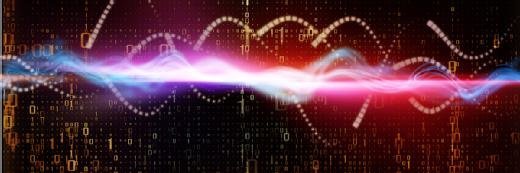
agsandrew - Fotolia
Consider the pros, cons of AI-based log analysis tools
Machine learning can help IT teams uncover new insights in system log files. But these advanced analytics capabilities, especially when achieved through on-premises tools, can be costly.
IT departments are under constant pressure to reduce outages and improve performance. Admins have traditionally used logs to pinpoint an issue's root cause, but logs have morphed into much more for the modern data center.
Logs generally contain a lot more information than a monitoring tool -- which often is limited to metrics -- to assist with IT troubleshooting. However, log files can be extensive in size -- sometimes hundreds of thousands of lines and several GBs. The average admin's desktop cannot even open a file this size, let alone find value in it. Logs, essentially, have become a form of big data.
Several vendors, such as SolarWinds Loggly and Splunk, have AI-based log analysis and aggregation tools that use machine learning to offer deep insight into data center infrastructure and applications. These tools crunch through logs from multiple sources, such as servers, networking gear and applications, to compile a more complete picture of what occurred, or might occur, in the IT environment. This capability to find the root cause of issues, and prevent new ones, is ideal for companies where poor performance or outages can quickly anger customers and drive them to look at other vendors.
Beware hidden costs
AI-based log analysis tools, however, come with both a visible and hidden cost: respectively, the cost of the software itself, and the fact that these products are very hardware-dependent. These tools require a lot of CPU power to work effectively, which can quickly drain compute resources from other workloads. IT teams can schedule jobs after hours to try to avoid this issue, but then collected data might become outdated and, therefore, less useful.
Also, machine learning for log analysis can strain IT storage resources. If admins pull log files from many sources, and store them for any amount of time, the data adds up quickly. Most likely, storing these files on the slowest disks will make the analytics less like machine learning and more like a line at the local DMV. This leads to the discussion of where to run these tools to ensure optimal performance.
Move machine learning to the cloud
While some enterprises might have the resources to run AI-based log analysis tools on premises, the majority don't. This has led to interest in cloud-based options, which have positives and negatives.
One key benefit is scalability. Compared to on-site tools, cloud-based alternatives have almost unlimited scalability to apply machine learning for log analysis. This enables real-time -- or at least near real-time -- turnaround times for data processing, which isn't always possible with on-premises tools.
The downside to this speed and efficiency is, again, cost. With any cloud-based product, enterprises pay more as they use more, and on a monthly basis. Many cloud vendors offer multiple tiers that account for data processing time frames, data volumes and number of endpoints. Evaluate where to start -- perhaps only with key applications for production environments or critical infrastructure. Admins, for example, might use an AI-based log analysis tool in the cloud for a key electronic health record system or Active Directory servers, while other services, such as those for printing, a DNS or Dynamic Host Configuration Protocol, are left out. Since there will be a monthly bill, be selective to prevent yearly costs in the thousands for log analysis.
In addition to costs, consider portability for cloud-based log services that use machine learning -- in case you decide to switch vendors. One advantage with logs is that, while they are critical to keep applications up and running, they are not part of production. This creates a little flexibility to move between services or vendors as needed.