7 top predictive analytics use cases: Enterprise examples
Across industries, companies are using predictive analytics to forecast future trends and actions. Learn about the most popular use cases for predictive analytics in 2022.
Predictive modeling helps companies optimize their internal operations, improve customer satisfaction, manage budgets, identify new markets and anticipate the impact of external events, among other uses. Moreover, as the technology becomes more accurate, easier to use and cheaper, the benefits of this type of analytics will continue to increase.
What is predictive modeling?
Predictive modeling, or predictive analytics, uses standard statistical techniques, machine learning, deep learning and other types of artificial intelligence technologies to predict future outcomes based on current and past data. It builds on descriptive analytics, which describes what happened, and is the precursor to prescriptive analytics, analyzes why something happened or will happen and what to do next.
One of the best-known and oldest examples of predictive models is weather forecasting. Predictive models are also used to create election forecasts, spread of diseases or estimate the effects of climate change.
But there are also plenty of enterprise applications of predictive analytics. Here are the top seven specific business use cases for predictive analytics today.
1. Supply chain management
Predictive analytics has long been used for operations, logistics and supply chain management.
Companies need to know how much to buy, and when and where to buy it. Buy too much, and money is tied up in inventory that can be better spent elsewhere. Buy too little and companies risk losing business, customers and revenue-generating opportunities.
The pandemic has highlighted the deficiencies companies have in their supply-chain management systems, increasing the need for statistical models that accurately forecast future events.
"Everyone is talking about supply chain," said Alexander Wurm, research analyst at Nucleus Research. "It's really exploded in predictive analytics, because you want to know in advance what's going on."
Meanwhile, the unprecedented nature of the pandemic meant that "you had to throw all the historic data out the window," he added.
That has forced companies to upgrade their predictive analytics technologies from ones that focused on historic trends, to ones that looked at real-time data and third-party information sources.
For example, companies are increasingly demanding real-time status updates on shipping containers. Fortunately, IoT devices have improved to the point that cargo ships, individual containers, pallets or even single boxes can now be tracked. At the same time, costs have decreased.
"That has changed the dynamics and ROI of these devices," Wurm said. They used to be kind of expensive, so you didn't want to use them unless it was very high importance."
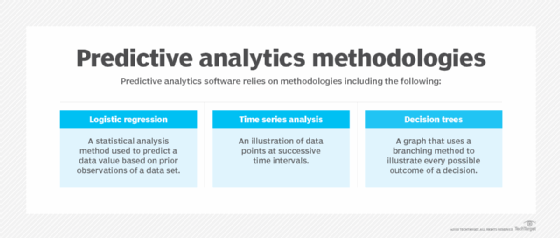
Quality control. It's not just location that can be tracked. Sensors can be used to measure temperature and impact, alerting companies, for example, to food shipments that have gone bad or goods that have been jostled. This kind of real-time data can make predictive analytics much more useful during times of rapid change.
Today, all the major supply chain management platforms offer extensive IoT-based tracking, Wurm said. Each vendor does a different level of this -- some track cargo ships, and some track individual items. But the trend is to track more, not less, as price points have come down.
The data that's collected then gets pushed out to analytics platforms. Some of the bigger vendors have analytics built in and may also have enterprise resource planning, sales and financial products that they tie into.
Smaller vendors may provide just the raw data that can then be pulled into a business intelligence platform alongside data from other sources. The business intelligence platforms will provide the integration hooks, as well as low-code or no-code tools to create predictive models, said Wurm.
The use of predictive analytics in supply chain management is expected to grow, according to a new survey by Deloitte and MHI, the logistics and supply chain industry association. The survey showed that demand forecasting is now the top use of predictive analytics, up from second place a year ago: 31% of companies are already using predictive analytics for supply chain management, compared to 28% last year. Another 48% plan to use the technology over the next five years.
2. Marketing: Buying behavior, product development, customer segmentation
Predictive analytics is everywhere when it comes to consumer products and services. Today, every major e-commerce site uses predictive analytics in one form or another, and many offline retailers, as well, use analytics to set the best possible prices for their products, or to send custom offers to potential customers.
The use of predictive models to anticipate the buying behavior of customers is also helping organizations develop new services and products for customers. In a MicroStrategy report released last year, 46% of enterprises said they've been able to create new products and revenue streams by using analytics.
Retail and healthcare companies lead in this area, according to the report, with 58% and 57% respectively stating they have used analytics for this purpose. But industries across the board are using predictive analytics to develop and tweak products and services, said Justin Richie, data science director at Nerdery. Richie noted that he recently worked with a pet adoption service that decided to use analytics to predict whether a particular animal was likely to get adopted.
Animals with low scores got extra help to increase their chances of getting placed. In addition to improving its adoption rates in 2021, the organization also found unexpected insights in the data. "They didn't realize that animals that were fostered were more likely to be adopted than animals in shelters," Richie said.
Dave Kuder, who heads up AI insights and engagement in the U.S. for Deloitte Consulting, agreed that marketing is one of the top enterprise use cases for predictive analytics right now. That's due in part to the fact that many marketing tools and systems from third-party vendors already have advanced analytics built in, he said. "AI and predictive analytics is permeating your organization and you're not even necessarily aware of it."
Predictive analytics market growing in size, importance
Projected to hit $10.5 billion this year, the market for predictive analytics is expected to nearly triple in size to $28 billion by 2026, according to Markets and Markets.
While staffing and budget issues related to the COVID-19 pandemic have put a hold on some companies' investment plans in analytics technology, for other companies, analytics has become even more critically important, helping enterprises navigate fast-changing customer behaviors and supply chain disruptions, according to the report.
