasons to target them because if I blackmail a famous person maybe they'll give me more money than an average person. With this explosion of data maybe the hackers and the attackers are going to catch on and realize that targeting everyday users takes a little bit more work but could be just as profitable if you scale it up and if you're able to continue attacking and working off their insecurities in a sense.
How difficult was it to develop the SNAP_R AI cyberattack?
Tully: The point here is that I didn't need to have an understanding of what was benign or what was malicious; I just had to know what that user cared about. The generative model didn't care about distinguishing bad from good. But if I wanted to make a defender against this model, I would have to ground all those malicious tweets that they know and then I would have to have a whole population of benign tweets, label each one, so the model can tell the difference between them, and then sort that out.
So, you see there's a bottleneck here. There's a bottleneck involved in labeling each of these pieces of the data. Nowadays with these models that are coming up, they're super complex. These deep learning models involved many layers, many units per layer, so there are millions of free parameters. And with that type of complex model you need commensurately more labeled data.
You can't just get by with 100 good, 100 bad. You need, now, on a scale of 100,000 good, 100,000 bad or millions of each. The models that are being talked about in this conference I'm sure are trained on that order of magnitude if they're in production, and the customers are seeing value out of that.
These are not trivial to generate. And not only the model itself, but labeling the data. And that is actually what takes the most time out of my life as a data scientist -- wrangling the data because data generally is unstructured. I have to structure it first and put it into place and then read it and label it, associate each piece of data with yes or no or benign or malicious.
This bottleneck is another thing that an attacker can exploit. And they have the advantage over the long term. You just extrapolate that out in the future you see that the odds start to get stacked in the attacker's favor.
There's not a huge amount of cost associated with a failed attempt. It's just always stacked in the attacker's favor. They worry about the details and the defender worries about the coverage.
So, red teaming is fun, it's sexy.