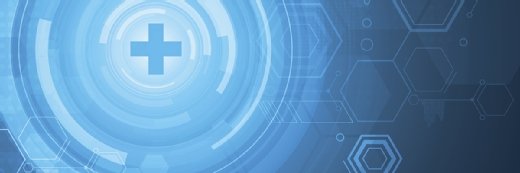
traffic_analyzer/DigitalVision V
From Pilot to Scale, Mount Sinai Leverages Autonomous Medical Coding
Mount Sinai uses autonomous medical coding technology to code about half of pathology cases, with plans to increase volume and scale soon.
An experiment to streamline medical coding is set to take off at Mount Sinai Health System.
The academic medical system nestled in the New York metropolitan area recently implemented autonomous medical coding within its pathology department to streamline coding and bring efficiencies to care delivery. Now, the technology codes about half of the system’s pathology cases, with expectations that AI-enabled coding can do more for the system.
“There’s hope we reach about 70 percent of our pathology volume in the next year,” David S. Mendelson, MD, Mount Sinai’s vice chair for radiology information technology and associate CMIO for the Mount Sinai doctor’s faculty practice, recently told RevCycleIntelligence. “And we do believe there are other opportunities. We are exploring autonomous coding for a variety of specialties.”
Autonomous medical coding is at the top of many healthcare provider wish lists this year, as organizations look to automation and other technologies, like AI, to make the administration of healthcare less complex. Medical coding is, after all, one of the most labor-intensive aspects of the healthcare revenue cycle.
Provider organizations need coding to be precise and accurate to not only paint a complete picture of patient encounters but also to generate revenue and reduce denials and other payment delays. Organizations rely on their staff’s expertise to search medical records to capture the correct codes for claims management, data analytics, and more.
Autonomous medical coding may be able to help, especially as providers face a shortage of medical coders. The technology leverages AI to assign medical codes automatically.
“There are a couple of venues where AI has rapidly been used in healthcare,” Mendelson explained. “One venue we see is workflow. We're really beginning to see, in a variety of ways, that AI can take over relatively mundane, repetitive areas in healthcare operations. And it’s not that those areas don't require expertise, but AI and machine learning can be trained to have that expertise.”
“It stood out a couple of years ago that coding was one of those areas,” Mendelson continued. “There are tasks here that are repetitive and basically work off of well-understood ways of coding things.”
This got the radiologist, by practice, asking why AI couldn’t look at the clinical documentation to help generate the codes staff would have to pick through and select. Could AI and machine learning be trained to do the same thing?
“The truth of the matter is, if they can accurately do this, they'll do it much faster than human coders will,” Mendelson said.
With the support of the CFO and prior experience with a vendor, Mount Sinai decided to pilot autonomous medical coding in pathology with CodaMetrix. The medical system went live in January 2022 with an initial coding rate between 15 and 20 percent of pathology cases. Working out some kinks, that rate quickly increased.
“Interestingly, implementation is very dependent on the way the existing lab system provides the information to the coding vendor,” Mendelson explained. “There are a lot of different lab systems out there, and they export billing data and coding reports in different ways. There's a level of work that the coding vendor has to do to configure themselves to receive the data so it's in a form that they can then code accurately. There's a lot of background work.”
This is a factor providers need to anticipate when implementing autonomous medical coding technology, Mendelson warned. In addition to the pathologist’s report, ancillary information must be considered to code and bill for the service accurately.
Mount Sinai’s coding and billing supervisors also worked closely with the vendor to identify why some cases could be coded using AI and others could not.
Implementing autonomous coding technology successfully relies on the humans who have always done the work. AI algorithms must be trained to produce accurate, complete results, and autonomous medical coding is no different. Some patient cases are complex and require human expertise to break down what happened in the language of medical codes.
“CodaMetrix sends back reports to us with the codes, or it sends back a report of what it knows it can't code, so the human coders then attack those and code them manually,” Mendelson said.
Identifying gaps in what the technology can and cannot do is part of the implementation process, but these bumps in the road have not dampened Mount Sinai’s appetite for AI in medical coding. The system moved beyond the pilot and continued their partnership to optimize pathology coding further and eventually more.
“There's no doubt in my mind this will be a staple in healthcare within the next few years,” Mendelson stated. “Let's face it, the reality is everybody's facing economic challenges in healthcare right now. You see hospitals closing, and there are a lot of mergers going on.”
Healthcare organizations are seeking ways to minimize costs while streamlining the delivery of care. Financial performance for major health systems to small physician practices has been spotty over the last year as providers grapple with economic headwinds, rising expenses, and stabilizing patient volumes. Many have turned to technology like autonomous medical coding to address staffing shortages and other inefficiencies costing their organizations money.
“You want patients to get high-quality services, and you want them to get appropriate services as rapidly as they can. So, AI is going to influence that,” Mendelson underscored. “In terms of managing the operation and the revenue coming in, AI seems to be a natural fit.”
These conditions are giving rise to a booming autonomous medical coding market, which will likely mirror what has happened with vendors in the radiology technology market, AI’s other top area of focus.
“There are a lot of startups because this kind of AI seems to be the low-hanging fruit in healthcare, at least right now,” Mendelson explained. “There are going to be some companies that rise above the others and do code accurately.”
Providers will have many choices when it comes to implementing autonomous medical coding. However, they will need to find the right fit for their organization, department, and specific workflows. Data collection and sharing obstacles, market volatility, and change management will need to be considered as providers continue down the path to AI-enabled medical coding.
One aspect Mendelson encouraged leaders to consider is their workforce. AI technology can reduce headcount for organizations, but leaders should think of what their workforce can do alongside AI-enabled workflows. Certain job functions may disappear with the rise of this technology, but other functions will emerge for humans to do.
“Realistically, in the next few years, you're going to see AI and machine learning algorithms doing autonomous coding throughout most of healthcare,” Mendelson said. “There will probably be a remaining role for human coders, for encounters that are a little more complex, a little more nuanced. Those will still require a level of expertise because they're just more complex. But I think the majority of coding is going to be taken over.”