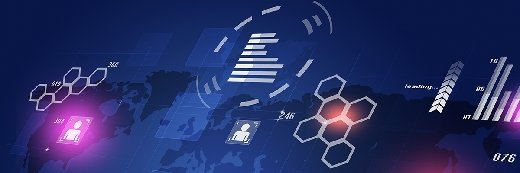
alex_aldo - Fotolia
What makes up a strong data science team structure?
Enterprises rely on a strong data science team to get the most from their data. Read on to find out what talents you'll need to employ to support your organization's data.
Each role in the data science team structure is unique. Understanding those roles and using them appropriately can make the difference between getting value out of your hefty investment in data scientists and overpaying for an underperforming team.
"Instead of saying, 'Let's just get the data scientists, and let's just build a data team,' it has to tie to business challenge, [such as] process optimization, cost savings, new product lines or what your competitors are doing," said Beena Ammanath, executive director of the Deloitte AI Institute.
Who's on a data science team?
Clearly, data scientists are a large part of the data science team structure. While many data scientists tend to have advanced degrees in math or statistics and coding skills in R or Python, they must also understand what the business wants to achieve. Their work tends to be exploratory and iterative.
Michael Yurushkin, CTO and founder of BroutonLab, a data science consultancy based in Russia, said what an organization wants to achieve should determine the type of data scientists it hires.
"If your goal is to improve content discovery, ad targeting, revenue optimization and search results, hire a team of machine learning experts," Yurushkin said. "If your objective is to test your product design using controlled experiments with minimum bias, you need a team of statisticians specialized in experimental design and causal inference."
Tyler Folkman, head of AI at Branded Entertainment Network, a product placement and licensing company based in Los Angeles, said he's a big fan of full-stack data science in which data scientists gather their own data, clean it, process it, build models, get those models to production and ensure they are providing value to their end user.
Data scientists need reliable data, however. That's where data engineers come in. They set up the data pipeline and manage the data.
"Data engineers build tools which allow data scientists to easily and effectively work full-stack," Folkman said. "I've yet to find a vendor that provides everything needed out of the box, so having data engineers to build your own platform [that] combines internal tools, open source tools and even enterprise tools is extremely valuable."
Most experts said data analysts usually work on a data analyst team or in lines of business instead of the data science team. Irrespective of where they sit, they are less technical than data scientists and data engineers, and they focus on the late stage of data science, which is analytics and sharing insights.
Folkman included data analysts and research scientists as part of the data science team structure. The analysts own the data, help make sure it's healthy and provide insights to the entire company. Research scientists advance the state of the art and invest in fundamental research.
BroutonLab's Yurushkin recommended a data strategist who serves as a link between the business and the data science team. He also recommended a data architect for companies that plan to have a large data science team.
Jesse Anderson, managing director of the Big Data Institute and author of the forthcoming book Data Teams: A Unified Management Model for Successful Data-Focused Teams, recommended three kinds of data teams: data science, data engineering and operations.
"Operations engineers have specialized their abilities for monitoring and the operational excellence around these big data systems," Anderson said.
However, when the data engineering team is missing, no one is paying attention to architecture or code quality issues, which creates years of Technical debt. When the operations team is missing, organizations may have models and code in place that don't work well in production, Anderson said.
The role of citizen data scientists and the tools they use
Citizen data scientists are power users who work in lines of business. Unlike true data scientists, they tend to lack deep statistical knowledge, do not program in R or Python and have no understanding of how machine learning works.
Augmented analytics tool vendors that say they are democratizing data science mean they're providing simple, powerful tools citizen data scientists can use to solve relatively simple problems, such as understanding why sales dipped in a region or quarter. Augmented analytics tools use AI and machine learning to simplify tasks, such as data preparation and analysis. By comparison, data scientists use expert-level tools that help solve complex problems.
"Citizen data science is just being able to access the same data that the rest of the organization is using for decisioning without waiting for support," Anderson said.
There are two ways to approach citizen data science. The first is to have a data science team build or otherwise provide self-service tools for the masses. The other is enabling lines of business to acquire their own tools. The former approach minimizes tool sprawl. It also minimizes risk by ensuring that data and data usage are governed and secure.
"If you are very well ahead in this journey, having citizen data science across your entire organization is super-critical because you want them to be able to do their own data exploration," Ammanath said. "But, if you're very early in your journey, it probably doesn't make sense to just let everybody loose on data because you need to understand the quality and context of the data."
Who do data scientists report to?
Data science teams can report to the CEO, COO, CFO, CIO, CTO, chief administrative officer (CAO), chief data officer (CDO), or other C-suite or VP title. Who the team reports to will influence what the team does. According to Anderson, chief marketing officers can be too product-focused, CFOs can be too risk-averse and a CTO or VP of engineering may not understand how data science differs from software engineering.
Ammanath said data science teams should report to a CAO or CDO because it's important to have a centralized data science function. Otherwise, the initiative gets narrow and lost.
Who the team reports to will often be determined by how it is organized. Some organizations create a centralized data science team. In other companies, lines of business hire their own data scientists. A third option for more mature organizations is to combine the two structures into a hub-and-spoke model that has a center of excellence supplemented by data scientists or data science teams with specific business domain expertise.
Do you need a CAO or CDO?
The CAO and CDO roles are often confused. Companies may use one title or the other without regard to their differences. A large company may have both.
"Chief analytics officers usually have a highly analytical background, whereas a chief data officer probably has a data engineering background, perhaps data warehouse or maybe even a DBA [database administrator] background," Anderson said.
Should you hire a CAO or CDO? The short answer is yes, if your organization is mature enough to properly support the talent and you realize you need that level of accountability. Large companies tend to create the position when the need for it becomes too obvious to deny.