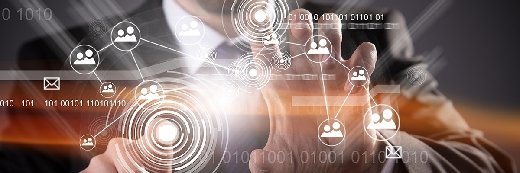
Kesu - Fotolia
Miscues in 2016 inform presidential polling data in 2020
Polls and predictive analytics models are improved in 2020 after the failure to accurately predict the outcome of the 2016 election, but surprises still may loom.
Based on presidential polling data and predictive analytics models, Hillary Clinton was expected to defeat Donald Trump in 2016.
Easily.
Much of the presidential polling data and predictive modeling, however, failed to get it right and Trump was elected president. Polling organizations didn't include enough non-college educated voters in their data, and too few polls were run in the days before the election in the states that wound up determining the outcome.
Four years later, pollsters have learned important lessons in order to include the voters they missed in 2016 who ultimately swung the election, and presidential polling data is being collected differently in 2020 than it was before the last presidential election.
But 2020, with a global pandemic hitting the United States harder than any other country and now vast swaths of the West Coast consumed by wildfires, is different also than any year. And whether the changes made to presidential election polling will lead to more accurate predictions this time around won't be known until the final polls are conducted before the election and the votes are counted.
"We learned what happened in 2016, and we've corrected for it," said Michael Cohen, founder and CEO of Cohen Research Group and an adjunct professor at Johns Hopkins University and the University of California, Washington Center. "If you see a poll now that's out of whack and seems like an outlier, generally the problem is they haven't balanced by education."
The lessons learned from 2016 will presumably fix what went wrong.
The adjustment bureau
Polling organizations absorbed what happened in 2016. They understand what they missed, and have made changes to their presidential election polls this time around. As a result, the analytics models that use polls as their primary data source will be better informed.
First and foremost, presidential polling data now largely incorporate the demographics it missed in 2016.
Using census information, polling organizations can see who self-identifies as college educated and who doesn't, and they can make sure presidential polling data more accurately reflects the entire population.
Likewise, David Paleologos, director of the Suffolk University Political Research Center in Boston, said that polling organizations are being more careful to include non-college educated voters in their data.
"Obviously, all of the polling community is sensitive to that demographic now that it has been flagged, whether it was an actual dynamic or not," he said.
In addition, those states where it was assumed Clinton would win in 2016 but lost -- Pennsylvania, Michigan and Wisconsin, in particular -- are receiving plenty of attention in the pre-election presidential election polls, and not only from local polling organizations but also those that are among the most respected nationally.
Just as Florida, Arizona and North Carolina are among the states assumed to be in play, and so are the ones that traditionally voted Democrat but swung Republican for Trump four years ago.
"The top pollsters will be polling the [blue] states again, like many of us did in 2012 but did not do in 2016," Paleologos said . "We're planning to poll most if not all of the [blue] states and then some, and maybe we'll poll other states less because we have a budget. Maybe instead of polling Florida three times we poll them twice and then include a couple of polls in Wisconsin and Pennsylvania."
What went wrong last time
Meanwhile, Paleologos noted that the national polls, which measure the popular vote, showed Hillary Clinton winning by various margins.
“And that was borne out," Paleologos said.
"But the state polls in the blue states were off," he added, referring to states that normally lean toward the Democratic Party.
Clinton, in fact, received almost 3 million more votes nationwide than Trump, but the polls were incorrect in states like Michigan, Pennsylvania and Wisconsin that went for Trump and decided the outcome in the Electoral College.
Predictive models, meanwhile, are largely based on presidential polling data. Modelers create algorithms that aggregate the various polls, while giving more weight to the ones believed to be most reliable in order to try to forecast the outcome. News organizations then use the models to inform the public, while campaigns are able to make data-driven decisions based on them.
But in 2016, because the data the models used -- the polls -- failed to pick up on the voters who swung the election, so too did the models that gave Clinton an overwhelming expectation of victory.
None of the major predictive models said a Clinton victory was 100% certain, though they said it was extremely likely. Among major predictive models, The New York Times' Upshot election forecast gave Clinton an 85% chance of victory -- Trump only won 15 election simulations out of 100 -- while CNN's Political Prediction Market gave Clinton a 91% chance of winning and the FiveThirtyEight forecast more conservatively placed the likelihood of a Clinton win at 71.4%.
And The Huffington Post's predictive model gave Clinton a 98% chance of winning.
An attempt and a miss
On Nov. 7, 2016, the day before the presidential election, a national poll by ABC News and The Washington Post showed Clinton ahead 49% to 45%. Another from Fox News had Clinton ahead 48% to 44%, and one from Monmouth University showed Clinton 50% up 44%.
And, indeed, the former Secretary of State and first lady comfortably won the popular vote.
But Clinton was also expected to win states such as Michigan, Pennsylvania and Wisconsin that narrowly went for Trump and swung the Electoral College in his favor. According to the website 270toWin.com, polls showed Clinton winning all three states she ultimately lost, with Clinton up 4% in Pennsylvania, 5% in Michigan and 7% in Wisconsin.
