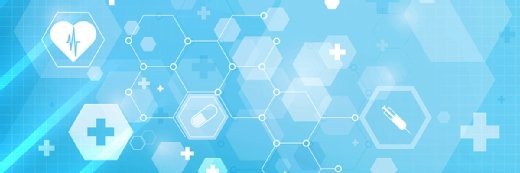
Rifqyhsn Design/istock via Getty
Predicting COVID-19 Outcomes with Artificial Intelligence, EHR Data
A simple artificial intelligence model analyzes EHR data to accurately predict which patients will have good outcomes from COVID-19.
Since the sudden onset of the COVID-19 pandemic, researchers and provider organizations have been working to develop accurate, timely artificial intelligence and data analytics tools that can help clinicians make more informed decisions.
For more coronavirus updates, visit our resource page, updated twice daily by Xtelligent Healthcare Media.
As the healthcare crisis has worn on and the industry has discovered more about the virus, developers have had think carefully about what information will be most relevant and helpful for clinical care delivery. With the operational needs of physicians rapidly changing over the course of the last several months, this has proven to be a challenging task.
“Telling a provider that someone might need an ICU bed isn’t useful, because ICU beds are already limited to patients in immediate need of higher levels of care. Predicting future needs wouldn’t change clinical management,” Yindalon Aphinyanaphongs, MD, PhD, assistant professor in the Department of Medicine at NYU Langone Health, told HealthITAnalytics.
“We had to figure out what would be useful for clinicians, and we finally settled down on favorable outcomes with COVID-19. We wanted to build something that would give providers a little bit of confidence that their patients will be okay.”
Aphinyanaphongs and his team used a combination of artificial intelligence and EHR data to better determine which patients who have tested positive for COVID-19 may be sent home safely.
The tool analyzed thousands of patient cases in New York, using each person’s vital signs, lab values, and oxygen requirements to estimate if they would have good or bad outcomes in the next four days. The model can identify hospitalized patients likely to have good outcomes with 90 percent accuracy.
The model was created to be able to seamless integrate with partner organizations’ existing infrastructure, Aphinyanaphongs said.
“We purposely built the model so that it could be easily deployed to every other Epic installation. So rather than using all features and variables that are available to us, we took the opposite approach and considered what would be the minimum amount of data needed to make a prediction. We also had to think about how a partner organization could implement this model with minimal effort,” he said.
“That was probably the biggest barrier: Some things weren't designed for this use case yet, so we had to invent some new stuff to make it work. But the end product is extremely exciting. Not only can we help patients here, but we also have this deployable infrastructure where we can build these models and share them among organizations with very little lift.”
He also emphasized that while the model focuses on favorable outcomes, it doesn’t mean that a patient who doesn’t have a favorable outcome is at risk of an adverse event.
“The model works after you've been in the hospital and you've had the worst of COVID – that’s where the model is designed to operate. It's not designed to operate at the beginning when you come in and you're on the uptrend. The virus is replicating and it's not really designed to identify adverse events in that population,” said Aphinyanaphongs.
“We’ve been running a randomized control trial with this model since May. The paper is out there to say, we've built the model, here are some statistics, and it's deployable. The RCT is designed to prove that this model can make a difference in the outcomes that matter.”
With these AI models, the goal is to deliver the right information to the right providers at the right time.
“It's all about better decision-making. These tools act as clinical decision support – they’re tools to help physicians and providers make sense of all the data feeds that are coming at them,” Aphinyanaphongs stated.
“With this novel disease, models like this can cut through the noise and help the clinician make a mental model about how to care for these patients. That's a huge contribution here, to help patients get out of the hospital just a little bit earlier.”
Providers should also continue to be vigilant when using tools like these, Aphinyanaphongs noted.
“You have to understand your patient populations and decide whether the outputs make sense for certain individuals. You see examples where the physician has some knowledge that the machine doesn't have. That's why it's all about augmentation. It's all about bringing together the AI with the knowledge that the physician has to help them make better decisions,” he said.
“That's the right way to use this tool, is to have your thoughts about what you think is happening, and then looking for corroboration with the tool. And if you’re divergent, you can see whether you’ve missed something.”
The model built by Aphinyanaphongs and his team is one that is designed to seamless fit into clinicians’ workflows, eliminating difficult integration processes.
“It would be great for organizations to not think of AI models as these tools that take months and months and months to deploy, and then when you finally get it out there, it's too late,” Aphinyanaphongs said.
“These are tools that can be used today if you have the right foundational infrastructure. That message should be passed on, because it was expressly built to be compatible and easily deployed.”
Going forward, developers and provider organizations will need to further validate and demonstrate the potential for these models to improve care decisions.
“We should continue to leave a high bar for AI. It's not enough for us to say, this platform can provide you this prediction, so it’s going to make your care better. Through this randomized controlled trial, we'll see what the benefits are. It's kind of scary, because we've invested a lot of time and energy, so you want there to be a positive result. But it may turn out that it’s not useful. With AI in healthcare, there will be a lot of stories like that,” Aphinyanaphongs concluded.
“We are just beginning to have a suite of tools that allow us to do this in a digital fashion, which means that it is cheaper to do than trying to do full blown randomized clinical trials. But the industry should continue to think about how we can get people to think of these things as devices that need to be proven to work.”