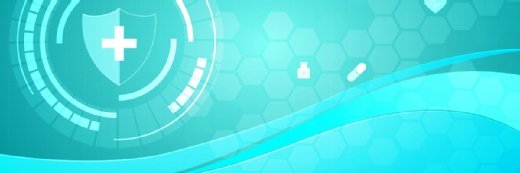
Pramote Lertnitivanit/istock via
How digital twins are reshaping clinical trials
As drug development costs soar and timelines tighten, AI digital twins help sponsors cut enrollment, speed timelines and improve trial design, while meeting regulatory standards.
With the pharmaceutical industry facing increasing pressure to optimize development costs and timelines, AI-generated digital twins could change how clinical trials are conceived and conducted. By using patient baseline characteristics to simulate individualized health trajectories, these advanced statistical models enable more efficient study designs, better decision-making and, in some cases, significant cost savings.
"An AI-generated digital twin is a comprehensive prediction of a patient’s future health outcomes," said Jon Walsh, Co-founder and Chief Scientific Officer at Unlearn, in an interview. "These predictions cover the multitude of assessments, laboratory tests and events that define a patient's health trajectory."
While the term "digital twin" is often associated with synthetic control arms, Walsh stressed that the most powerful and regulatory-friendly application lies in randomized controlled trials (RCTs). In this context, digital twins do not replace human subjects but act as prognostic covariates, enhancing trial efficiency while preserving randomization and statistical rigor.
"Digital twins make every patient more valuable," Walsh explained. "Applied correctly, this means that trials may be run with fewer participants to achieve the same quality of evidence."
Enhancing RCT efficiency and data confidence
Within RCTs, digital twins allow for prognostic covariate adjustment, which leverages predictive data as part of the statistical model to reduce variability and sharpen treatment effect estimates. This framework is consistent with FDA and European Medicines Agency (EMA) guidance and has already demonstrated success in clinical applications.
This approach enables sponsors to reduce sample sizes without compromising the integrity of the results. In a development environment where phase 3 trials often exceed $100 million in cost and require years to complete, even modest reductions in enrollment can deliver substantial time and budgetary gains.
Digital twins can also support the analysis of subgroup effects and bolster confidence in trial outcomes. Applied retrospectively, they provide a tool for evaluating the plausibility of control arm results -- an increasingly valuable function in complex or heterogeneous indications.
Supporting replication without repeating entire trials
As U.S. policymakers and agencies like the NIH begin to prioritize replication in research, possibly redirecting as much as 20% of clinical trial funding to these efforts, digital twins offer a scalable alternative to fully reenrolling patient cohorts. With the NIH investing roughly $3 billion annually in clinical trials, the ability to reuse baseline data for additional analyses could drive major resource efficiencies.
"Digital twins are one approach to enable highly efficient replication studies that can lower the resource burden compared to the original trial," Walsh clarified. "This can include supporting novel designs that replicate key results while also assessing additional clinical or biological questions of interest."
In effect, this strategy allows for scientific reproducibility without repeating entire protocols, making it especially relevant in therapeutic areas with limited eligible patient populations or high participant burden.
Early-stage acceleration through synthetic controls
In early development -- particularly phase 1b and phase 2 -- digital twins can be used as synthetic controls in open-label or single-arm studies. This design is gaining traction among sponsors seeking to make faster go/no-go decisions while minimizing patient exposure to placebos or standard-of-care comparators.
"Synthetic controls are most useful when ethical obligations mean patients should only be receiving the treatment being evaluated," Walsh stated. "They're also helpful in resource-constrained early trials where speed and cost-efficiency matter most."
By acting as a reference comparator, digital twins can enable smaller, more agile trial designs while still providing data that inform next-phase planning or investor milestone reporting.
Aligning with regulatory expectations for AI integration
As AI becomes more prevalent in clinical development, regulators have started to clarify expectations for its use. Both the FDA and EMA have published draft guidance outlining the key requirements for AI models in drug development.
While regulatory approaches may differ slightly, Walsh said most agencies align on four key principles for AI use in clinical trials:
- Understand the risks. Clearly define the potential impact of the AI application.
- Ensure traceability. Maintain a transparent, well-documented model development.
- Prioritize explainability. Make complex models interpretable and understandable.
- Demonstrate validation. Provide rigorous, fit-for-purpose evidence of effectiveness.
"The focus in specific applications has largely been on explainability and validation," Walsh pointed out. "Validation is critical to support the expected benefit of digital twins to trials."
Explainability helps build trust in the AI model's predictions and provides transparency into the biological and clinical signals driving patient outcomes. Validation efforts might include simulations using historical datasets, performance benchmarking against real trial outcomes and stress testing to confirm predictive reliability.
Sponsors using digital twins are expected to provide regulators with detailed documentation that aligns with these principles, ensuring AI tools complement -- not replace -- established statistical and clinical norms.
Validating accuracy
In a study published in Alzheimer's & Dementia earlier this year, researchers applied digital twin models retrospectively to a completed phase 2 Alzheimer’s trial and found strong alignment between predicted and observed outcomes.
"The performance of digital twins was sufficient to enable a reduction of up to 10% of the sample size in a future trial," Walsh highlighted. "For a single phase 3 trial, that translates to four months shorter enrollment and tens of millions in cost savings."
The study also confirmed that the models preserved statistical integrity and unbiased treatment effect estimates, making them useful regulatory-grade tools. In Alzheimer's and similarly high-risk therapeutic areas, these efficiencies offer a competitive advantage.
Building the infrastructure for scalable AI deployment
Because digital twins are expected to become a standard fixture in clinical research by the end of the decade, sponsors and contract research organizations that want to stay competitive must begin laying the groundwork now.
This includes the following three core investments:
- Data infrastructure. Establishing high-quality, analysis-ready datasets optimized for AI and statistical modeling, not just regulatory submission.
- Model development capabilities. Building or accessing tools to generate accurate, scalable models across diverse therapeutic areas.
- Collaborative platforms. Enabling seamless interaction between data scientists, biostatisticians and clinical teams to accelerate adoption and iteration.
"Without applications and the right collaboration models, adoption will suffer, and sponsors will lose competitive ground," Walsh warned.
As interest in digital twin applications grows, clinical teams and regulatory reviewers must understand the distinction between their uses. Walsh emphasized that, while synthetic controls are one valid use case, RCTs offer broader and arguably more robust opportunities.
"This method is much safer than synthetic controls," he said, "in that it follows regulatory guidance, produces unbiased treatment effect estimates and preserves type 1 error rate control."
As pressure mounts to do more with less, digital twins have the potential of offering pharma companies a data-driven, regulator-accepted method for unlocking the next generation of clinical trial efficiency, without sacrificing safety, validity or speed to market.
Alivia Kaylor is a scientist and the senior site editor of Pharma Life Sciences.