There wasn't a singular, clear reason presidential polling data failed to pick Trump in those states in 2016.
But according to poll experts, despite the ability to reach potential voters in myriad ways -- about 85% of polling is now done via mobile phone, according to Paleologos -- the miss can be blamed on failure to survey one particular segment of the population that leans toward Trump and a lack of polling in the states that surprisingly went to Trump on election night, Nov. 8, 2016.
"The biggest thing that people missed was the effect of education as a demographic," Cohen said.. "Education level really was a big thing, and if you do not match up education levels with what the voters are, you're going to be way off with [Trump]."
Bad data in, bad data out
And without polls accounting for that significant demographic, Cohen continued, pollsters essentially fed bad information to the predictive models that rely on polls as their primary source of data.
Paleologos similarly mentioned that many think presidential election polls failed to survey enough non-college educated voters but added that compounding the issue was the absence of quality polling in states like Michigan and Wisconsin -- the biggest surprises on Election Day.
Polling organizations such as Paleologos' Suffolk University -- CNN, Gallup, ABC News/The Washington Post and Quinnipiac University are among many others -- must be selective about where they focus their efforts. Like any organization, they're limited by the size of their staff and funding and have to carefully allocate resources. They can't simply poll every state all the time, so beyond polling nationally, they choose which states to poll based on which states figure to be most in contention.
Florida was expected to be tightly contended. So was North Carolina. So, plenty of presidential polls were conducted there by reputable polling organizations in the weeks before the election. Ten were conducted in Florida in November alone, while eight were conducted in North Carolina between Halloween and the general election.
Michigan and Wisconsin, however, were not expected to be contended, so leading polling organizations conducted far fewer polls there in the weeks before the election.
Polls needed better data
The data simply lacked quality.
"What a lot of people don't realize is that none of the top pollsters... polled those states in 2016," Paleologos said. "We were all competing in Florida and Nevada to be the most accurate -- Virginia, North Carolina, New Hampshire, Arizona. Those were the states we thought were the relevant states, not knowing what was about to happen."
Likewise, Matthew Knee, director of analytics at political consulting firm WPA Intelligence, said that while polls got the popular vote correctly, they failed to catch what was happening in the states that swung the election, leading to poor predictive models.
"People focused on national polls, and this is not a national popular-vote election," he said. "The polls were pretty darn close on the popular vote, but what they missed was Trump pulling off narrow wins in states where there was a lot less polling. And in many of these places Trump really did pull ahead right at the end."
For example, in Michigan, the Republican National Committee's model predicted a Trump victory, but that model was done after most polls had been completed, Knee added.
Uncertainty ahead
While the predictive models just under two months out from the election show Biden beating Trump, and the presidential polling data in the algorithms used by those models has been corrected for the mistakes of four years ago, there's no way of knowing whether the polls and predictive models are doing a better job of forecasting the election than they did in 2016.
Just as polling organizations didn't know until afterward they were missing an important segment of the population in their presidential polling data, and just as they didn't realize until too late they were ignoring the states that turned out to be the real battlegrounds, they won't know until after the election results whether they missed something.
"My worry is we end up in a situation where we've corrected for education, we've made our best guesses at who turns out, and we end up missing the one thing we should have known," Cohen said. "And then four years from now, we're going to put that into the model and find out we missed another thing. The biggest problem with politics is you are always fighting the last election."
In addition, 2020 is unlike any election year in history.
For nearly 250 years, an overwhelming majority of people have gone to the polls on Election Day and cast their ballots in person. A small segment of the electorate has voted absentee in the past, and in recent years some states have allowed early voting.
Because of COVID-19, however, there is expected to be a huge increase in the number of mail-in ballots, and given that 2020 may be the first time for most people voting by mail there is plenty of potential for error -- filling out a ballot incorrectly, for example, or missing the deadline for submitting mail-in ballots.
Throw in the recent financial struggles of the United States Postal Service and there's another factor to somehow be factored into the predictive models.
Now there are wildfires burning along the West Coast, and what that might mean to undecided voters remains to be seen. In addition, the security of the election remains an ongoing concern.
"The big problem is that 2020 is amazingly different than 2016," Cohen said.
In his own district in Virginia, Cohen continued, there have been more than 100% more requests for mail-in ballots than there were four years ago.
"We do not have great models for when mail-in ballots go gangbusters like they are now," he said. "If there is a shocker on Election Day, it's because we didn't see what that effect would be. Right now, we're basically changing the rules of how you vote. The unpredictability of 2020 is how we we're going to vote."
While polling organizations learned from the mistakes of 2016, they have lessons to learn from 2020 as well, and what those are won't be known until Nov. 5, or whenever the final votes are counted and a winner is declared.
"There are always lessons," Paleologos said. "We're researchers, so we're curious by nature and we're looking to find that nugget of information that might be crucial. You want to keep an open mind to the states you didn't think were in play that might be in play. We're trying to find if there's a hidden vote for either candidate."
Finding that hidden vote, and knowing which unexpected states may be in play, however, is difficult, Cohen said. The Republican Party and the Democratic Party remain stable, but what they stand for and who they represent are constantly evolving.
"The problem with elections," Cohen said, "is that each election is unique."