predictive modeling
What is predictive modeling?
Predictive modeling is a mathematical process used to predict future events or outcomes by analyzing patterns in a given set of input data. It is a crucial component of predictive analytics, a type of data analytics which uses current and historical data to forecast activity, behavior and trends.
Examples of predictive modeling include estimating the quality of a sales lead, the likelihood of spam or the probability someone will click a link or buy a product. These capabilities are often baked into various business applications, so it is worth understanding the mechanics of predictive modeling to troubleshoot and improve performance.
Although predictive modeling implies a focus on forecasting the future, it can also predict outcomes (e.g., the probability a transaction is fraudulent). In this case, the event has already happened (fraud committed). The goal here is to predict whether future analysis will find the transaction is fraudulent. Predictive modeling can also forecast future requirements or facilitate what-if analysis.
"Predictive modeling is a form of data mining that analyzes historical data with the goal of identifying trends or patterns and then using those insights to predict future outcomes," explained Donncha Carroll a partner in the revenue growth practice of Axiom Consulting Partners. "Essentially, it asks the question, 'have I seen this before' followed by, 'what typically comes after this pattern.'"
Top types of predictive models
There are many ways of classifying predictive models and in practice multiple types of models may be combined for best results. The most salient distinction is between unsupervised versus supervised models.
- Unsupervised models use traditional statistics to classify the data directly, using techniques like logistic regression, time series analysis and decision trees.
- Supervised models use newer machine learning techniques such as neural networks to identify patterns buried in data that has already been labeled.
The biggest difference between these approaches is that with supervised models more care must be taken to properly label data sets upfront.
"The application of different types of models tends to be more domain-specific than industry-specific," said Scott Buchholz, government and public services CTO and emerging technology research director at Deloitte Consulting.
In certain cases, for example, standard statistical regression analysis may provide the best predictive power. In other cases, more sophisticated models are the right approach. For example, in a hospital, classic statistical techniques may be enough to identify key constraints for scheduling, but neural networks, a type of deep learning, may be required to optimize patient assignment to doctors.
Once data scientists gather this sample data, they must select the right model. Linear regressions are among the simplest types of predictive models. Linear models take two variables that are correlated -- one independent and the other dependent -- and plot one on the x-axis and one on the y-axis. The model applies a best fit line to the resulting data points. Data scientists can use this to predict future occurrences of the dependent variable.
Some of the most popular methods include the following:
- Decision trees. Decision tree algorithms take data (mined, open source, internal) and graph it out in branches to display the possible outcomes of various decisions. Decision trees classify response variables and predict response variables based on past decisions, can be used with incomplete data sets and are easily explainable and accessible for novice data scientists.
- Time series analysis. This is a technique for the prediction of events through a sequence of time. You can predict future events by analyzing past trends and extrapolating from there.
- Logistic regression. This method is a statistical analysis method that aids in data preparation. As more data is brought in, the algorithm's ability to sort and classify it improves and therefore predictions can be made.
- Neural networks. This technique reviews large volumes of labeled data in search of correlations between variables in the data. Neural networks form the basis of many of today's examples of artificial intelligence (AI), including image recognition, smart assistants and natural language generation.
The most complex area of predictive modeling is the neural network. This type of machine learning model independently reviews large volumes of labeled data in search of correlations between variables in the data. It can detect even subtle correlations that only emerge after reviewing millions of data points. The algorithm can then make inferences about unlabeled data files that are similar in type to the data set it trained on.
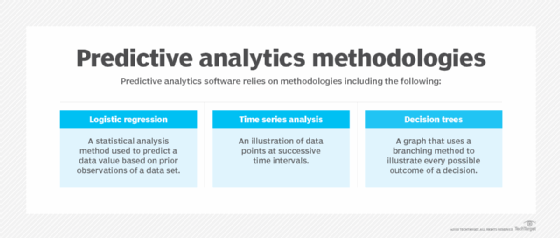
Common algorithms for predictive modeling
- Random Forest. This algorithm combines unrelated decision trees and uses classification and regression to organize and label vast amounts of data.
- Gradient boosted model. Similar to Random Forest, this algorithm uses several decision trees, but in this method, each tree corrects the flaws of the previous one and builds a more accurate picture.
- K-Means. This algorithm groups data points in a similar fashion as clustering models and is popular in devising personalized retail offers. It create personalized offers by seeking out similarities among large groups of customers.
- Prophet. A forecasting procedure, this algorithm is especially effective when dealing with capacity planning. This algorithm deals with time series data and is relatively flexible.