The importance of predictive analytics in the enterprise was evident in a 2021 survey of nearly 2,000 knowledge workers conducted by Hanover Research for LogiAnalytics: 90% of respondents said that analytics are "very or extremely valuable" when making business decisions, and 87% said their organizations used analytics "often or very often" to make business decisions.
3. Risk management
Large financial institutions have to deal with massive volumes of information when it comes to loan applications or requests for insurance policies.
It takes time for humans to go through all this, especially in cases where industry knowledge and judgment is required, such as small business loans or business insurance policies.
With personal insurance policies, such as auto or life insurance, there are well-developed actuarial tables and risk formulas, and credit ratings for personal loans.
When it comes to enterprise customers, the situation is vastly different, Kuder explained. "There's still tremendous opportunities with small business and large corporate customers." for insurance companies.
Take, for example, a simple example of insuring two restaurants, he said. One has a single fryer, and the other has four. Intuitively, it might make sense for the restaurant with four fryers to be riskier, he said. "There's more chances to get burned."
But in fact, the single-fryer restaurant is riskier, because it's more likely to be a mom-and-pop operation without the kind of risk controls you have with a larger operation.
"Understanding [subtleties like] this … can help you quantify risk in who we should generally assign lower premiums to and who we should assign higher premiums to, so you can be more competitive in the marketplace," Kuder said. "This is a critical piece in the financial services space -- how do I get the right price and do it as quickly as possible."
A human being might know, based on experience, which restaurant is riskier. But today's firms need to make the decision almost instantaneously. Humans can't keep up -- but predictive analytics and AI can.
4. Fraud detection
Intentional fraud is a huge problem affecting all industries. The latest global crime survey from PricewaterhouseCooper reports that fraud rates have reached record highs, affecting 47% of all companies and costing a staggering $42 billion.
A recent report by LexisNexis found that retail fraud alone was up 7.1% last year -- and up 15% since the start of the pandemic -- and it's becoming more sophisticated and complex. The use of analytics in fraud detection can help, the report said, by creating a smooth and frictionless experience for retail customers. Analytics can authenticate customers when they first log in and then continue monitoring to spot suspicious behaviors as they happen.
This kind of filtering is essential today, as most companies are working with small teams of investigators who can't go through every single potential fraud case, said Deloitte's Kuder.
"Your goal is to give them the most likely claims to investigate for potential fraud," he said. "That's a perfect use case for predictive models. You can scan through hundreds of thousands of claims and refer just the top 100 that are mostly likely to be fraudulent. If you give them the right ones, the team is likely to be very productive. If you give them the wrong ones, they're not going to be productive."
5. Cybersecurity
Attackers are getting ever more adept at evading traditional signature-based defense systems. Attacks evolve quickly, and often take advantage of compromised credentials and other normally legitimate access channels.
As more employees work from home, the potential attack surface has increased dramatically. Home offices typically don't have the same kind of enterprise-grade security controls as internal corporate networks. Meanwhile, staff shortages have exacerbated the existing cybersecurity talent crunch, while the move to distributed architectures and accelerating software development cycles have greatly expanded the potential attack surface.
The numbers are staggering, as the following research studies show:
- A new global report from Zscaler on the state of enterprise attack surfaces found nearly 400,000 servers exposed to the internet, more than 200,000 exposed ports and more than 60,500 exposed instances in AWS, Azure and Google Cloud.
- Ninety-eight percent of senior executives in a recent Deloitte survey said they had a cyber incident over the past year.
- Meanwhile, the costs of a data breach have hit a 17-year high, according to the latest IBM and Ponemon research report, with the average data breach now costing a company $4.24 million.
Predictive analytics has a crucial role in protecting the enterprise. Analytics can help predict whether a new application or website is likely to be safe or malicious -- or whether a particular person's behavior is suspicious.
"With employees, you're talking about user behavior analytics," said Josh Axelrod, a cybersecurity leader at Ernst & Young. "As you begin to examine those types of behaviors, you can use analytics capabilities and machine learning to understand what is normal and what is not normal."
Analytics can also be used not just to spot suspicious movement of data, such as breach-related exfiltration of company secrets or sensitive customer information, but to spot early indicators that could indicate that an attack is imminent.
"You use analytics capabilities with machine learning and artificial intelligence to understand what is normal and what is not," Axelrod said.
Employee and customer behaviors have changed since the pandemic began, he added, which makes analytics a little less effective if the systems can't adjust quickly enough.
"It doesn't break the systems, necessarily," he added. "We've advanced enough in predictive analytics."
But it makes it even more important for companies to invest in more computing power, better algorithms and more base knowledge, he said.
6. Healthcare
The use of predictive analytics in healthcare is becoming pervasive, as documented in this TechTarget examination of the topic. From large academic hospitals and insurance companies to private physician practices, predictive analytics is being used to improve clinical care, streamline administrative tasks and optimize operations. Predictive analytics is being built into electronic health records, and IT vendors are forging partnerships with healthcare institutions to develop proprietary algorithms to improve quality and services. One long-established practice is using predictive analytics to predict which patients are at high risk of hospital readmission and adjust their post-hospitalization plans accordingly.
7. Predictive maintenance of equipment
As noted in the section on the use of predictive analytics in supply chain management, IoT data is being used to gauge the location and status of shipped goods. It is also being used to forecast equipment breakdowns.
"Modern predictive maintenance takes a proactive approach to equipment servicing using technologies such as IoT sensors and data analysis," explained Alan Earls in his recent article on predictive maintenance's role in monitoring equipment and preventing failures. Combined with machine learning, these technologies offer a "level of prediction and accuracy … that was not possible before," he said. Benefits include: less downtime, savings on machine repairs, better worker safety, higher product quality and better customer experiences.