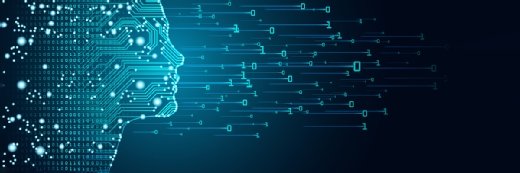
Getty Images
Machine learning tools ease cloud security, log management
Machine learning has increasingly found a home in enterprise cloud management teams, but the technology is still far from being a magic fix for complex tasks, such as multi-cloud cost optimization.
AI and machine learning continue to make their mark on nearly every aspect the IT landscape. For cloud admins, these technologies promise to automate mundane infrastructure management chores and offload maintenance functions. But while the effects of machine learning tools are significant in areas such as cloud log management and security, other use cases remain a work in progress.
Recently, the number of cloud applications, services and users has grown exponentially in the enterprise. One way to address this complexity is the addition of more IT staff, but few enterprises have the funding to match the rate of cloud expansion.
As a result, public cloud providers and third-party vendors continue to roll out AI-infused cloud management tools that can collect large volumes of data, identify patterns and automate repetitive chores.
Log management in the cloud
AI and machine learning tools are an especially good fit for log management because of their number-crunching capabilities, said Torsten Volk, managing research director at analyst firm Enterprise Management Associates.
During the day, cloud servers complete countless small steps to run various applications. When performance problems arise, IT pros often check their logs to pinpoint the application or infrastructure components that caused the problem. As enterprises expand their footprints in public cloud, the number of potential logs they need to sift through continues to grow.
Because of these mountainous data volumes, the process is time-consuming. AI and machine learning tools from vendors such as IBM, FixStream, Loom Systems, Devo and ScienceLogic automate the process of collecting and correlating records, highlighting deviations and, in some cases, even taking steps to rectify the problem. Loom, for instance, collects log information from more than 50 sources, including AWS S3 and Lamba, as well as Azure IaaS services.
Security in the cloud
On a daily basis, new devices access corporate networks, and cloud applications are deployed, upgraded, moved or taken offline. IT needs to ensure these constant states of flux don't create a point of entry for hackers to steal confidential information.
Again, AI-driven tools can help enterprises proactively address some of these challenges. Tools from vendors such as Jask, Reveelium, Splunk and Symantec sort through records and identify anomalies in cloud service access. The tools' increasing intelligence enables them to take corrective action, such as automatically removing malware after it is quarantined.
Public cloud providers, including AWS, also offer native machine learning tools for cloud security. Amazon Macie, for example, is a managed service that uses machine learning to automatically identify and guard sensitive data in the cloud. Microsoft's Azure Security Center also includes machine learning capabilities.
That's the ticket
Trouble ticketing is another area where AI and machine learning have great potential. Admins spend much of their day answering configuration, access and response time questions. Vendors like Loom, Talla and Zendesk have started to use natural language processing to offload rudimentary information input, such as entering the username and device. As a result, IT staff will have more time to add new cloud services, rather than deal with these mundane tasks.
Trouble spots
AI for cloud management, however, is still at the beginning of what's bound to be a long journey.
Every enterprise uses a unique, and often wide, set of cloud applications and services, and most AI tools currently hone in on one or two specific problems. For example, companies often struggle to accurately monitor and optimize the cost of their public cloud services. That process is exceedingly complex, especially when you consider that AWS alone has more than 92,000 pricing combinations, said Lauren E. Nelson, principal analyst at Forrester Research. Vendors ranging from AWS itself to Google, IBM, Microsoft and RightScale have developed AI-based cost monitoring products, but they don't support every cloud service from every vendor.
IT and development teams, in general, need to learn how to chain together multiple AI and machine learning models to get broader, more complete pictures of their environment, Volk said.
Additionally, IT staff often lack experience with AI, so there is a learning curve before they can put all the pieces in place.