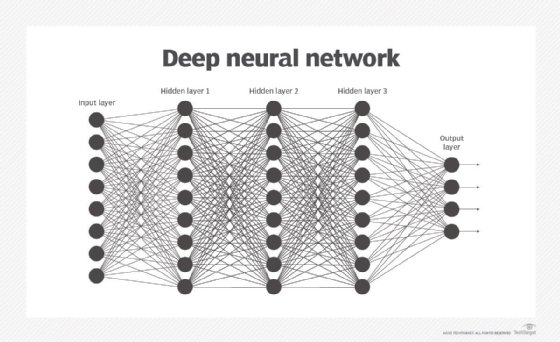
What are the uses of predictive modeling?
Predictive modeling is often associated with meteorology and weather forecasting, but predictive models have many applications in business. Today's predictive analytics techniques can discover patterns in the data to identify upcoming risks and opportunities for an organization.
"Almost anywhere a smart human is regularly making a prediction in a historically data rich environment is a good use case for predicative analytics," Buchholz said. "After all, the model has no ego and won't get bored."
One of the most common uses of predictive modeling is in online advertising and marketing. Modelers use web surfers' historical data, to determine what kinds of products users might be interested in and what they are likely to click on.
Bayesian spam filters use predictive modeling to identify the probability that a given message is spam.
In fraud detection, predictive modeling is used to identify outliers in a data set that point toward fraudulent activity. In customer relationship management, predictive modeling is used to target messaging to customers who are most likely to make a purchase.
Carroll said that predictive modeling is widely used in predictive maintenance, which has become a huge industry generating billions of dollars in revenue. One of the more notable examples can be found in the airline industry where engineers use IoT devices to remotely monitor performance of aircraft components like fuel pumps or jet engines.
These tools enable preemptive deployment of maintenance resources to increase equipment utilization and limit unexpected downtime. "These actions can meaningfully improve operational efficiency in a world that runs just in time where surprises can be very expensive," Caroll said.
Other areas where predictive models are used include the following:
- capacity planning
- change management
- disaster recovery
- engineering
- physical and digital security management
- city planning
How to build a predictive model
Building a predictive model starts with identifying historical data that's representative of the outcome you are trying to predict.
"The model can infer outcomes from historical data but cannot predict what it has never seen before," Carroll said. Therefore, the volume and breadth of information used to train the model is critical to securing an accurate prediction for the future.
The next step is to identify ways to clean, transform and combine the raw data that leads to better predictions.
Skill is required in not only finding the appropriate set of raw data but also transforming it into data features that are most appropriate for a given model. For example, calculations of time-boxed weekly averages may be more useful and lead to better algorithms than real-time levels.
It is also important to weed out data that is coincidental or not relevant to a model. At best, the additional data will slow the model down, and at worst, it will lead to less accurate models.
This is both an art and a science. The art lies in cultivating a gut feeling for the meaning of things and intuiting the underlying causes. The science lies in methodically applying algorithms to consistently achieve reliable results, and then evaluating these algorithms over time. Just because a spam filter works on day one does not mean marketers will not tune their messages, making the filter less effective.
Analyzing representative portions of the available information -- sampling -- can help speed development time on models and enable them to be deployed more quickly.
Benefits of predictive modeling
Phil Cooper, group VP of products at Clari, a RevOps software startup, said some of the top benefits of predictive modeling in business include the following:
- Prioritizing resources. Predictive modeling is used to identify sales lead conversion and send the best leads to inside sales teams; predict whether a customer service case will be escalated and triage and route it appropriately; and predict whether a customer will pay their invoice on time and optimize accounts receivable workflows.
- Improving profit margins. Predictive modeling is used to forecast inventory, create pricing strategies, predict the number of customers and configure store layouts to maximize sales.
- Optimizing marketing campaigns. Predictive modeling is used to unearth new customer insights and predict behaviors based on inputs, allowing organizations to tailor marketing strategies, retain valuable customers and take advantage of cross-sell opportunities.
- Reducing risk. Predictive analytics can detect activities that are out of the ordinary such as fraudulent transactions, corporate spying or cyber attacks to reduce reaction time and negative consequences.
The techniques used in predictive modeling are probabilistic as opposed to deterministic. This means models generate probabilities of an outcome and include some uncertainty.
"This is a fundamental and inherent difference between data modeling of historical facts versus predicting future events [based on historical data] and has implications for how this information is communicated to users," Cooper said. Understanding this difference is a critical necessity for transparency and explainability in how a prediction or recommendation was generated.
Challenges of predictive modeling
Here are some of the challenges related to predictive modeling.
Data preparation. One of the most frequently overlooked challenges of predictive modeling is acquiring the correct amount of data and sorting out the right data to use when developing algorithms. By some estimates, data scientists spend about 80% of their time on this step. Data collection is important but limited in usefulness if this data is not properly managed and cleaned.
Once the data has been sorted, organizations must be careful to avoid overfitting. Over-testing on training data can result in a model that appears very accurate but has memorized the key points in the data set rather than learned how to generalize.
Technical and cultural barriers. While predictive modeling is often considered to be primarily a mathematical problem, users must plan for the technical and organizational barriers that might prevent them from getting the data they need. Often, systems that store useful data are not connected directly to centralized data warehouses. Also, some lines of business may feel that the data they manage is their asset, and they may not share it freely with data science teams.
Choosing the right business case. Another potential obstacle for predictive modeling initiatives is making sure projects address significant business challenges. Sometimes, data scientists discover correlations that seem interesting at the time and build algorithms to investigate the correlation further. However, just because they find something that is statistically significant does not mean it presents an insight the business can use. Predictive modeling initiatives need to have a solid foundation of business relevance.
Bias. "One of the more pressing problems everyone is talking about, but few have addressed effectively, is the challenge of bias," Carroll said. Bias is naturally introduced into the system through historical data since past outcomes reflect existing bias.
Nate Nichols, distinguished principal at Narrative Science, a natural language generation tools provider, is excited about the role that new explainable machine learning methods such as LIME or SHAP could play in addressing concerns about bias and promoting trust.
"People trust models more when they have some understanding of what the models are doing, and trust is paramount for predictive analytic capabilities," Nichols said. Being able to provide explanations for the predictions, he said, is a huge positive differentiator in the increasingly crowded field of predictive analytic products.
Predictive modeling versus predictive analytics
Predictive modeling is but one aspect in the larger predictive analytics process cycle. This includes collecting, transforming, cleaning and modeling data using independent variables, and then reiterating if the model does not quite fit the problem to be addressed.
"Once data has been gathered, transformed and cleansed, then predictive modeling is performed on the data," said Terri Sage, chief technology officer at 1010data, an analytics consultancy.
Collecting data, transforming and cleaning are processes used for other types of analytic development.
"The difference with predictive analytics is the inclusion and discarding of variables during the iterative modeling process," Sage explained.
This will differ across various industries and use cases, as there will be diverse data used and different variables discovered during the modeling iterations.
For example, in healthcare, predictive models may ingest a tremendous amount of data pertaining to a patient and forecast a patient's response to certain treatments and prognosis. Data may include the patient's specific medical history, environment, social risk factors, genetics -- all which vary from person to person. The use of predictive modeling in healthcare marks a shift from treating patients based on averages to treating patients as individuals.
Similarly, with marketing analytics, predictive models might use data sets based on a consumer's salary, spending habits and demographics. Different data and modeling will be used for banking and insurance to help determine credit ratings and identify fraudulent activities.
Predictive modeling tools
Before deploying a predictive model tool, it is crucial for your organization to ask questions and sort out the following: Clarify who will be running the software, what the use case will be for these tools, what other tools will your predictive analytics be interacting with, as well as the budget.
Different tools have different data literacy requirements, are effective in different use cases, are best used with similar software and can be expensive. Once your organization has clarity on these issues, comparing tools becomes easier.
- Sisense. A business intelligence software aimed at a variety of companies that offers a range of business analytics features. This requires minimal IT background.
- Oracle Crystal Ball. A spreadsheet-based application focused on engineers, strategic planners and scientists across industries that can be used for predictive modeling, forecasting as well as simulation and optimization.
- IBM SPSS Predictive Analytics Enterprise. A business intelligence platform that supports open source integration and features descriptive and predictive analysis as well as data preparation.
- SAS Advanced Analytics. A program that offers algorithms that identify the likelihood of future outcomes and can be used for data mining, forecasting and econometrics.
The future of predictive modeling
There are three key trends that will drive the future of data modeling.
- First, data modeling capabilities are being baked into more business applications and citizen data science tools. These capabilities can provide the appropriate guardrails and templates for business users to work with predictive modeling.
- Second, the tools and frameworks for low-code predictive modeling are making it easier for data science experts to quickly cleanse data, create models and vet the results.
- Third, better tools are coming to automate many of the data engineering tasks required to push predictive models into production. Carroll predicts this will allow more organizations to shift from simply building models to deploying them in ways that deliver on their potential value.